Massive Connectivity Over MIMO-OFDM: Joint Activity Detection and Channel Estimation With Frequency Selectivity Compensation
IEEE Transactions on Wireless Communications(2022)
摘要
In this paper, we study how to efficiently and reliably detect active devices and estimate their channels in a multiple-input multiple-output orthogonal frequency-division multiplexing (OFDM) based grant-free non-orthogonal multiple access system to enable massive machine-type communication (mMTC). First, by exploiting the correlation of the channel frequency responses across the OFDM subcarriers, we propose a block-wise linear channel model. Specifically, the continuous OFDM subcarriers are divided into several sub-blocks and a linear function with only two variables (mean and slope) is used to approximate the frequency-selective channel in each sub-block. This significantly reduces the number of variables to be determined in channel estimation, and the sub-block number can be adjusted to reliably compensate the channel frequency-selectivity. Second, we formulate the joint active device detection and channel estimation in the block-wise linear system as a Bayesian inference problem. By exploiting the block-sparsity of the channel matrix, we propose an efficient turbo message passing algorithm to solve the Bayesian inference problem. We then develop the state evolution to predict the performance of the turbo message passing algorithm. We further incorporate machine learning approaches into turbo message passing to learn unknown model parameters. Numerical results demonstrate the superior performance of the proposed algorithm over the state-of-the-art algorithms.
更多查看译文
关键词
Grant-free access,orthogonal frequency-division multiplexing,turbo message passing
AI 理解论文
溯源树
样例
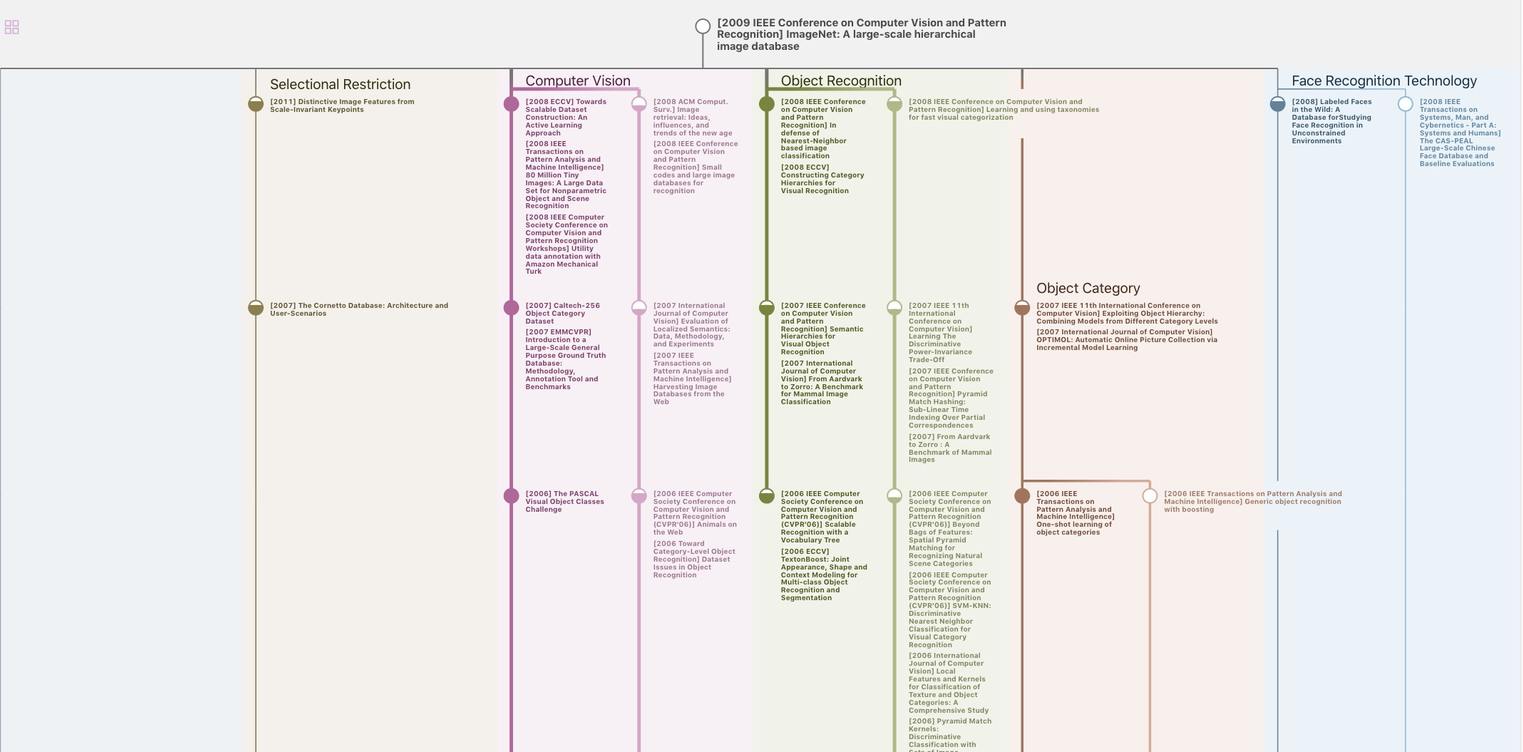
生成溯源树,研究论文发展脉络
Chat Paper
正在生成论文摘要