Towards Robust 3D Object Recognition with Dense-to-Sparse Deep Domain Adaptation
2022 IEEE INTERNATIONAL CONFERENCE ON FLEXIBLE AND PRINTABLE SENSORS AND SYSTEMS (IEEE FLEPS 2022)(2022)
摘要
Three-dimensional (3D) object recognition is crucial for intelligent autonomous agents such as autonomous vehicles and robots alike to operate effectively in unstructured environments. Most state-of-art approaches rely on relatively dense point clouds and performance drops significantly for sparse point clouds. Unsupervised domain adaption allows to minimise the discrepancy between dense and sparse point clouds with minimal unlabelled sparse point clouds, thereby saving additional sparse data collection, annotation and retraining costs. In this work, we propose a novel method for point cloud based object recognition with competitive performance with state-of-art methods on dense and sparse point clouds while being trained only with dense point clouds.
更多查看译文
关键词
Deep Domain Adaptation,Point Cloud Classification,Object Recognition,Deep Neural Networks
AI 理解论文
溯源树
样例
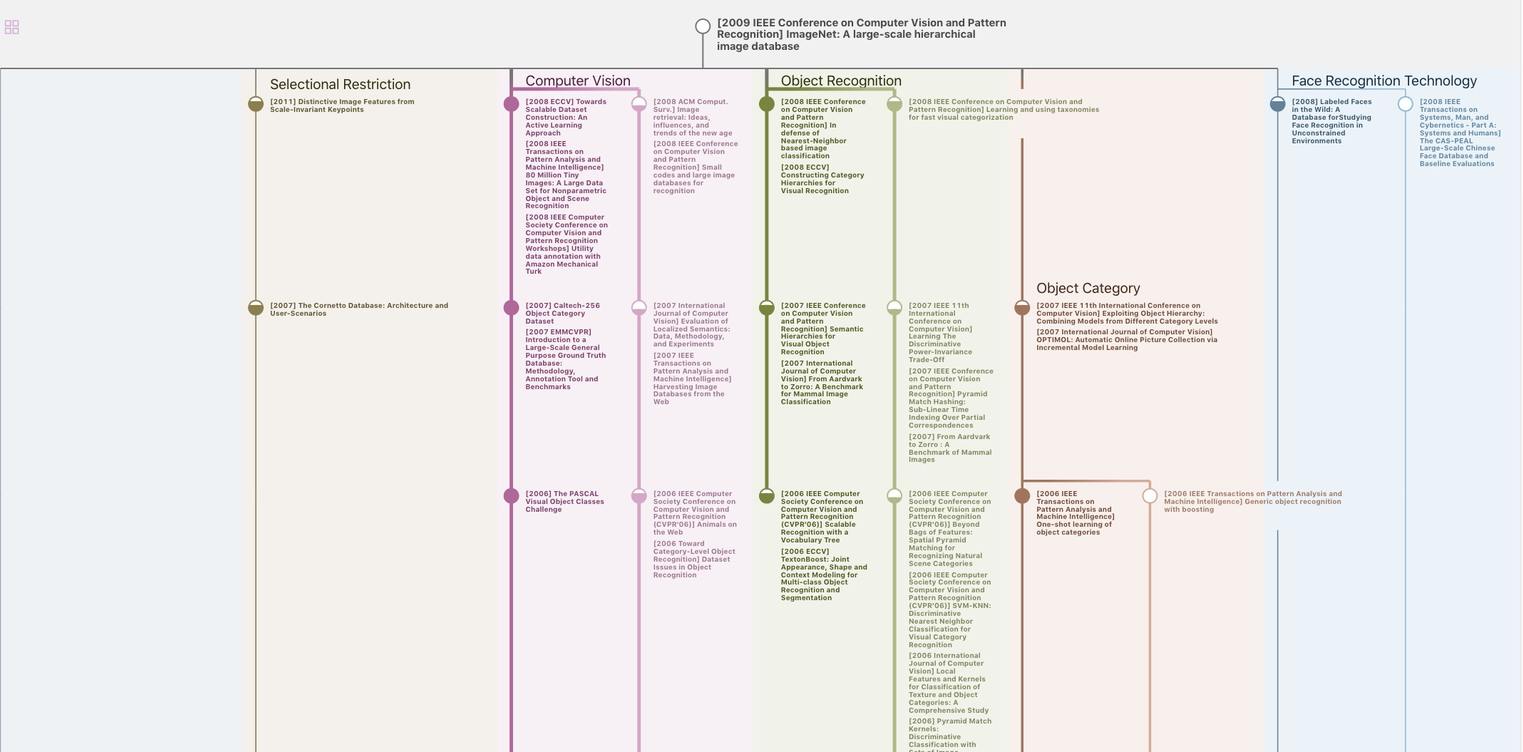
生成溯源树,研究论文发展脉络
Chat Paper
正在生成论文摘要