The role of information structures in game-theoretic multi-agent learning
Annual Reviews in Control(2022)
摘要
Multi-agent learning (MAL) studies how agents learn to behave optimally and adaptively from their experience when interacting with other agents in dynamic environments. The outcome of a MAL process is jointly determined by all agents’ decision-making. Hence, each agent needs to think strategically about others’ sequential moves, when planning future actions. The strategic interactions among agents makes MAL go beyond the direct extension of single-agent learning to multiple agents. With the strategic thinking, each agent aims to build a subjective model of others decision-making using its observations. Such modeling is directly influenced by agents’ perception during the learning process, which is called the information structure of the agent’s learning. As it determines the input to MAL processes, information structures play a significant role in the learning mechanisms of the agents. This review creates a taxonomy of MAL and establishes a unified and systematic way to understand MAL from the perspective of information structures. We define three fundamental components of MAL: the information structure (i.e., what the agent can observe), the belief generation (i.e., how the agent forms a belief about others based on the observations), as well as the policy generation (i.e., how the agent generates its policy based on its belief). In addition, this taxonomy enables the classification of a wide range of state-of-the-art algorithms into four categories based on the belief-generation mechanisms of the opponents, including stationary, conjectured, calibrated, and sophisticated opponents. We introduce Value of Information (VoI) as a metric to quantify the impact of different information structures on MAL. Finally, we discuss the strengths and limitations of algorithms from different categories and point to promising avenues of future research.
更多查看译文
关键词
Multi-agent learning,Information structures,Reinforcement learning,Belief generation,Game theory,Value of Information
AI 理解论文
溯源树
样例
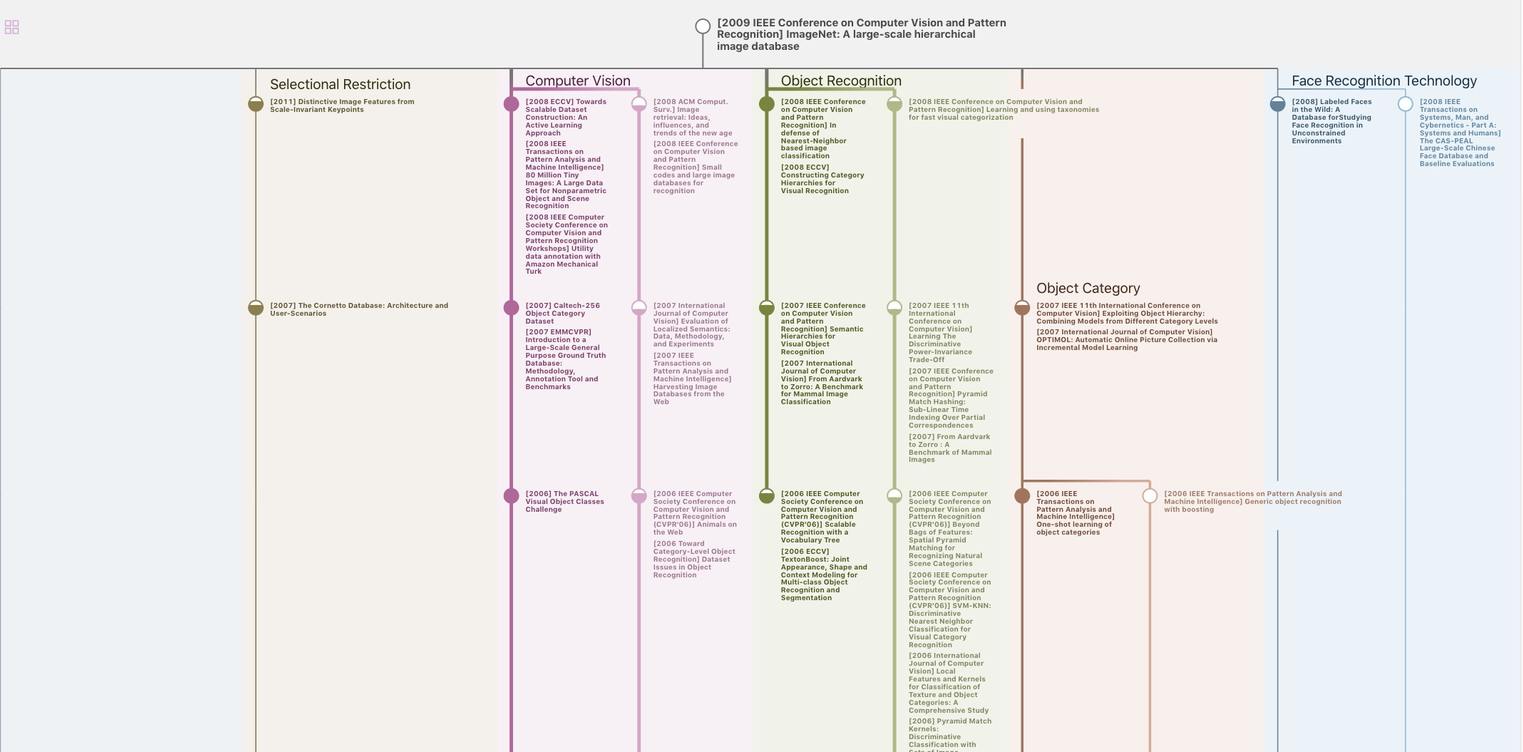
生成溯源树,研究论文发展脉络
Chat Paper
正在生成论文摘要