Side-effects of Learning from Low Dimensional Data Embedded in an Euclidean Space.
CoRR(2022)
Abstract
The low dimensional manifold hypothesis posits that the data found in many applications, such as those involving natural images, lie (approximately) on low dimensional manifolds embedded in a high dimensional Euclidean space. In this setting, a typical neural network defines a function that takes a finite number of vectors in the embedding space as input. However, one often needs to consider evaluating the optimized network at points outside the training distribution. This paper considers the case in which the training data is distributed in a linear subspace of Rd. We derive estimates on the variation of the learning function, defined by a neural network, in the direction transversal to the subspace. We study the potential regularization effects associated with the network’s depth and noise in the codimension of the data manifold. We also present additional side effects in training due to the presence of noise.
MoreTranslated text
Key words
learning,data,space,low-dimensional
AI Read Science
Must-Reading Tree
Example
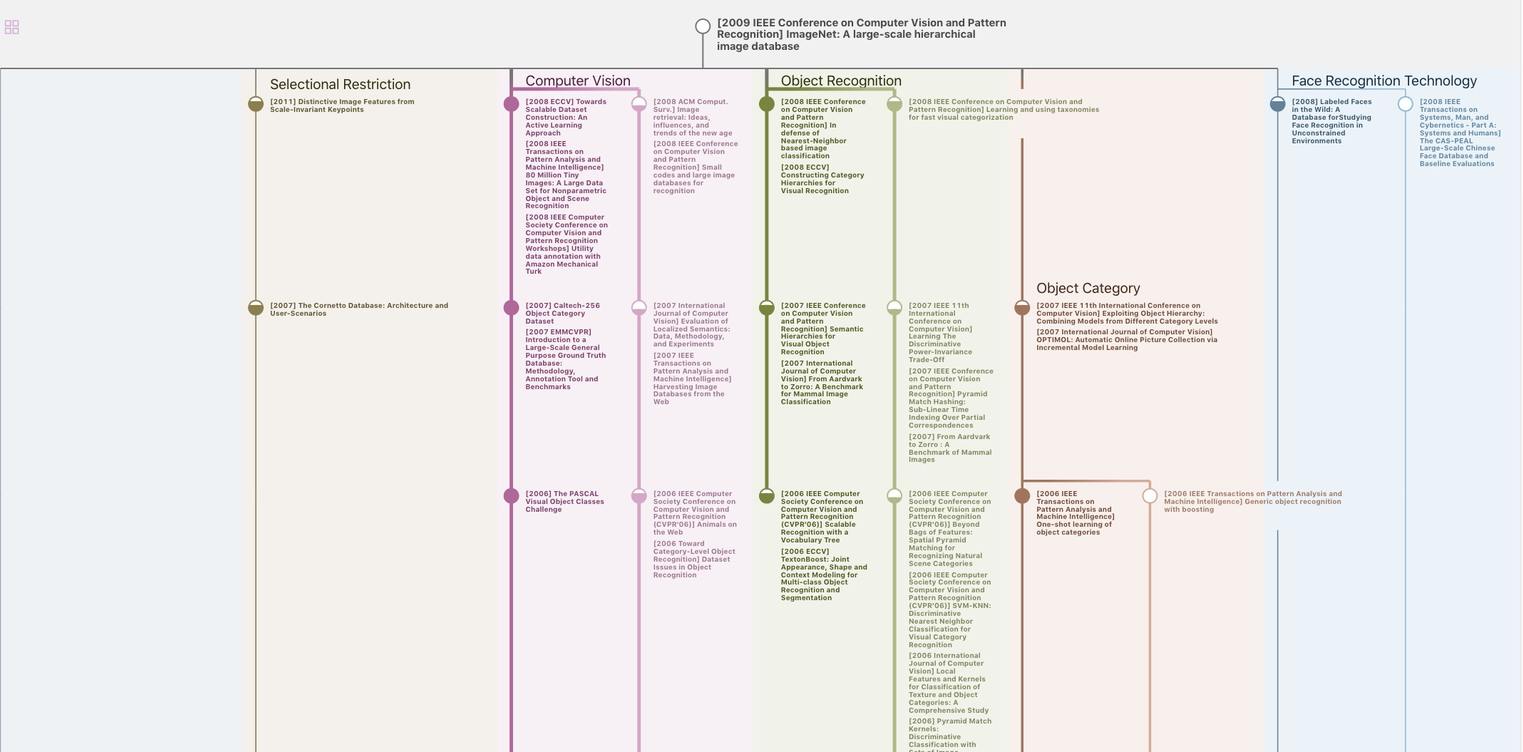
Generate MRT to find the research sequence of this paper
Chat Paper
Summary is being generated by the instructions you defined