FG-Net: A Fast and Accurate Framework for Large-Scale LiDAR Point Cloud Understanding
IEEE Transactions on Cybernetics(2023)
摘要
This work presents FG-Net, a general deep learning framework for large-scale point cloud understanding without voxelizations, which achieves accurate and real-time performance with a single NVIDIA GTX 1080 8G GPU and an i7 CPU. First, a novel noise and outlier filtering method is designed to facilitate the subsequent high-level understanding tasks. For effective understanding purpose, we propose a novel plug-and-play module consisting of correlated feature mining and deformable convolution-based geometric-aware modeling, in which the local feature relationships and point cloud geometric structures can be fully extracted and exploited. For the efficiency issue, we put forward a new composite inverse density sampling (IDS)-based and learning-based operation and a feature pyramid-based residual learning strategy to save the computational cost and memory consumption, respectively. Compared with current methods which are only validated on limited datasets, we have done extensive experiments on eight real-world challenging benchmarks, which demonstrates that our approaches outperform state-of-the-art (SOTA) approaches in terms of accuracy, speed, and memory efficiency. Moreover, weakly supervised transfer learning is also conducted to demonstrate the generalization capacity of our method.
更多查看译文
关键词
3-D scene classification,3-D semantic segmentation,large-scale point cloud understanding,scene understanding in robotics,weakly supervised transfer learning
AI 理解论文
溯源树
样例
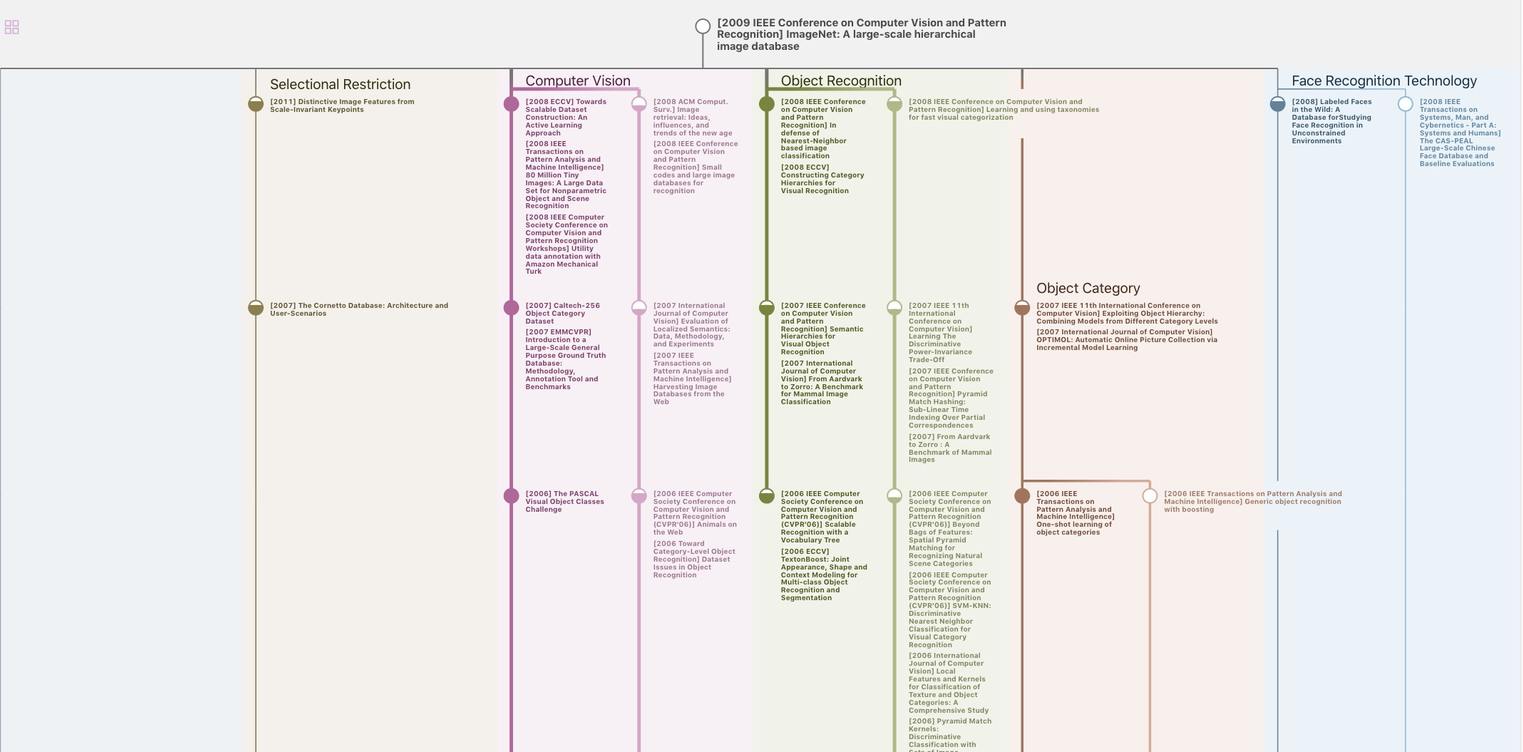
生成溯源树,研究论文发展脉络
Chat Paper
正在生成论文摘要