DCCA and DMCCA framework for multimodal biometric system
Multimedia Tools and Applications(2022)
摘要
Deep networks have been successfully applied to unsupervised feature learning for single modalities such as Text, Image, or Audio. Here, we present the Deep Network that learns nonlinear transformations of two or more biometric modalities. The resultant representation is a combination of maximally correlated features of the input modalities. It can be viewed as a feature fusion technique with the nonlinear extension of the linear multiset canonical correlation analysis (MCCA). The Foundation of a multibiometric system is information fusion. Feature level fusion is an important area of research owing to the information richness of features. This Deep learning model outlines the integrated correlation among multiple feature vectors and forms a new fused feature vector. This paper presents the Deep Canonical Correlation Analysis (DCCA) for bimodal biometric system and Deep Multiset Canonical Correlation Analysis (DMCCA) framework for the multimodal biometric system outperforming the traditional CCA based fusion methods. Experiments are carried out with the SDUMLA-HMT multimodal dataset. Using the proposed DCCA method performance of a bi-modal biometric system with iris and fingerprint modalities in terms of EER (equal error rate) is 3.78% and 0.116% (single modality recognition). While for the multibiometric system with four modalities (iris, fingerprint, finger-vein, and face) with DMCCA, EER is 0.717%.
更多查看译文
关键词
Fusion techniques, Deep learning, Canonical correlation analysis
AI 理解论文
溯源树
样例
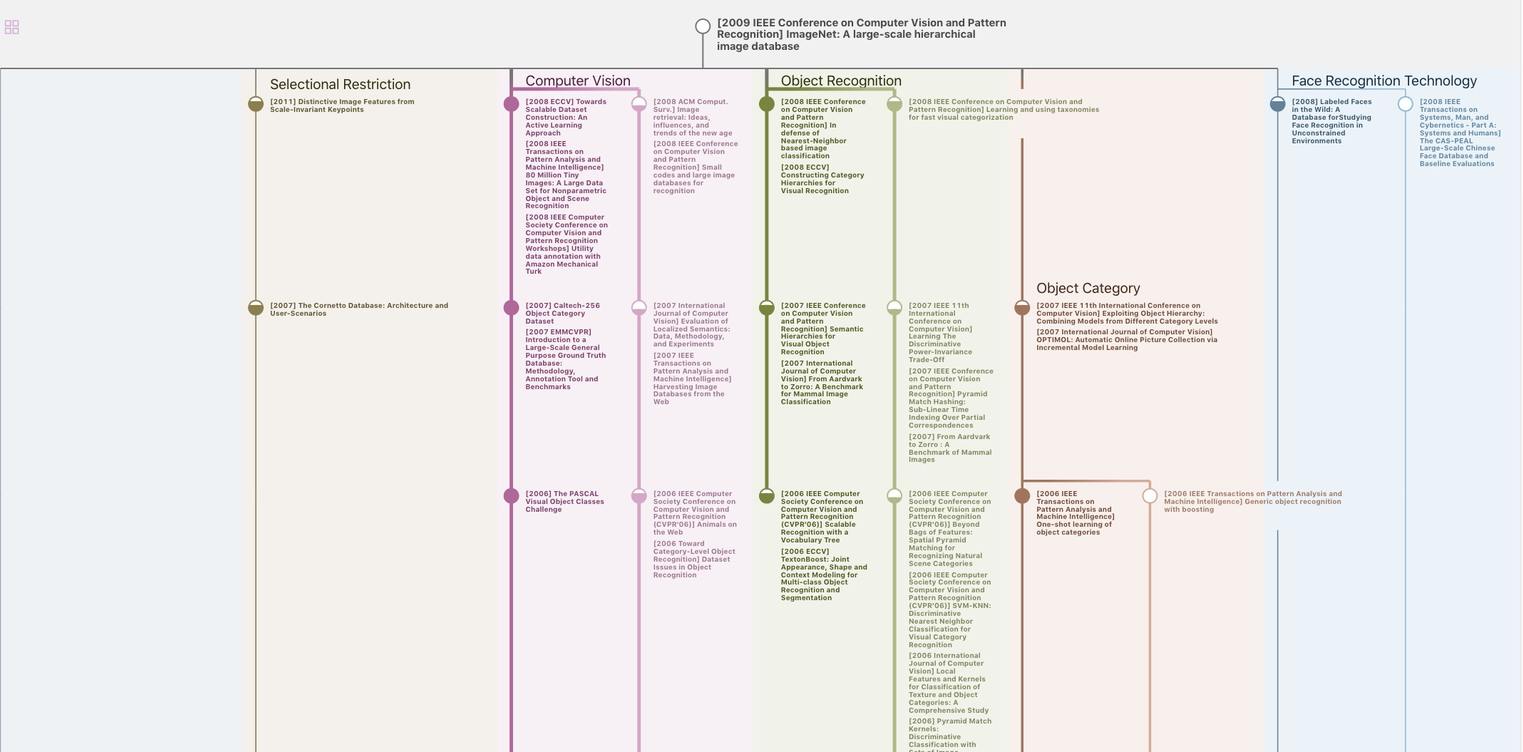
生成溯源树,研究论文发展脉络
Chat Paper
正在生成论文摘要