Leveraging baseline covariates to analyze small cluster-randomized trials with a rare binary outcome
BIOMETRICAL JOURNAL(2024)
摘要
Cluster-randomized trials (CRTs) involve randomizing entire groups of participants-called clusters-to treatment arms but are often comprised of a limited or fixed number of available clusters. While covariate adjustment can account for chance imbalances between treatment arms and increase statistical efficiency in individually randomized trials, analytical methods for individual-level covariate adjustment in small CRTs have received little attention to date. In this paper, we systematically investigate, through extensive simulations, the operating characteristics of propensity score weighting and multivariable regression as two individual-level covariate adjustment strategies for estimating the participant-average causal effect in small CRTs with a rare binary outcome and identify scenarios where each adjustment strategy has a relative efficiency advantage over the other to make practical recommendations. We also examine the finite-sample performance of the bias-corrected sandwich variance estimators associated with propensity score weighting and multivariable regression for quantifying the uncertainty in estimating the participant-average treatment effect. To illustrate the methods for individual-level covariate adjustment, we reanalyze a recent CRT testing a sedation protocol in 31 pediatric intensive care units.
更多查看译文
关键词
covariate adjustment,finite-sample corrections,generalized estimating equations,inverse probability weights,overlap weights,sandwich variance estimator
AI 理解论文
溯源树
样例
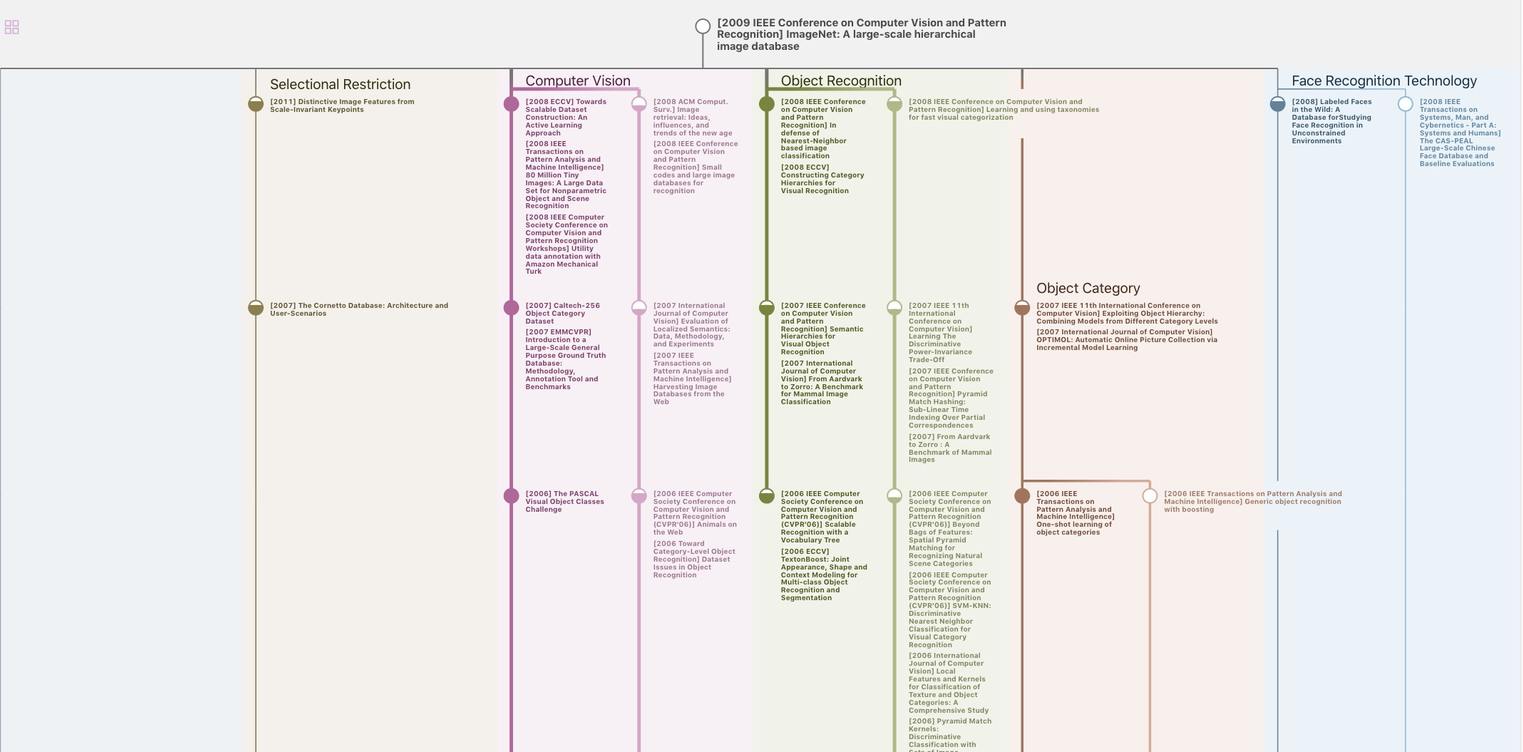
生成溯源树,研究论文发展脉络
Chat Paper
正在生成论文摘要