Paediatric major incident triage and the use of machine learning techniques to develop an alternative triage tool with improved performance characteristics.
medRxiv(2021)
摘要
Background Triage is a key principle in the effective management of major incidents. However, there is an increasing body of evidence demonstrating that existing paediatric methods are associated with high rates of under-triage and are not fit for purpose. The aim of this study was to derive a novel paediatric triage tool using machine learning (ML) techniques. Methods The United Kingdom Trauma Audit Research Network (TARN) database was interrogated for all paediatric patients aged under 16 years for the ten-year period 2008-2017. Patients were categorised as Priority One if they received one or more life-saving interventions from a previously defined list. Six ML algorithms were investigated for identifying patients as Priority One. Subsequently, the best performing model was chosen for further development using a risk score approach and clinically relevant modifications in order to derive a novel triage tool (LASSO M2). Using patients with complete pre-hospital physiological data, a comparative analysis was then performed comparing this to existing pre-hospital paediatric major incident triage tools. Performance was evaluated using sensitivity, specificity, under-triage (1-sensitivity) and over-triage (1-positive predictive value). Results Complete physiological data were available for 4962 patients. The LASSO M2 model demonstrated the best performance at identifying paediatric patients in need of life-saving intervention, sensitivity 88.8% (95% CI 85.5, 91.5) and was associated with the lowest rate of under-triage, 11.2% (8.5, 14.5). In contrast, the Paediatric Triage Tape and JumpSTART both had poor sensitivity when identifying those requiring life-saving intervention (36.1% (31.8, 40.7) and 44.7% (40.2, 49.4)) respectively. Conclusion The ML derived triage tool (LASSO M2) outperforms existing methods of paediatric major incident triage at identifying patients in need of life-saving intervention. Prior to its recommendation for clinical use, further work is required to externally validate its performance and undertake a feasibility assessment in a clinical context.
更多查看译文
AI 理解论文
溯源树
样例
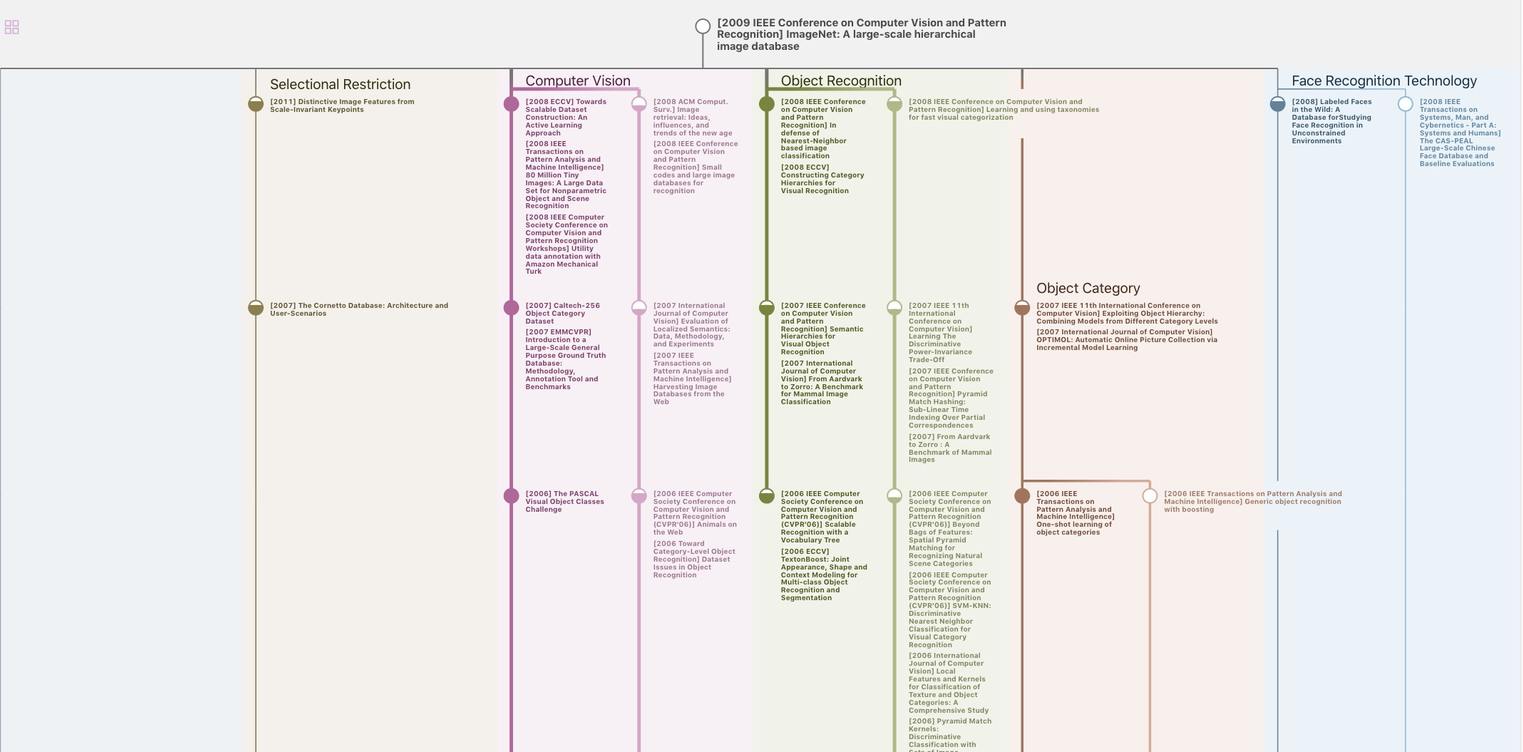
生成溯源树,研究论文发展脉络
Chat Paper
正在生成论文摘要