Spatially-Consistent Feature Matching and Learning for Heritage Image Analysis
International Journal of Computer Vision(2022)
摘要
Progress in the digitization of cultural assets leads to online databases that become too large for a human to analyze. Moreover, some analyses might be challenging, even for experts. In this paper, we explore two applications of computer vision to analyze historical data: watermark recognition and one-shot repeated pattern detection in artwork collections. Both problems present computer vision challenges which we believe to be representative of the ones encountered in cultural heritage applications: limited supervision is available, the tasks are fine-grained recognition, and the data comes in several different modalities. Both applications are also highly practical, as recognizing watermarks makes it possible to date and locate documents, while detecting repeated patterns allows exploring visual links between artworks. We demonstrate on both tasks the benefits of relying on deep mid-level features. More precisely, we define an image similarity score based on geometric verification of mid-level features and show how spatial consistency can be used to fine-tune out-of-the-box features for the target dataset with weak or no supervision. This paper relates and extends our previous works (Shen et al. in Discovering visual patterns in art collections with spatially-consistent feature learning, 2019; Shen et al. in Large-scale historical watermark recognition dataset and a new consistency-based approach, 2020). Our code and data are available at http://imagine.enpc.fr/~shenx/HisImgAnalysis/ .
更多查看译文
关键词
Feature learning,Self-supervised learning,Artwork analysis,Watermark recognition
AI 理解论文
溯源树
样例
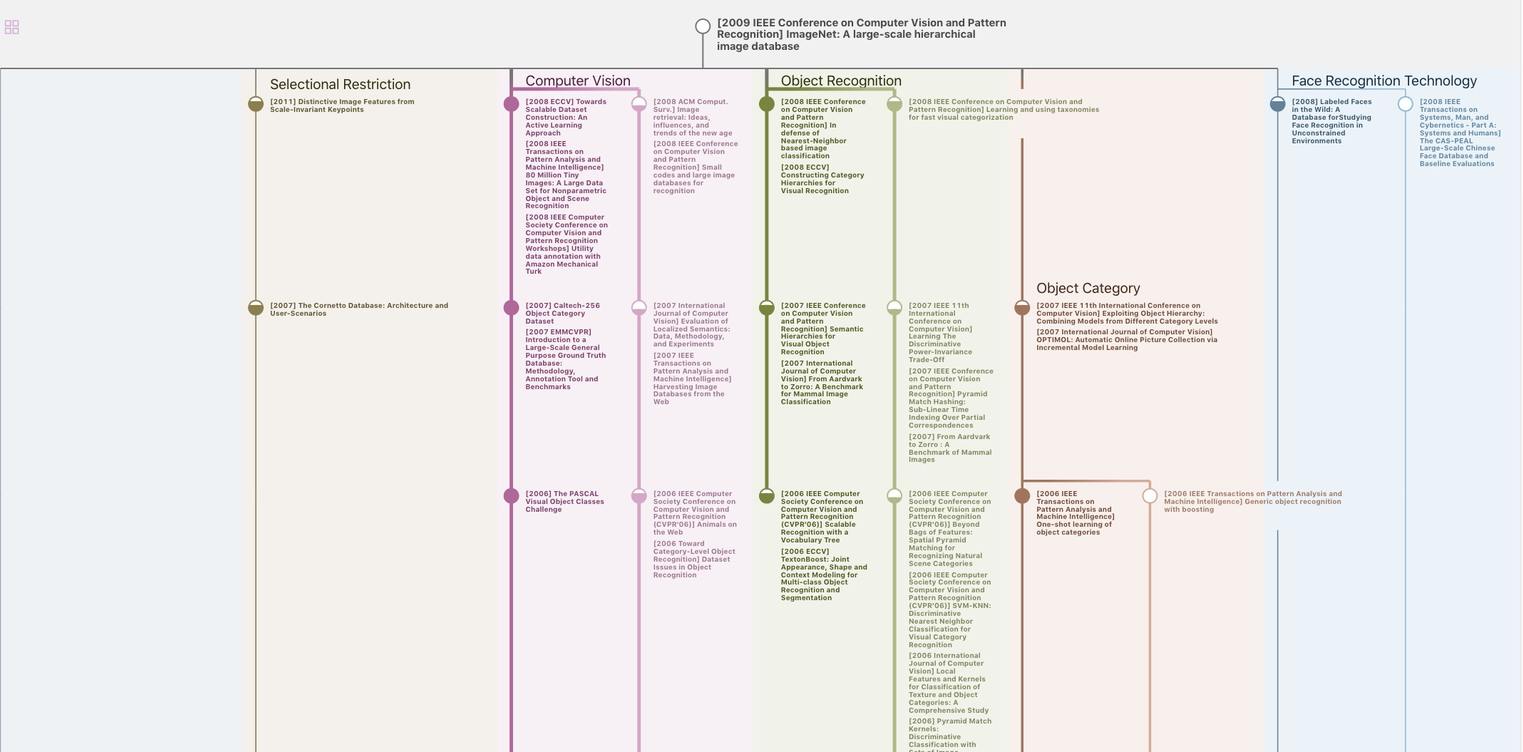
生成溯源树,研究论文发展脉络
Chat Paper
正在生成论文摘要