Differential Privacy and Swapping: Examining De-Identification’s Impact on Minority Representation and Privacy Preservation in the U.S. Census
2022 IEEE Symposium on Security and Privacy (SP)(2022)
摘要
There has been considerable controversy regarding the accuracy and privacy of de-identification mechanisms used in the U.S. Decennial Census. We theoretically and experimentally analyze two such classes of mechanisms, swapping and differential privacy, especially examining their effects on ethnoracial minority groups.We first prove that the expected error of queries made on swapped demographic datasets is greater in sub-populations whose racial distributions differ more from the racial distribution of the global population. We also prove that the probability that m unique entries exist in a sub-population shrinks exponentially as the sub-population size grows. These properties suggest that swapping, which prioritizes unique entries, will produce poor accuracy for minority groups.We then empirically analyze the impact of swapping and differential privacy on the accuracy and privacy of a demographic dataset. We evaluate accuracy in several ways, including methods that stress the effect on minority groups. We evaluate privacy by counting the number of re-identified entries in a simulated linkage attack. Finally, we explore the disproportionate presence of minority groups in identified entries.Our empirical lindings corroborate our theoretical results: for minority representation, the utility of differential privacy is comparable to the utility of swapping, while providing a stronger privacy guarantee. Swapping places a disproportionate privacy burden on minority groups, whereas an ε-differentially private mechanism is ε-differentially private for all subgroups.
更多查看译文
关键词
Differential-privacy,census,data-swapping
AI 理解论文
溯源树
样例
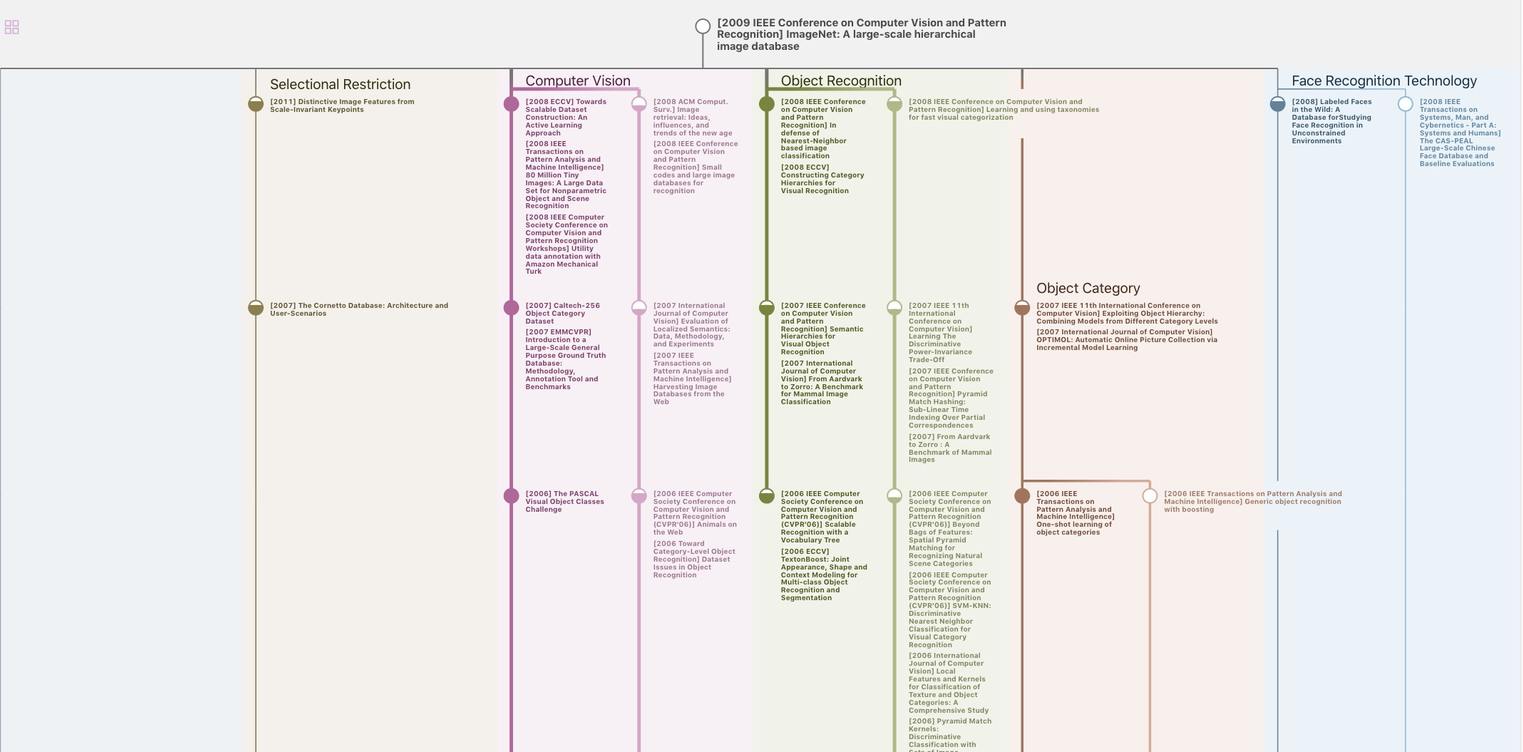
生成溯源树,研究论文发展脉络
Chat Paper
正在生成论文摘要