DFAID: Density-aware and feature-deviated active intrusion detection over network traffic streams
COMPUTERS & SECURITY(2022)
摘要
We study the problem of active intrusion detection over network traffic streams. Existing works create clusters for known classes and manually label instances outside the clusters for detecting novel attack classes and concept drift, yet several challenges are present. First, these methods assume that different classes of network traffic distribute far from each other in feature space, while similar attack classes could violate this assumption. It makes the true novel classes and concept drift undetectable, therefore a degraded performance. Second, prior works depending on heavily calculating and retraining cannot achieve efficient incremental updates over the infinite and high-speed streams of network traffic. Last, related methods rarely leverage the domain knowledge in intrusion detection. To address these issues, we propose DFAID, a Density-aware and Feature-deviated Active Intrusion Detection framework over network traffic streams. We first design the mask density score and the feature deviation score to maximize the effectiveness of labeled instances, effectively detecting novel attack classes and the concept drift when similar classes exist. Then, DFAID leverages robust incremental clustering structures to group instances in local regions, relieving the burden on the speed and reducing effects of noisy instances. Last, we further present DFAID-DK by incorporating the Domain Knowledge of temporal correlations between network attacks to correct the wrong predictions. Extensive experiments on two well-known benchmarks, CICIDS2017 and ISCX-2012, demonstrate that DFAID and its variation DFAID-DK both achieve significant improvement compared with related methods in terms of f1-score (21.7%, 22.7%) on average, and its running speed is an order of magnitude faster. 71585"> 2022 Elsevier Ltd. All rights reserved.
更多查看译文
关键词
Intrusion detection,Network traffic streams,Active learning,Incremental update,Domain knowledge
AI 理解论文
溯源树
样例
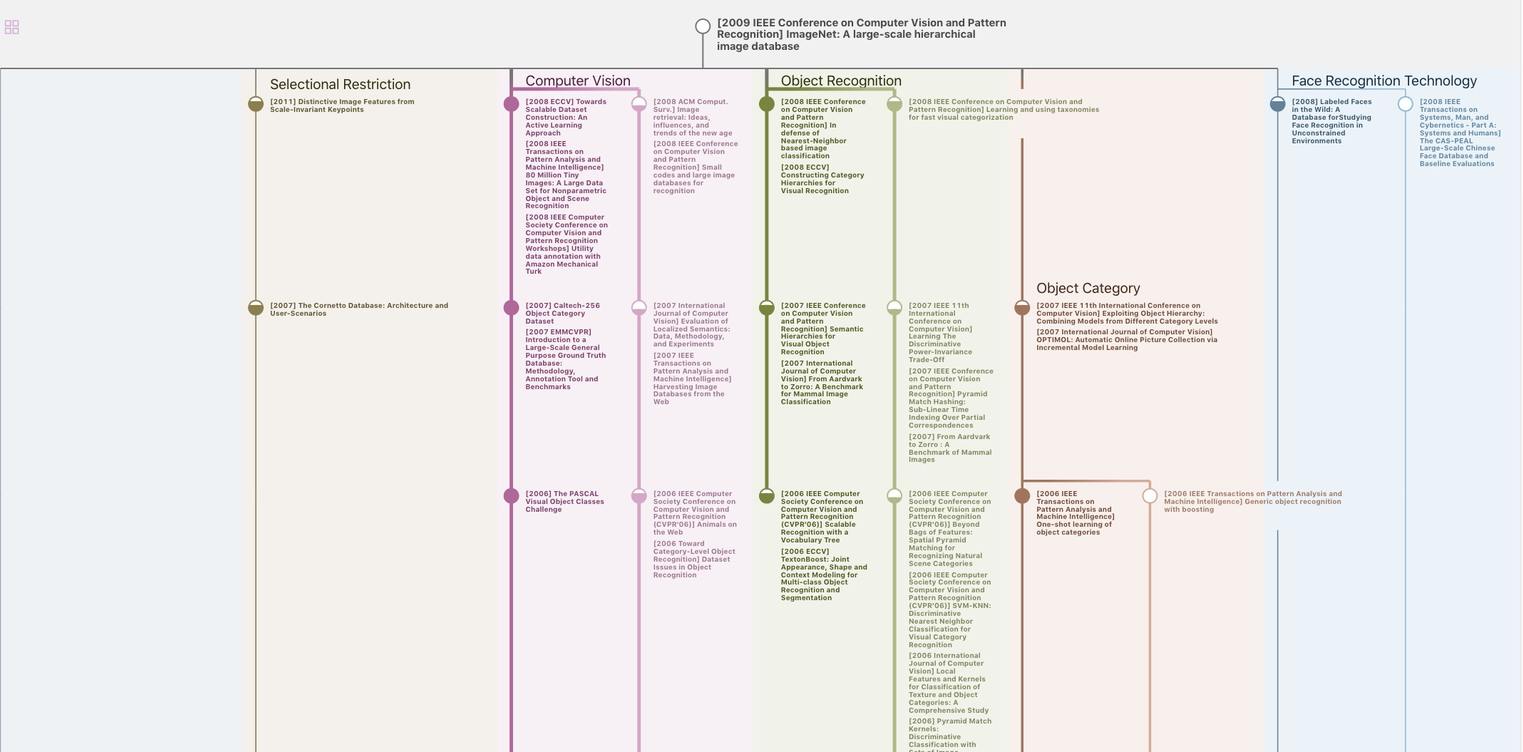
生成溯源树,研究论文发展脉络
Chat Paper
正在生成论文摘要