EASE: Entity-Aware Contrastive Learning of Sentence Embedding
North American Chapter of the Association for Computational Linguistics (NAACL)(2022)
摘要
We present EASE, a novel method for learning sentence embeddings via contrastive learning between sentences and their related entities. The advantage of using entity supervision is twofold: (1) entities have been shown to be a strong indicator of text semantics and thus should provide rich training signals for sentence embeddings; (2) entities are defined independently of languages and thus offer useful cross-lingual alignment supervision. We evaluate EASE against other unsupervised models both in monolingual and multilingual settings. We show that EASE exhibits competitive or better performance in English semantic textual similarity (STS) and short text clustering (STC) tasks and it significantly outperforms baseline methods in multilingual settings on a variety of tasks. Our source code, pre-trained models, and newly constructed multilingual STC dataset are available at https://github.com/studio-ousia/ease.
更多查看译文
关键词
sentence,learning,ease,entity-aware
AI 理解论文
溯源树
样例
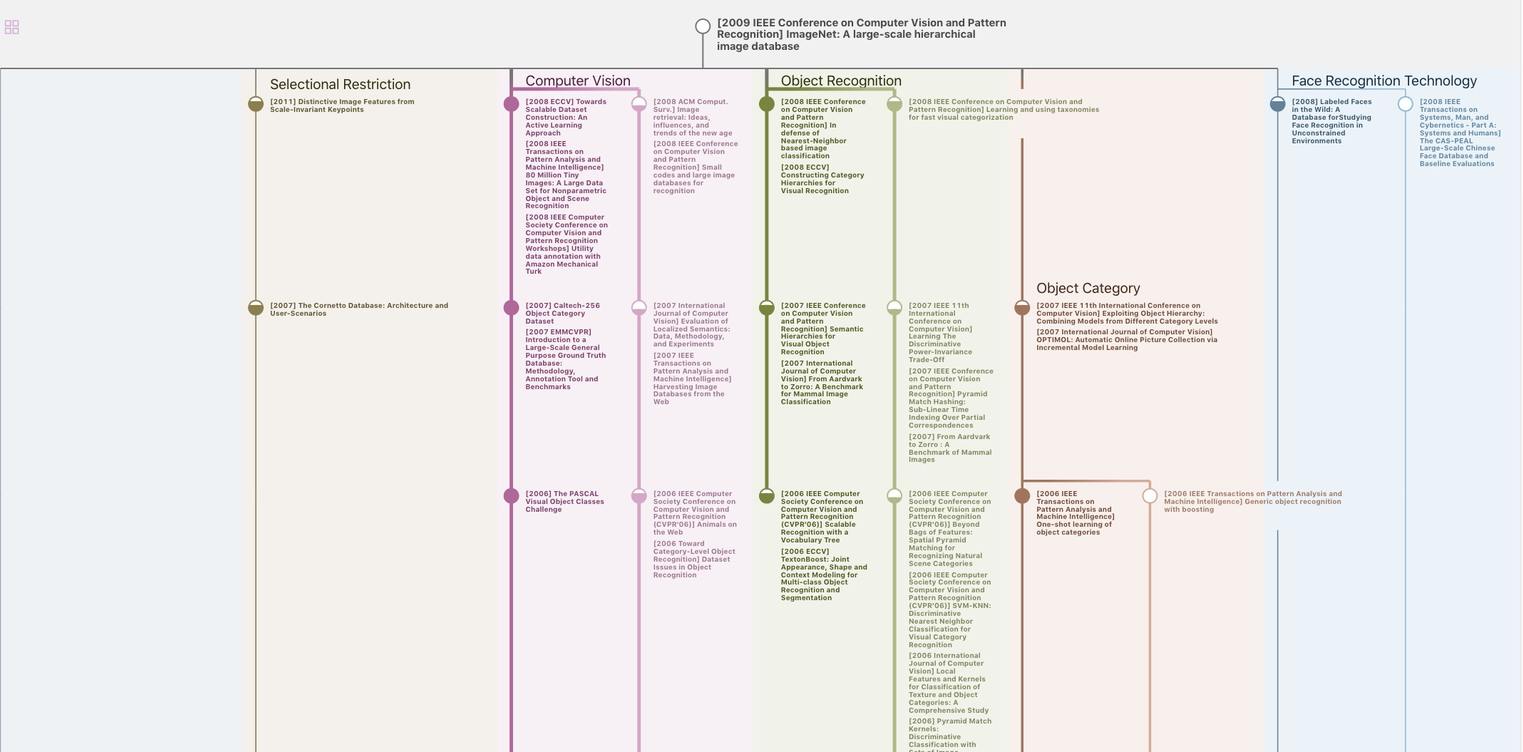
生成溯源树,研究论文发展脉络
Chat Paper
正在生成论文摘要