Performance enhancement of meta-heuristics through random mutation and simulated annealing-based selection for concurrent topology and sizing optimization of truss structures
Soft Computing(2022)
摘要
The present investigation includes the performance enhancement concept of discrete meta-heuristics (MHs) for truss design with concurrent size and topology optimization. The five basic MHs, viz. teaching–learning-based optimization (TLBO), whale optimization algorithm (WOA), dragonfly algorithm (DA), heat transfer search (HTS), and ant lion optimization (ALO) algorithm, are investigated. Often these MHs found incompetent in answering complex problems like concurrent topology and sizing optimization of truss structures due to their poor convergence rate, local optima trap, and higher computation time. Also, the balance between diversification and intensification is very significant for MHs efficiency. A mutation is a strong strategy for balancing the diversification and intensification of MHs and can assist in guiding the population towards the global optimum. Moreover, population diversity can be improved by incorporating the selection of simulated annealing that can reduce the chances of local optima tarp. Therefore, an effective search technique based on a random mutation search along with simulated annealing-based selection is developed. Here, five modified MHs, i.e. the modified DA (MDA), modified ALO (MALO), modified WOA (MWOA), modified HTS (MHTS), and modified TLBO (MTLBO) algorithms, using random mutation search phase and SA-based selection are proposed. The developed techniques are implemented on three standard test problems where dynamic and static constraints and multiple load cases are imposed. The comparative performance of the proposed algorithms and their original algorithms is carried out. An empirical evaluation was done using Friedman rank, and the respective algorithms ranks are assigned. The findings reveal that the new technique results in significant performance enhancement of the various MHs by synchronizing the diversification and intensification of search.
更多查看译文
关键词
Modified algorithm,Meta-heuristic,Mutation,Structural optimization,Discrete section,Static and dynamic constraints,Simulated annealing
AI 理解论文
溯源树
样例
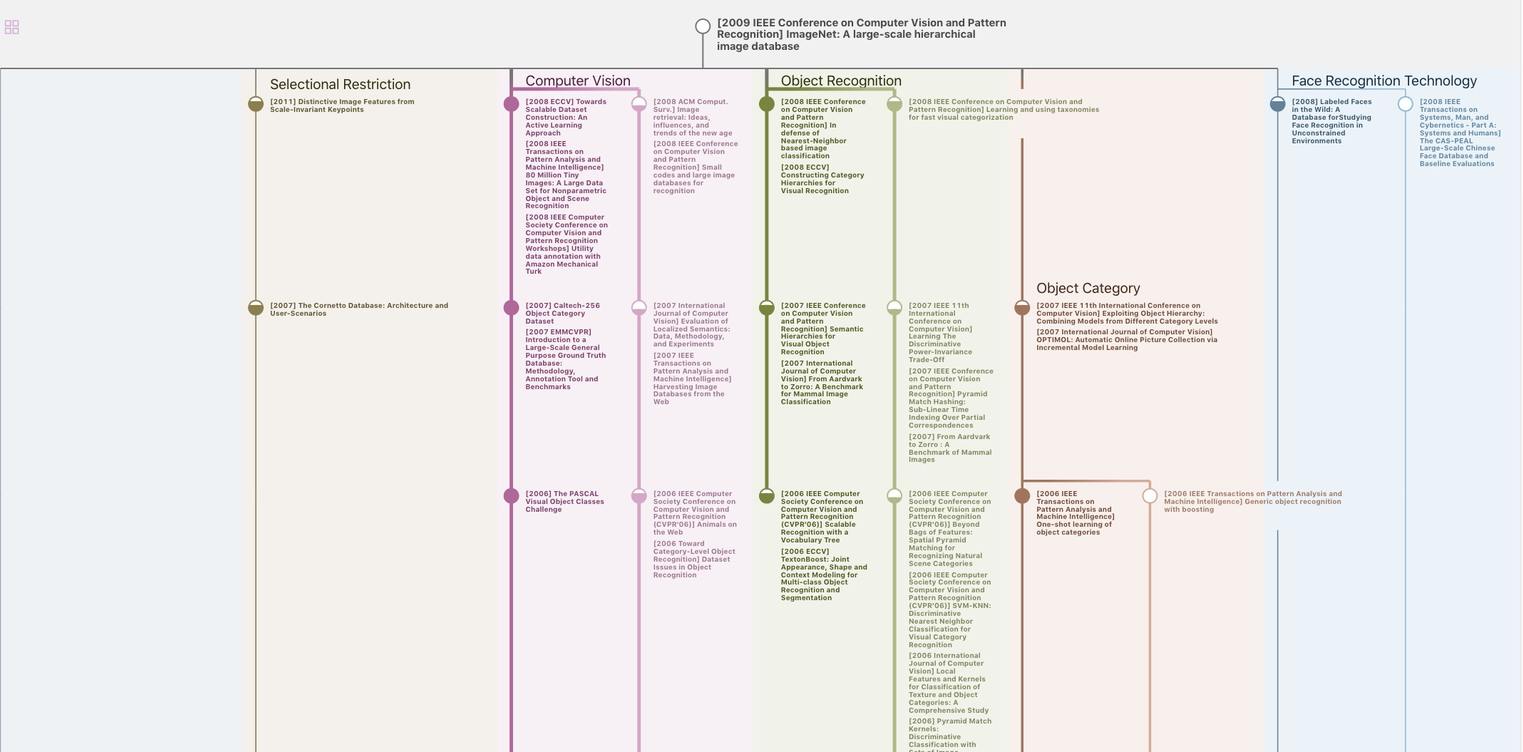
生成溯源树,研究论文发展脉络
Chat Paper
正在生成论文摘要