A noise-resilient online learning algorithm with ramp loss for ordinal regression
INTELLIGENT DATA ANALYSIS(2022)
摘要
Ordinal regression has been widely used in applications, such as credit portfolio management, recommendation systems, and ecology, where the core task is to predict the labels on ordinal scales. Due to its learning efficiency, online ordinal regression using passive aggressive (PA) algorithms has gained a much attention for solving large-scale ranking problems. However, the PA method is sensitive to noise especially in the scenario of streaming data, where the ranking of data samples may change dramatically. In this paper, we propose a noise-resilient online learning algorithm using the Ramp loss function, called PA-RAMP, to improve the performance of PA method for noisy data streams. Also, we validate the order preservation of thresholds of the proposed algorithm. Experiments on real-world data sets demonstrate that the proposed noise-resilient online ordinal regression algorithm is more robust and efficient than state-of-the-art online ordinal regression algorithms.
更多查看译文
关键词
Ordinal regression,online learning,PA-RAMP algorithm,ramp loss
AI 理解论文
溯源树
样例
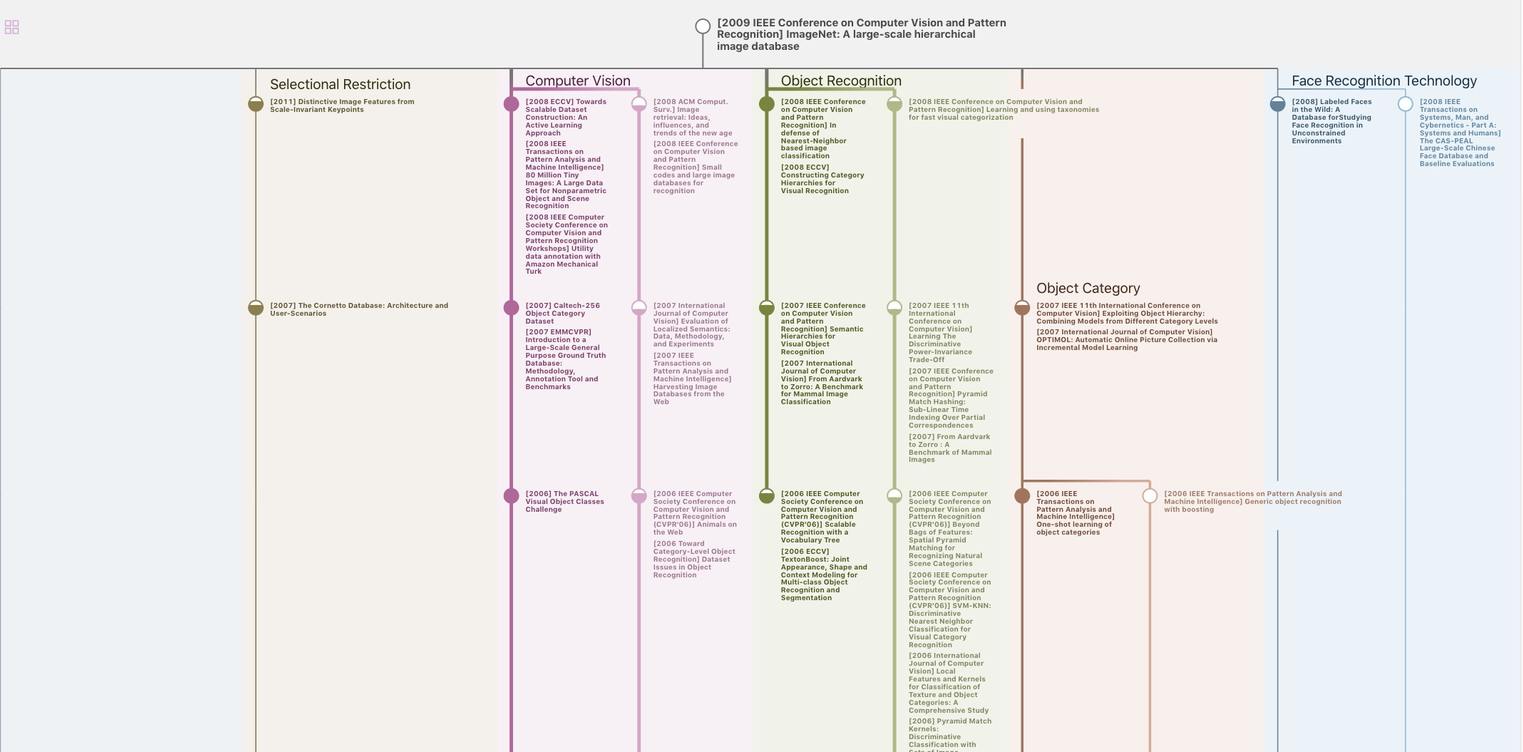
生成溯源树,研究论文发展脉络
Chat Paper
正在生成论文摘要