Segmentation stability of human head and neck cancer medical images for radiotherapy applications under de-identification conditions: benchmarking data sharing and artificial intelligence use-cases
Frontiers in oncology(2023)
摘要
Background Demand for head and neck cancer (HNC) radiotherapy data in algorithmic development has prompted increased image dataset sharing. Medical images must comply with data protection requirements so that re-use is enabled without disclosing patient identifiers. Defacing, i.e., the removal of facial features from images, is often considered a reasonable compromise between data protection and re-usability for neuroimaging data. While defacing tools have been developed by the neuroimaging community, their acceptability for radiotherapy applications have not been explored. Therefore, this study systematically investigated the impact of available defacing algorithms on HNC organs at risk (OARs).
Methods A publicly available dataset of magnetic resonance imaging scans for 55 HNC patients with eight segmented OARs (bilateral submandibular glands, parotid glands, level II neck lymph nodes, level III neck lymph nodes) was utilized. Eight publicly available defacing algorithms were investigated: afni\_refacer, DeepDefacer, defacer, fsl\_deface, mask\_face, mri\_deface, pydeface, and quickshear. Using a subset of scans where defacing succeeded (N=29), a 5-fold cross-validation 3D U-net based OAR auto-segmentation model was utilized to perform two main experiments: 1.) comparing original and defaced data for training when evaluated on original data; 2.) using original data for training and comparing the model evaluation on original and defaced data. Models were primarily assessed using the Dice similarity coefficient (DSC).
Results Most defacing methods were unable to produce any usable images for evaluation, while mask\_face, fsl\_deface, and pydeface were unable to remove the face for 29%, 18%, and 24% of subjects, respectively. When using the original data for evaluation, the composite OAR DSC was statistically higher (p ≤ 0.05) for the model trained with the original data with a DSC of 0.760 compared to the mask\_face, fsl\_deface, and pydeface models with DSCs of 0.742, 0.736, and 0.449, respectively. Moreover, the model trained with original data had decreased performance (p ≤ 0.05) when evaluated on the defaced data with DSCs of 0.673, 0.693, and 0.406 for mask\_face, fsl\_deface, and pydeface, respectively.
Conclusion Defacing algorithms may have a significant impact on HNC OAR auto-segmentation model training and testing. This work highlights the need for further development of HNC-specific image anonymization methods.
### Competing Interest Statement
C.D.F. has received direct industry grant support, speaking honoraria, and travel funding from Elekta AB. The other authors have no conflicts of interest to disclose.
### Funding Statement
This work is supported by the International Laboratory of Social Neurobiology ICN HSE RF Government grant ag. No. 075 15 2019 1930 (to E.G.). This work was also supported by the National Institutes of Health (NIH)/National Cancer Institute (NCI) through a Cancer Center Support Grant (CCSG; P30CA016672-44). M.A.N. is supported by an NIH grant (R01DE028290-01). K.A.W. is supported by a training fellowship from The University of Texas Health Science Center at Houston Center for Clinical and Translational Sciences TL1 Program (TL1TR003169), the American Legion Auxiliary Fellowship in Cancer Research, and an NIH/National Institute for Dental and Craniofacial Research (NIDCR) F31 fellowship (1 F31DE031502-01). C.D.F. received funding from the NIH/NIDCR (1R01DE025248-01/R56DE025248); an NIH/NIDCR Academic-Industrial Partnership Award (R01DE028290); the National Science Foundation (NSF), Division of Mathematical Sciences, Joint NIH/NSF Initiative on Quantitative Approaches to Biomedical Big Data (QuBBD) Grant (NSF 1557679); the NIH Big Data to Knowledge (BD2K) Program of the NCI Early Stage Development of Technologies in Biomedical Computing, Informatics, and Big Data Science Award (1R01CA214825); the NCI Early Phase Clinical Trials in Imaging and Image-Guided Interventions Program (1R01CA218148); an NIH/NCI Pilot Research Program Award from the UT MD Anderson CCSG Radiation Oncology and Cancer Imaging Program (P30CA016672); an NIH/NCI Head and Neck Specialized Programs of Research Excellence (SPORE) Developmental Research Program Award (P50CA097007); and the National Institute of Biomedical Imaging and Bioengineering (NIBIB) Research Education Program (R25EB025787).
### Author Declarations
I confirm all relevant ethical guidelines have been followed, and any necessary IRB and/or ethics committee approvals have been obtained.
Yes
The details of the IRB/oversight body that provided approval or exemption for the research described are given below:
The study involves only openly avalailbe human data, which can be obtained from: https://wiki.cancerimagingarchive.net/display/Public/AAPM+RT-MAC+Grand+Challenge+2019. As per the corresponding description of this dataset: "Images of patients were retrospectively retrieved under an Institutional Review Board approved protocol and waiver of informed consent (RCR08-0300).”
I confirm that all necessary patient/participant consent has been obtained and the appropriate institutional forms have been archived, and that any patient/participant/sample identifiers included were not known to anyone (e.g., hospital staff, patients or participants themselves) outside the research group so cannot be used to identify individuals.
Yes
I understand that all clinical trials and any other prospective interventional studies must be registered with an ICMJE-approved registry, such as ClinicalTrials.gov. I confirm that any such study reported in the manuscript has been registered and the trial registration ID is provided (note: if posting a prospective study registered retrospectively, please provide a statement in the trial ID field explaining why the study was not registered in advance).
Yes
I have followed all appropriate research reporting guidelines and uploaded the relevant EQUATOR Network research reporting checklist(s) and other pertinent material as supplementary files, if applicable.
Yes
All data produced in the present study are available upon reasonable request to the authors.
更多查看译文
关键词
MRI,anonymization,artificial intelligence (AI),autosegmentation,defacing,head and neck cancer,medical imaging,radiotherapy
AI 理解论文
溯源树
样例
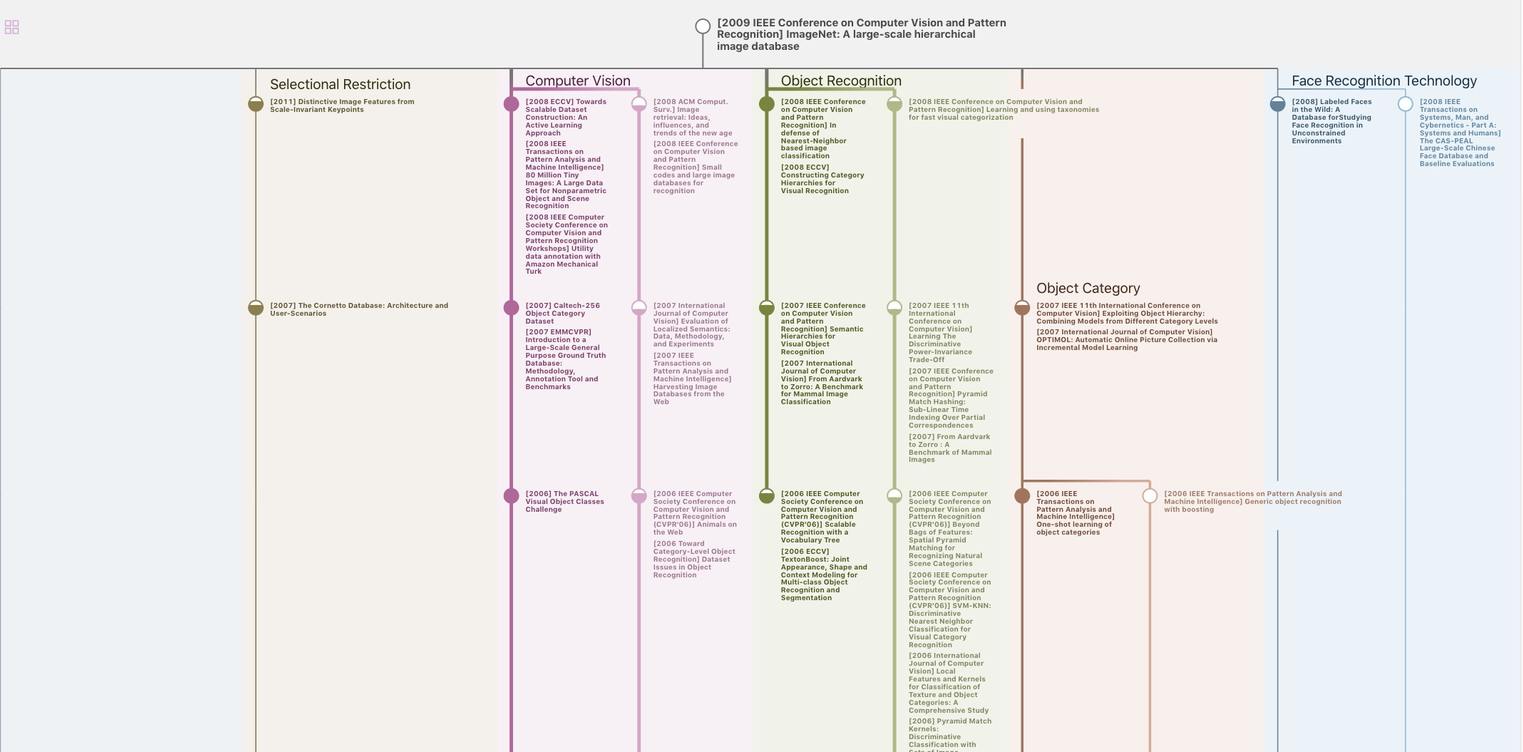
生成溯源树,研究论文发展脉络
Chat Paper
正在生成论文摘要