Machine Learning Based Propagation Loss Module for Enabling Digital Twins of Wireless Networks in ns-3
Workshop on ns-3 (WNS3)(2022)
摘要
The creation of digital twins of experimental testbeds allows the validation of novel wireless networking solutions and the evaluation of their performance in realistic conditions, without the cost, complexity and limited availability of experimental testbeds. Current trace-based simulation approaches for ns-3 enable the repetition and reproduction of the same exact conditions observed in past experiments. However, they are limited by the fact that the simulation setup must exactly match the original experimental setup, including the network topology, the mobility patterns and the number of network nodes. In this paper, we propose the Machine Learning based Propagation Loss (MLPL) module for ns-3. Based on network traces collected in an experimental testbed, the MLPL module estimates the propagation loss as the sum of a deterministic path loss and a stochastic fast-fading loss. The MLPL module is validated with unit tests. Moreover, we test the MLPL module with real network traces, and compare the results obtained with existing propagation loss models in ns-3 and real experimental results. The results obtained show that the MLPL module can accurately predict the propagation loss observed in a real environment and reproduce the experimental conditions of a given testbed, enabling the creation of digital twins of wireless network environments in ns-3.
更多查看译文
关键词
Machine learning, Trace-based simulation, Propagation loss model
AI 理解论文
溯源树
样例
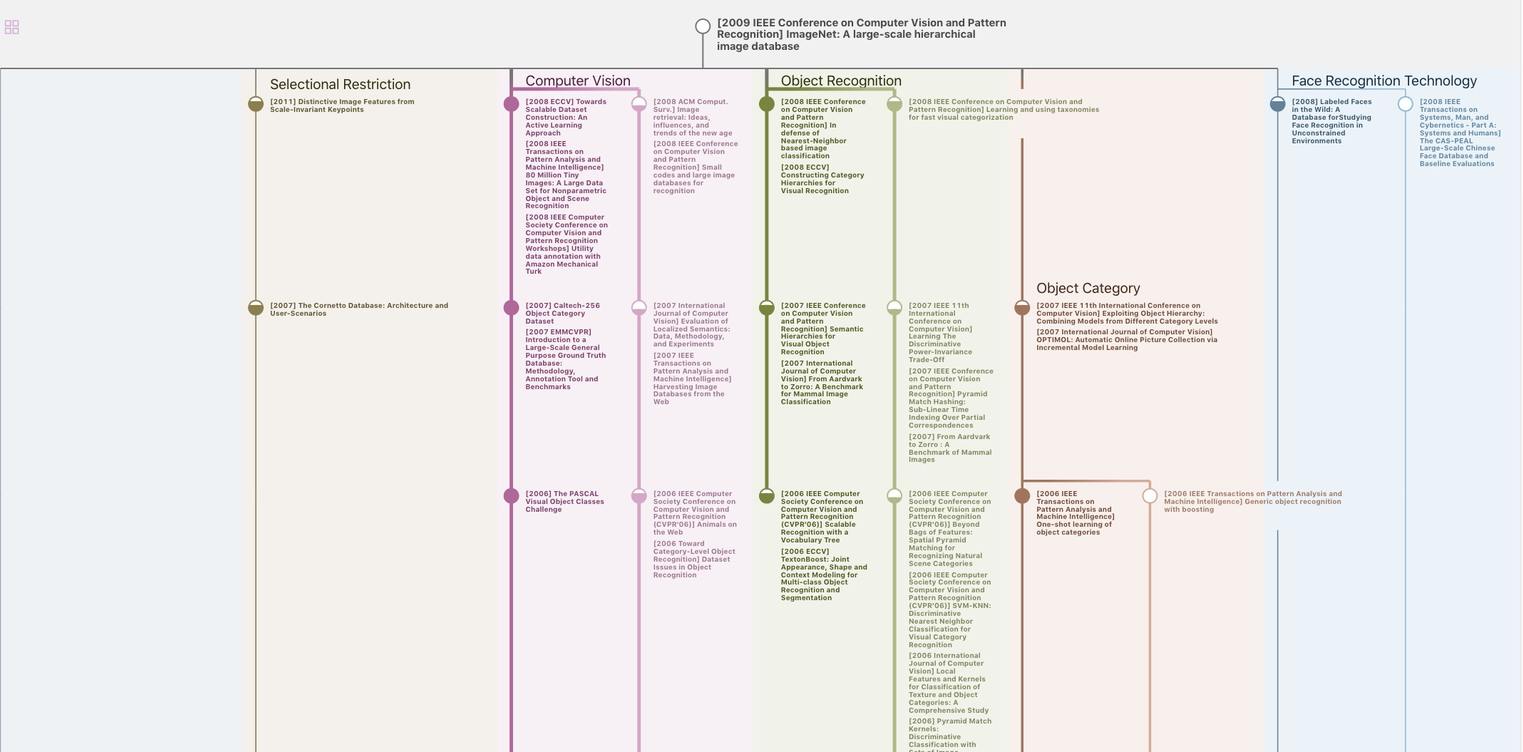
生成溯源树,研究论文发展脉络
Chat Paper
正在生成论文摘要