SMARTADAPT: Multi-branch Object Detection Framework for Videos on Mobiles
2022 IEEE/CVF CONFERENCE ON COMPUTER VISION AND PATTERN RECOGNITION (CVPR 2022)(2022)
摘要
Several recent works seek to create lightweight deep networks for video object detection on mobiles. We observe that many existing detectors, previously deemed computationally costly for mobiles, intrinsically support adaptive inference, and offer a multi-branch object detection framework (MBODF). Here, an MBODF is referred to as a solution that has many execution branches and one can dynamically choose from among them at inference time to satisfy varying latency requirements (e.g. by varying resolution of an input frame). In this paper, we ask, and answer, the wide-ranging question across all MBODFs: How to expose the right set of execution branches and then how to schedule the optimal one at inference time? In addition, we uncover the importance of making a content-aware decision on which branch to run, as the optimal one is conditioned on the video content. Finally, we explore a content-aware scheduler, an Oracle one, and then a practical one, leveraging various lightweight feature extractors. Our evaluation shows that layered on Faster R-CNN-based MBODF, compared to 7 baselines, our SMARTADAPT achieves a higher Pareto optimal curve in the accuracy-vs-latency space for the ILSVRC VID dataset.
更多查看译文
关键词
Vision applications and systems,Efficient learning and inferences,Machine learning,Motion and tracking,Recognition: detection,categorization,retrieval
AI 理解论文
溯源树
样例
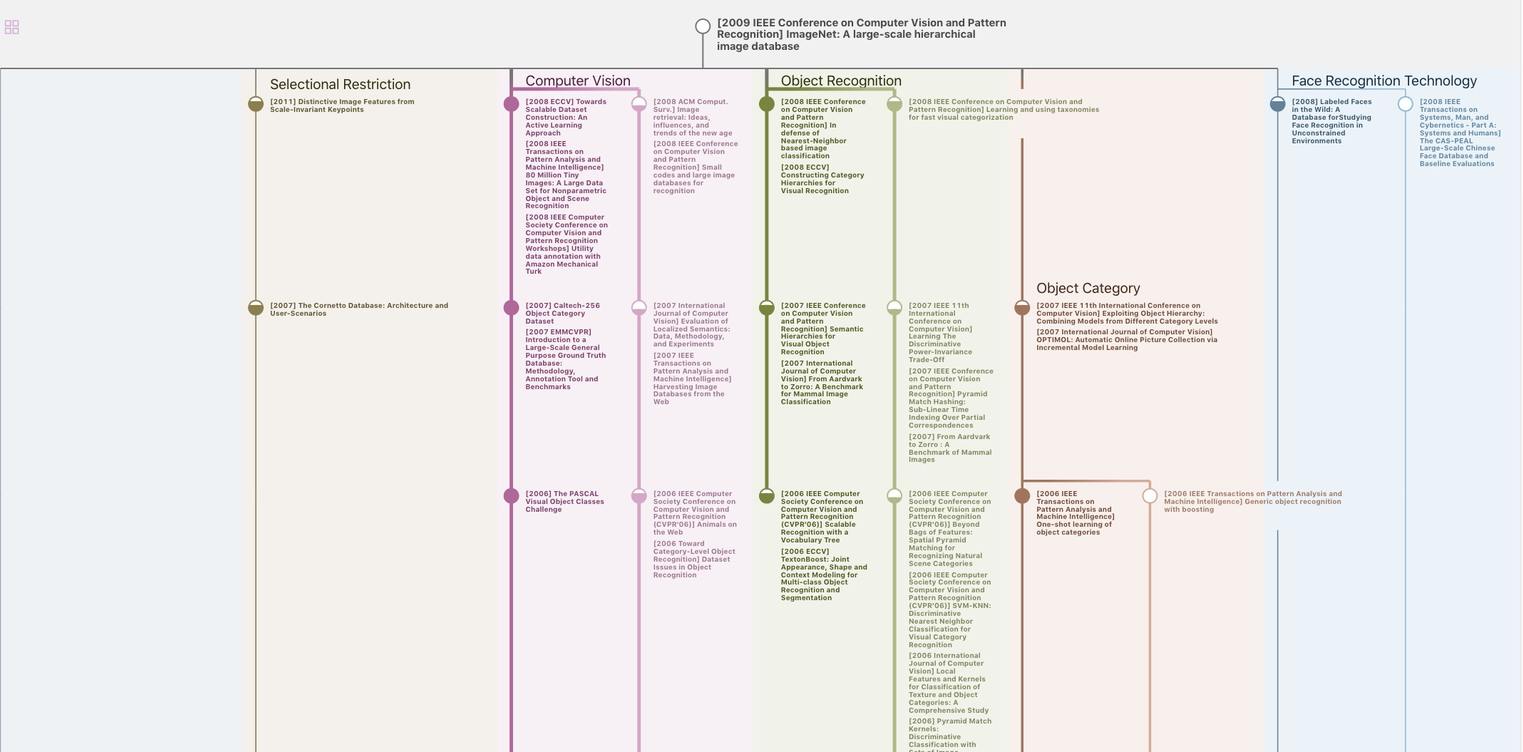
生成溯源树,研究论文发展脉络
Chat Paper
正在生成论文摘要