Error bounds for approximations with deep ReLU neural networks in Sobolev norms
semanticscholar(2019)
摘要
Despite the overwhelming success of deep neural networks in various applications [1]–[7], a comprehensive mathematical explanation of this success has not yet been found. Many attempts at unraveling the extreme efficiency of deep neural networks have been made in the context of approximation theory [8]–[13]. One of the applications of deep learning where a strong knowledge of the approximation capabilities of neural networks directly translates into quantifiable theoretical results appears when solving partial differential equations (PDEs) using deep learning techniques. Some notable advances in this direction have been made in [14]– [20]. In this regard, not only the approximation fidelity with respect to standard Lebesgue norms is of high interest, but also that with respect to Sobolev-type norms. First results in this direction were reported in [21]. In the paper [22] and this note, we derive upper and lower complexity bounds for approximations of Sobolev-regular functions by deep neural networks where the approximation error is measured with respect to weaker Sobolev norms. This extends results from [23], where Yarotsky considered approximations of Sobolev regular functions in L∞-norm. Furthermore we show that there is a trade-off between the regularity used in the norm in which the approximation error is measured and the complexity of the neural network. We expect our results to lead to complexity bounds for ReLU networks used in the numerical solution of PDEs.
更多查看译文
AI 理解论文
溯源树
样例
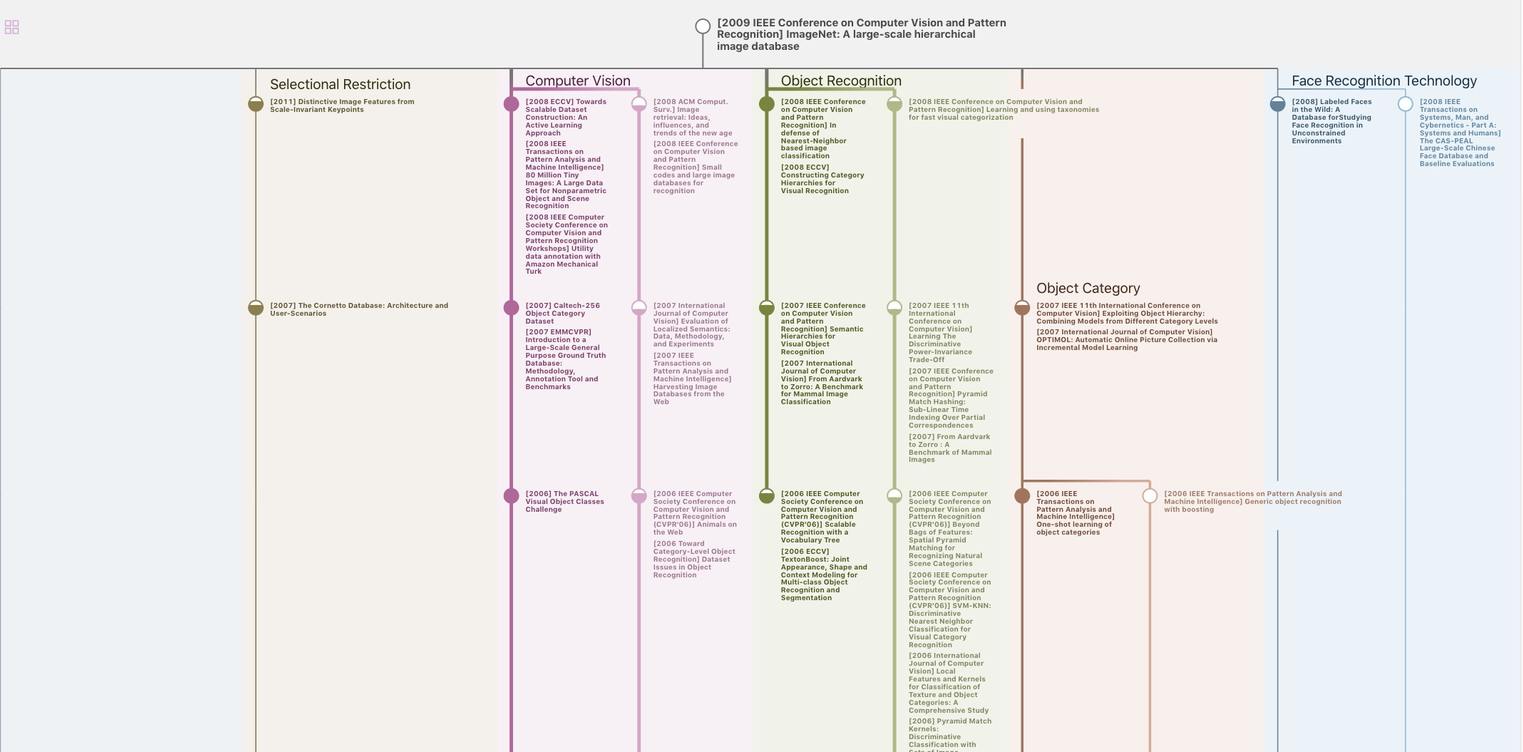
生成溯源树,研究论文发展脉络
Chat Paper
正在生成论文摘要