Attention based deep neural network for micro-fracture extraction of sequential coal rock CT images
Multimedia Tools and Applications(2022)
摘要
The extraction and analysis of fractures from sequential coal rock Computed Tomography images play an important guiding role in development of coalbed methane and permeability calculation. However, the fracture extraction from sequential coal rock CT images is usually associated with tremendous complexities due to the low-contrast and high noise. Especially, the fractures cannot be accurately segmented in the case of the close grayscale values between micro-fractures and the surrounding coal matrix. In order to tackle these challenges, this paper proposes a deep neural network (A-DNNet) based on U-Net framework for extracting micro-fractures of sequential coal rock images. Specifically, in the proposed A-DNNet, (1) a number of continuous slices are fed into CNN encoder, and these smaller size continuous feature maps are obtained by recursive operations of convolution and down-sampling; (2) ConvLSTM (convolutional LSTM) is introduced to model the inter-slices fracture correlations for the smaller size feature maps outputted by CNN encoder; (3) RFA is proposed to refine fracture features gradually by capturing discriminative features. Compared with U-Net algorithms, the extensive experiments demonstrate that our method improve the average accuracy by 1.14%, the average precision by 2.60%, the average Dice by 3.20%, the average F1 by 2.45%, respectively. The robust fractures extraction performance of our methods will inform continuing future research into the development for fracture evolution and permeability calculation.
更多查看译文
关键词
Attention, Sequential images, ConvLSTM, Coal rock fractures, U-Net
AI 理解论文
溯源树
样例
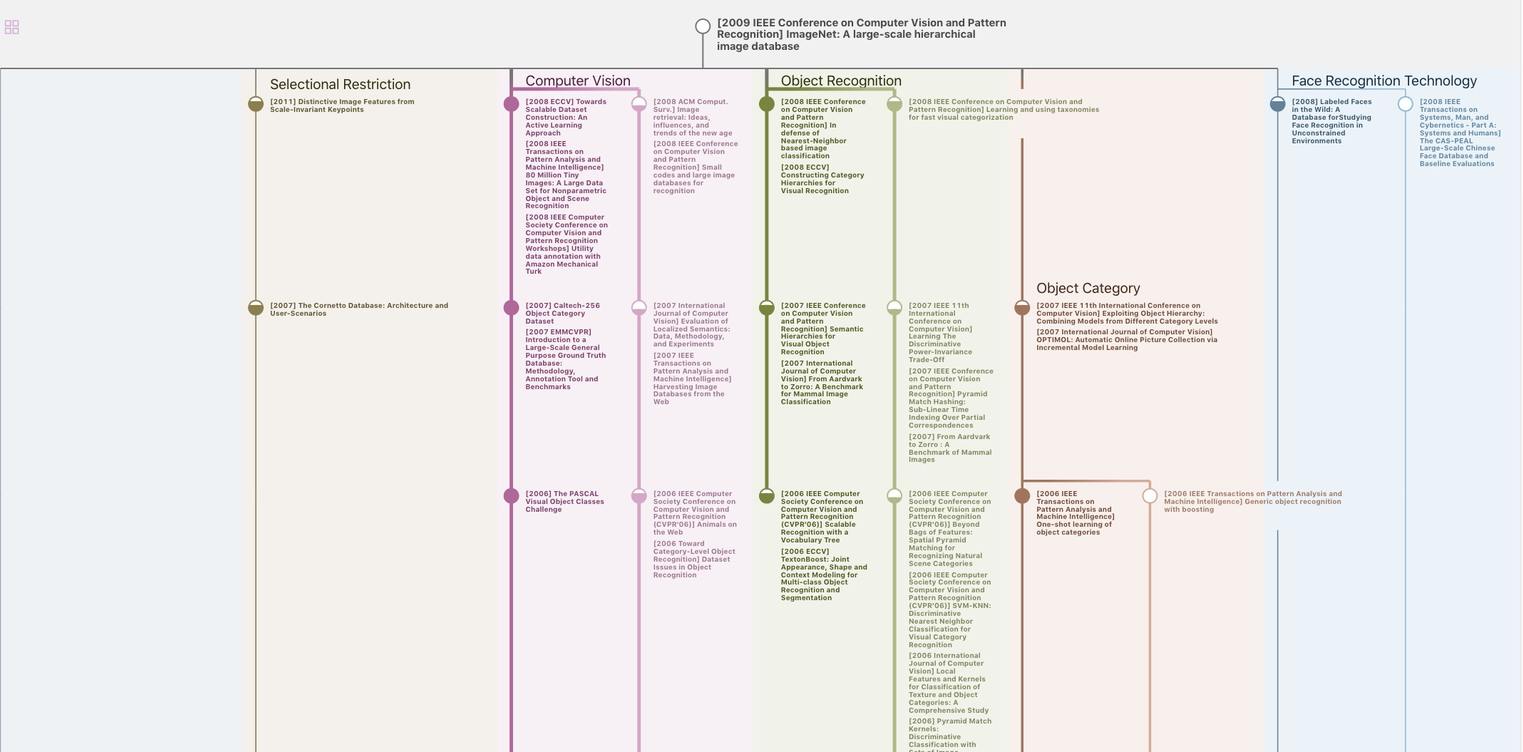
生成溯源树,研究论文发展脉络
Chat Paper
正在生成论文摘要