Variance Reduction based Partial Trajectory Reuse to Accelerate Policy Gradient Optimization
CoRR(2022)
摘要
We extend the idea underlying the success of green simulation assisted policy gradient (GS-PG) to partial historical trajectory reuse for infinite-horizon Markov Decision Processes (MDP). The existing GS-PG method was designed to learn from complete episodes or process trajectories, which limits its applicability to low-data environment and online process control. In this paper, the mixture likelihood ratio (MLR) based policy gradient estimation is used to leverage the information from historical state decision transitions generated under different behavioral policies. We propose a variance reduction experience replay (VRER) approach that can intelligently select and reuse most relevant transition observations, improve the policy gradient estimation accuracy, and accelerate the learning of optimal policy. Then we create a process control strategy by incorporating VRER with the state-of-the-art step-based policy optimization approaches such as actor-critic method and proximal policy optimizations. The empirical study demonstrates that the proposed policy gradient methodology can significantly outperform the existing policy optimization approaches.
更多查看译文
AI 理解论文
溯源树
样例
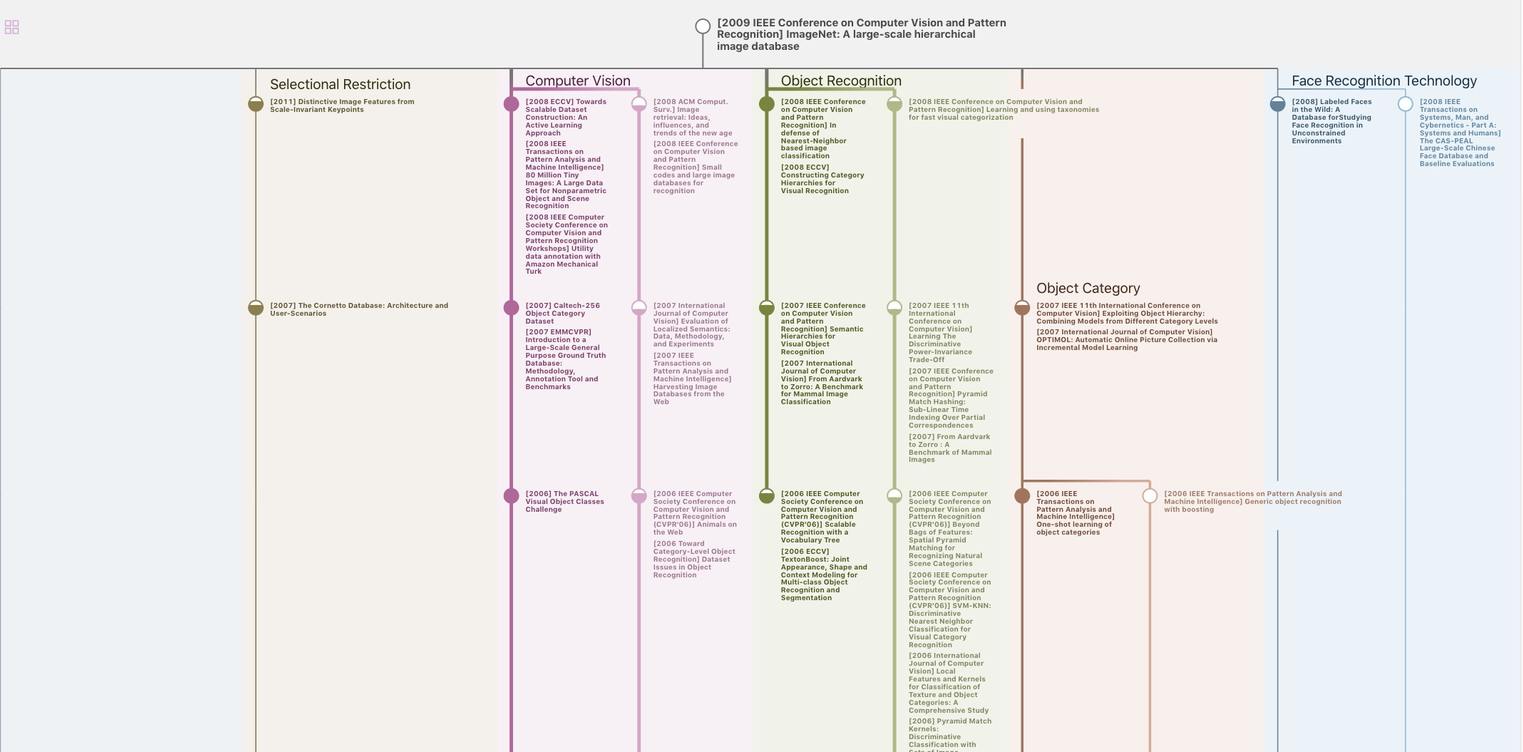
生成溯源树,研究论文发展脉络
Chat Paper
正在生成论文摘要