Map management for robust long-term visual localization of an autonomous shuttle in changing conditions
Multimedia Tools and Applications(2022)
摘要
Changes in appearance present a tremendous problem for the visual localization of an autonomous vehicle in outdoor environments. Data association between the current image and the landmarks in the map can be challenging in cases where the map was built with different environmental conditions. This paper introduces a solution to build and use multi-session maps incorporating sequences recorded in different conditions (day, night, fog, snow, rain, change of season, etc.). During visual localization, we exploit a ranking function to extract the most relevant keyframes from the map. This ranking function is designed to take into account the pose of the vehicle as well as the current environmental condition. In the mapping phase, covering all conditions by constantly adding data to the map leads to a continuous growth in the map size which in turn deteriorates the localization speed and performance. Our map management strategy is an incremental approach that aims to limit the size of the map while keeping it as diverse as possible. Our experiments were performed on real data collected with our autonomous shuttle as well as on a widely used public dataset. The results demonstrate that our keyframe-based ranking function is suitable for long-term scenarios. Our map management algorithm aims to build a map with as much diversity as possible whereas some state of the art approaches tend to filter out the less observed landmarks. This strategy shows a reduction of localization failures while maintaining real-time performance.
更多查看译文
关键词
Visual-based navigation,Computer vision for transportation,SLAM
AI 理解论文
溯源树
样例
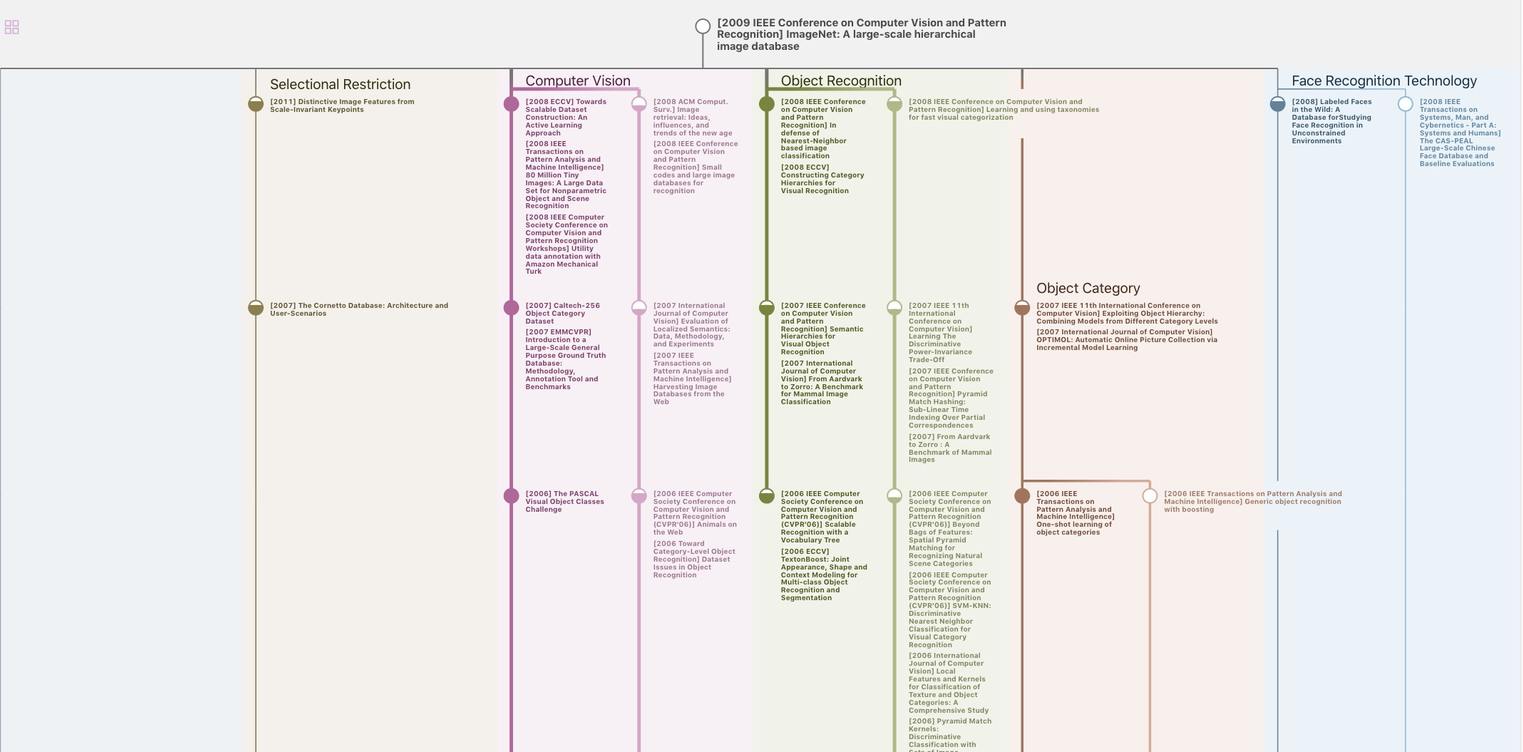
生成溯源树,研究论文发展脉络
Chat Paper
正在生成论文摘要