The AGEL Survey: Spectroscopic Confirmation of Strong Gravitational Lenses in the DES and DECaLS Fields Selected Using Convolutional Neural Networks
ASTRONOMICAL JOURNAL(2022)
摘要
We present spectroscopic confirmation of candidate strong gravitational lenses using the Keck Observatory and Very Large Telescope as part of our ASTRO 3D Galaxy Evolution with Lenses (AGEL) survey. We confirm that (1) search methods using convolutional neural networks (CNNs) with visual inspection successfully identify strong gravitational lenses and (2) the lenses are at higher redshifts relative to existing surveys due to the combination of deeper and higher-resolution imaging from DECam and spectroscopy spanning optical to near-infrared wavelengths. We measure 104 redshifts in 77 systems selected from a catalog in the DES and DECaLS imaging fields (r <= 22 mag). Combining our results with published redshifts, we present redshifts for 68 lenses and establish that CNN-based searches are highly effective for use in future imaging surveys with a success rate of at least 88% (defined as 68/77). We report 53 strong lenses with spectroscopic redshifts for both the deflector and source (z (src) > z (defl)), and 15 lenses with a spectroscopic redshift for either the deflector (z (defl) > 0.21) or source (z (src) >= 1.34). For the 68 lenses, the deflectors and sources have average redshifts and standard deviations of 0.58 +/- 0.14 and 1.92 +/- 0.59 respectively, and corresponding redshift ranges of z (defl) = 0.21-0.89 and z (src) = 0.88-3.55. The AGEL systems include 41 deflectors at z (defl) >= 0.5 that are ideal for follow-up studies to track how mass density profiles evolve with redshift. Our goal with AGEL is to spectroscopically confirm similar to 100 strong gravitational lenses that can be observed from both hemispheres throughout the year. The AGEL survey is a resource for refining automated all-sky searches and addressing a range of questions in astrophysics and cosmology.
更多查看译文
关键词
strong gravitational lenses,spectroscopic confirmation,convolutional neural networks,decals fields,des
AI 理解论文
溯源树
样例
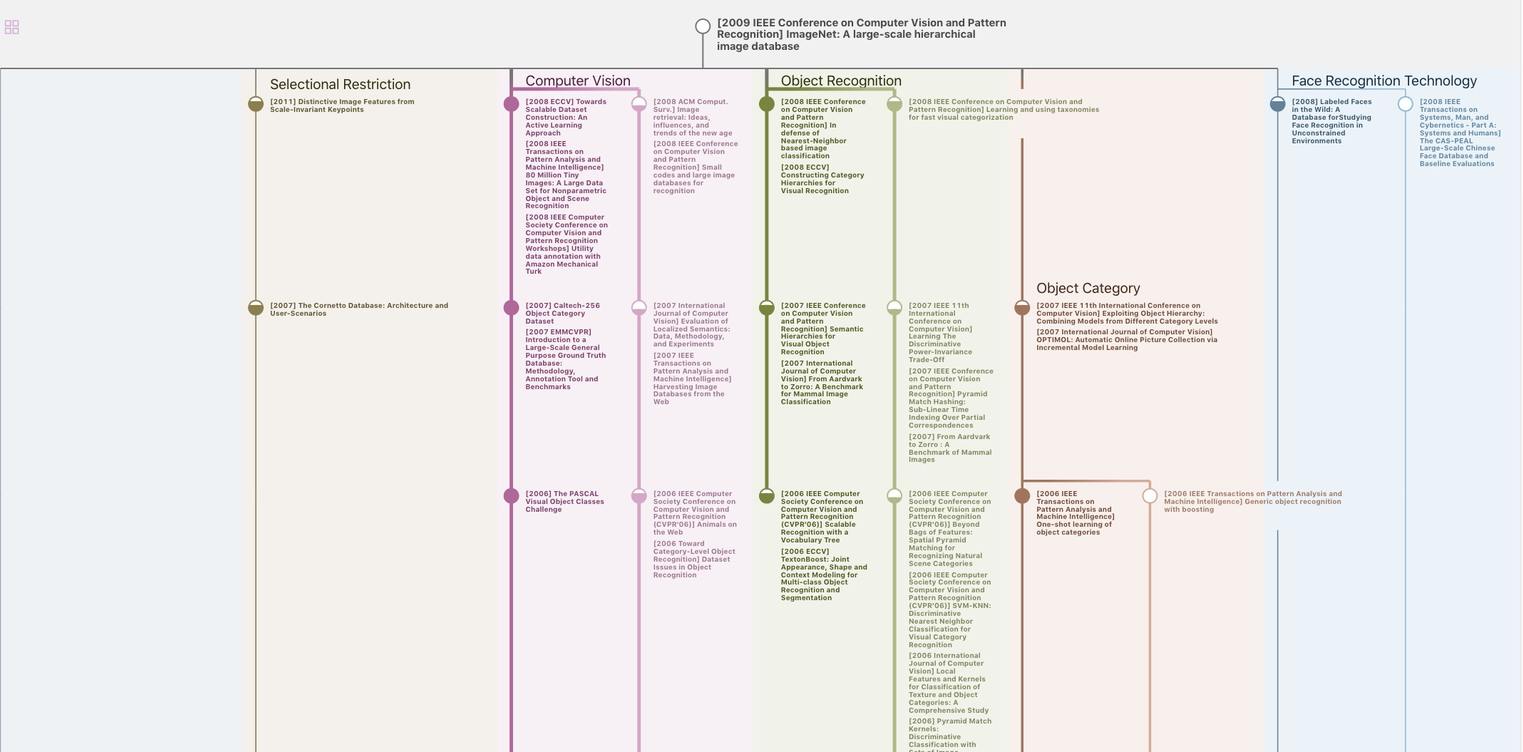
生成溯源树,研究论文发展脉络
Chat Paper
正在生成论文摘要