Predicting the abundances of aphids and their natural enemies in cereal crops: Machine-learning versus linear models
BIOLOGICAL CONTROL(2023)
摘要
Predicting the effects of crop management and landscape structure on biological pest control is a key challenge for implementing innovative pest management systems. Here, we compare the performances of two machine-learning methods (regression trees and random forests) with those of linear models in predicting cereal aphid abundance, parasitism, and natural enemies. We trained models using data describing the landscapes, cropping systems, and margins of 88 cereal fields in France. To assess the predictive performances of our models, we measured pseudo-R-2 and root-mean-square error using cross-validation. The two machine-learning methods performed reasonably well in comparison with linear models. Aphid abundance, parasitism, and natural enemies were predicted by both biological (abundances of functional groups) and environmental variables (describing fields, crop management and landscape structure). Parasitoids and hoverfly larvae, unlike coccinellid and lacewing larvae, presented a density-dependent numerical response to aphid populations. At the landscapes scale, linear elements (both grassy strips and hedgerows) were the most important predictors of aphid abundance and parasitism. In contrast, hoverfly larvae were more influenced by the proportion of cultivated areas and woodlands. At the field scale, our models ranked factors related to field size, margins, and soil type as the most important predictors of aphids and their natural enemies. Our analysis shows that machine-learning models outperform classical statistical ones, and therefore provide useful tools for predicting highly variable ecosystem services such as biological pest control.
更多查看译文
关键词
Conservation biological control,Landscape structure,Density-dependent parasitism,Random forests,Cross-validation,Model generalizability
AI 理解论文
溯源树
样例
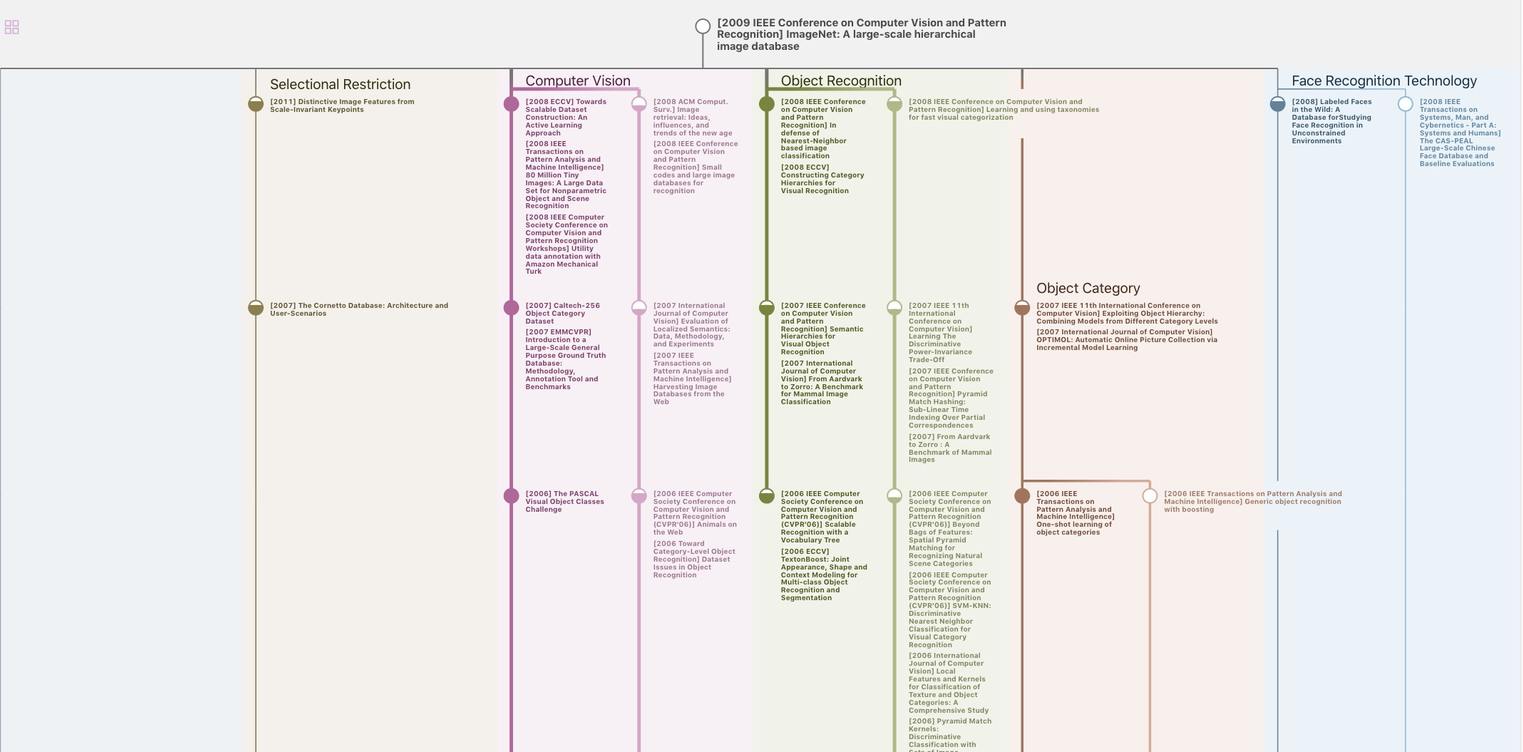
生成溯源树,研究论文发展脉络
Chat Paper
正在生成论文摘要