Multi-Agent Reinforcement Learning for Dynamic Resource Management in 6G in-X Subnetworks
IEEE Transactions on Wireless Communications(2023)
摘要
The 6G network enables a subnetwork-wide evolution, resulting in a "network of subnetworks". However, due to the dynamic mobility of wireless subnetworks, the data transmission of intra-subnetwork and inter-subnetwork will inevitably interfere with each other, which poses a great challenge to radio resource management. Moreover, most existing approaches require the instantaneous channel gain between subnetworks, which are usually difficult to be collected. To tackle these issues, in this paper we propose a novel effective intelligent radio resource management method using multi-agent deep reinforcement learning (MARL), which only needs the sum of received power, named received signal strength indicator (RSSI), on each channel instead of channel gains. However, to directly separate individual interference from RSSI is an almost impossible thing. To this end, we further propose a novel MARL architecture, named GA-Net, which integrates a hard attention layer to model the importance distribution of inter-subnetwork relationships based on RSSI and excludes the impact of unrelated subnetworks, and employs a graph attention network with a multi-head attention layer to exact the features and calculate their weights that will impact individual throughput. Experimental results prove that our proposed framework significantly outperforms both traditional and MARL-based methods in various aspects.
更多查看译文
关键词
Resource management,Interference,6G mobile communication,Wireless communication,Training,Power control,Dynamic scheduling,Graph neural network,interference mitigation,multi-agent DRL,resource management,subnetwork
AI 理解论文
溯源树
样例
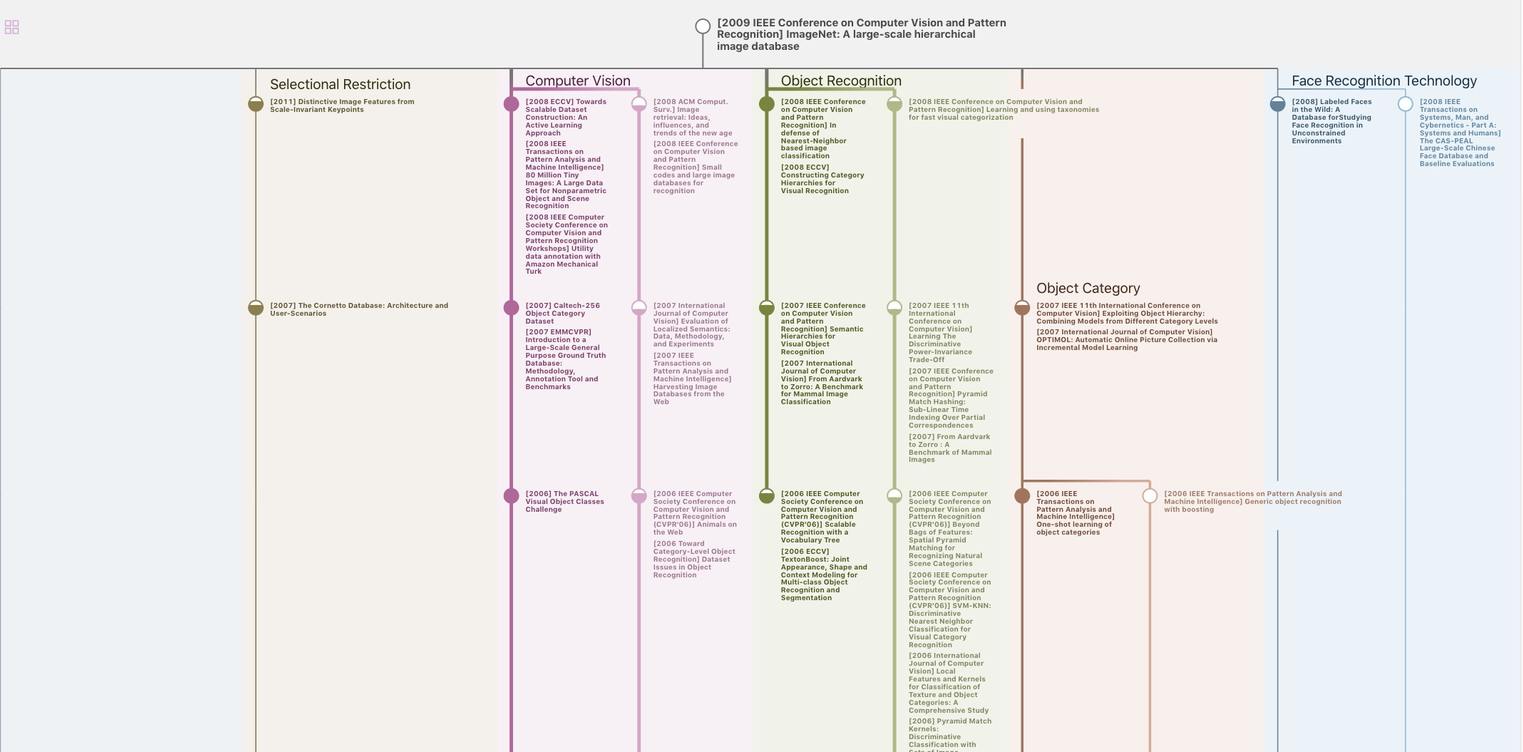
生成溯源树,研究论文发展脉络
Chat Paper
正在生成论文摘要