Performance Evaluation of Deep Neural Network Model for Coherent X-ray Imaging
AI(2022)
摘要
We present a supervised deep neural network model for phase retrieval of coherent X-ray imaging and evaluate the performance. A supervised deep-learning-based approach requires a large amount of pre-training datasets. In most proposed models, the various experimental uncertainties are not considered when the input dataset, corresponding to the diffraction image in reciprocal space, is generated. We explore the performance of the deep neural network model, which is trained with an ideal quality of dataset, when it faces real-like corrupted diffraction images. We focus on three aspects of data qualities such as a detection dynamic range, a degree of coherence and noise level. The investigation shows that the deep neural network model is robust to a limited dynamic range and partially coherent X-ray illumination in comparison to the traditional phase retrieval, although it is more sensitive to the noise than the iteration-based method. This study suggests a baseline capability of the supervised deep neural network model for coherent X-ray imaging in preparation for the deployment to the laboratory where diffraction images are acquired.
更多查看译文
关键词
coherent X-ray imaging, phase retrieval, deep neural network
AI 理解论文
溯源树
样例
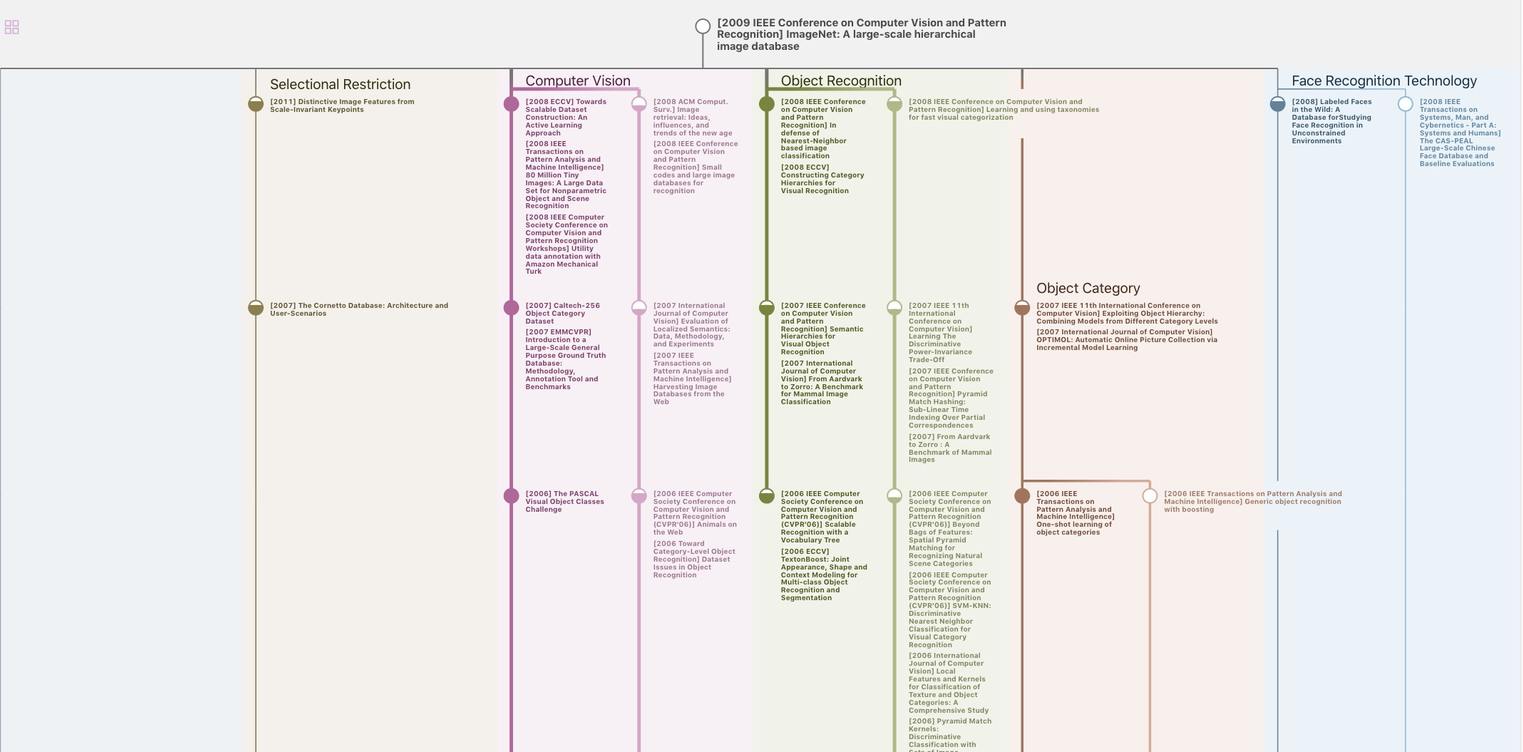
生成溯源树,研究论文发展脉络
Chat Paper
正在生成论文摘要