Understanding users’ requirements precisely: a double Bi-LSTM-CRF joint model for detecting user’s intentions and slot tags
Neural Computing and Applications(2022)
摘要
Understanding users’ requirements are essential to developing an effective AI service system, in which requirement expressions of users can be resolved into intent detection and slot filling tasks. In a lot of literature, the two tasks are normally considered as independent tasks and obtain satisfactory performance. Recently, many researchers have found that intent detection and slot filling can benefit each other since they always appear together in a sentence and may include shared information. Most of the existing joint models employ the structures of encoder and decoder and capture the cross-impact between two tasks by concatenation of hidden state information from two encoders, which ignore the dependencies among slot tags in specific intent. In this paper, we propose a novel Double-Bi-LSTM-CRF Model (DBLC), which can fit the dependency among hidden slot tags while considering the cross-impact between intent detection and slot filling. We also design and implement an intention chatbot on the tourism area, which can assist users to complete a travel plan through human-computer interaction. Extensive experiments show that our DBLC achieves state-of-the-art results on the benchmark ATIS, SNIPS, and multi-domain datasets.
更多查看译文
关键词
Intent detection, Slot filling, LSTM, Conditional random fields
AI 理解论文
溯源树
样例
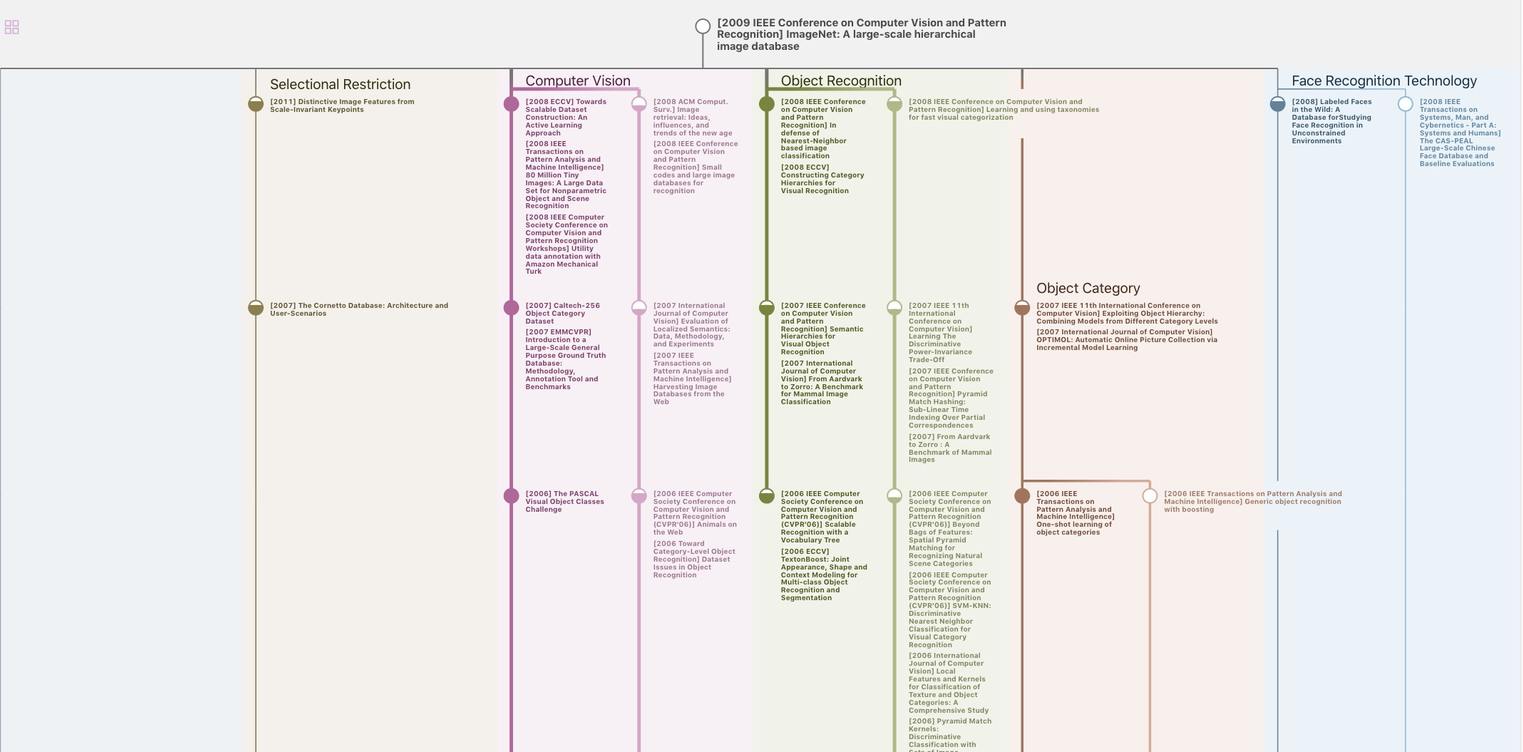
生成溯源树,研究论文发展脉络
Chat Paper
正在生成论文摘要