Data-driven tensor train gradient cross approximation for hamilton-jacobi-bellman equations
SIAM JOURNAL ON SCIENTIFIC COMPUTING(2023)
摘要
A gradient-enhanced functional tensor train cross approximation method for the resolution of the Hamilton-Jacobi-Bellman (HJB) equations associated with optimal feedback control of nonlinear dynamics is presented. The procedure uses samples of both the solution of the HJB equation and its gradient to obtain a tensor train approximation of the value function. The collection of the data for the algorithm is based on two possible techniques: Pontryagin Maximum Principle and State-Dependent Riccati Equations. Several numerical tests are presented in low and high dimension showing the effectiveness of the proposed method and its robustness with respect to inexact data evaluations, provided by the gradient information. The resulting tensor train approximation paves the way towards fast synthesis of the control signal in real-time applications.
更多查看译文
关键词
dynamic programming,optimal feedback control,Hamilton-Jacobi-Bellman equations,tensor decomposition,high-dimensional approximation
AI 理解论文
溯源树
样例
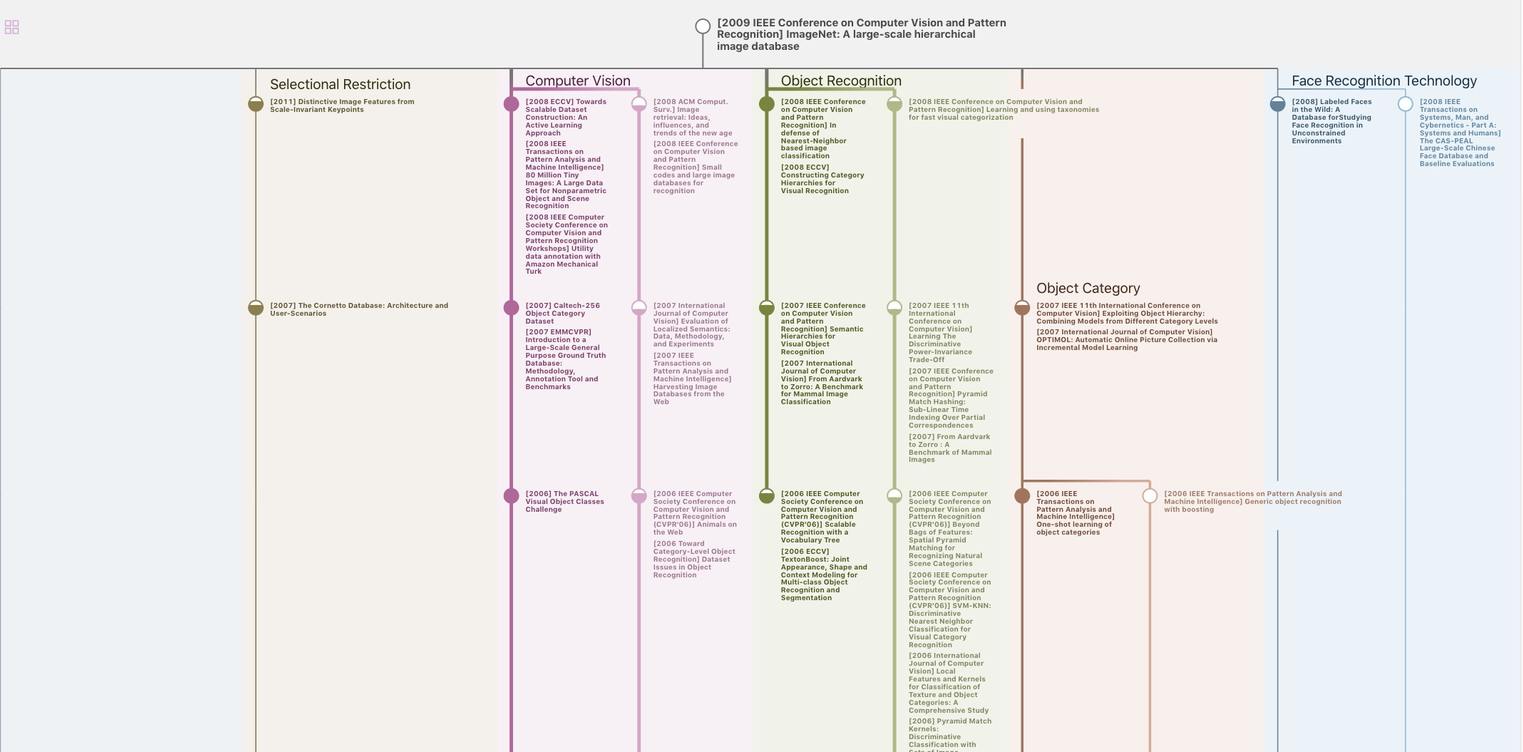
生成溯源树,研究论文发展脉络
Chat Paper
正在生成论文摘要