Multimodal time-aware attention networks for depression detection
Journal of Intelligent Information Systems(2022)
摘要
Depression is a common mental disorder, which may lead to suicide when the condition is severe. With the advancement of technology, there are billions of people who share their thoughts and feelings on social media at any time and from any location. Social media data has therefore become a valuable resource to study and detect the depression of the user. In our work, we use Instagram as the platform to study depression detection. We use hashtags to find users and label them as depressive or non-depressive according to their self-statement. Text, image, and posting time are used jointly to detect depression. Furthermore, the time interval between posts is important information when studying medical-related data. In this paper, we use time-aware LSTM to handle the irregularity of time intervals in social media data and use an attention mechanism to pay more attention to the posts that are important for detecting depression. Experiment results show that our model outperforms previous work with an F1-score of 95.6%. In addition to the good performance on Instagram, our model also outperforms state-of-the-art methods in detecting depression on Twitter with an F1-score of 90.8%. This indicates the potential of our model to be a reference for psychiatrists to assess the patient; or for users to know more about their mental health condition.
更多查看译文
关键词
Depression detection,Social media,Deep learning
AI 理解论文
溯源树
样例
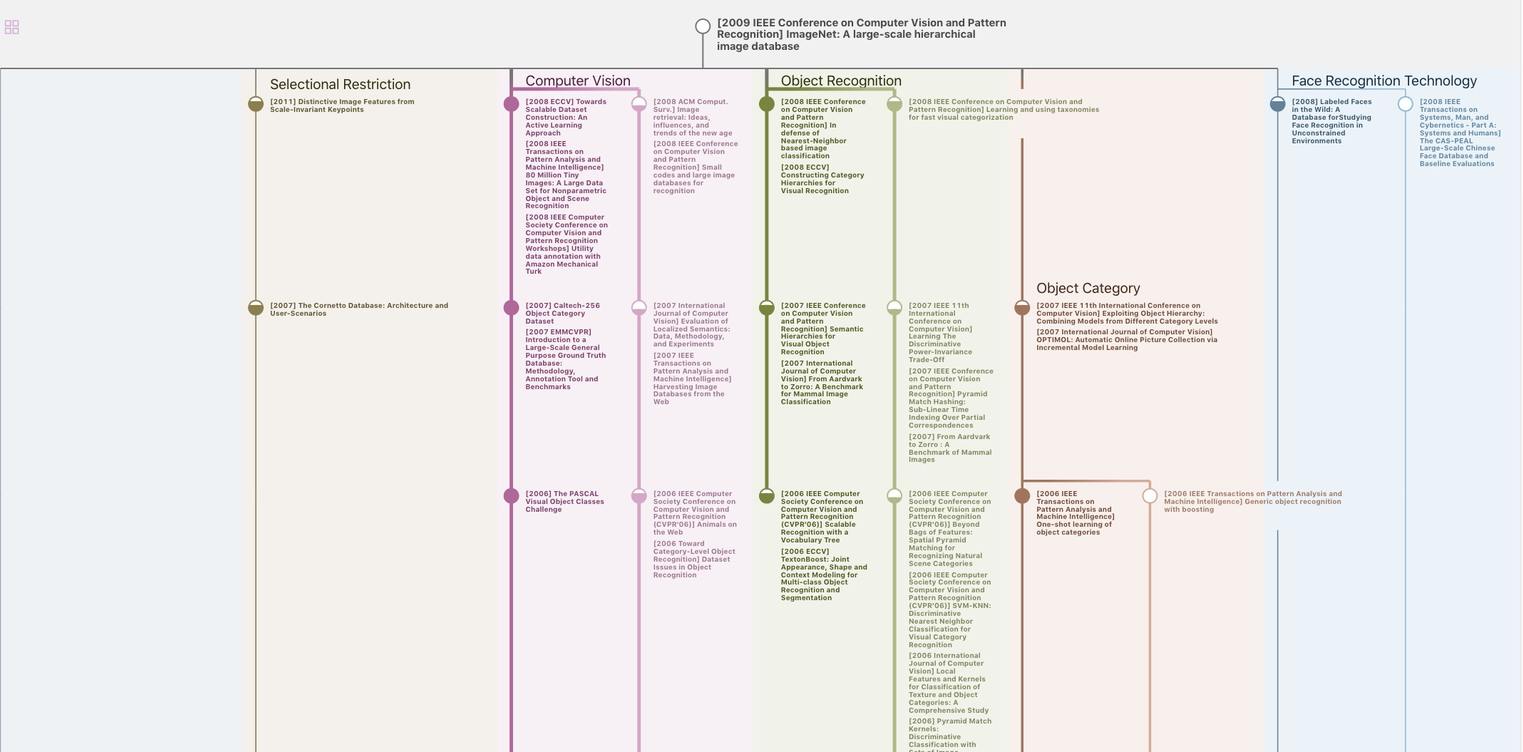
生成溯源树,研究论文发展脉络
Chat Paper
正在生成论文摘要