Machine tool thermal state representation using modal analysis
semanticscholar(2021)
摘要
Thermally induced deformations degrade the performance of machine tools leading to dimensional errors in manufactured products. Therefore, models are often used to map related observed data such as temperature of key points of the structure to the resultant thermal errors. Predictions from these models are then factored in to the controller commands to offset the errors. However, these data driven models can only learn from the experiences recorded in their training data. Therefore, being able to quantify the state of the machine tool from the data can lead to better modelling results. This work proposes a novel approach for representing the thermal state of a machine tool. Modal analysis and K-Means clustering are used to extract the descriptor Proper Orthogonal Decomposition (POD) modes in the temeprature data which encode the thermal state of the machine tool. These descriptor POD modes identify the different conditions experienced during machining. These features are then used in determining whether any future observed data contains thermal states in the training process. The results obtained show that the appraoch is able to quantify the differences in the machine’s thermal state. These finding will be used to improve thermal error modelling in machine tools.
更多查看译文
AI 理解论文
溯源树
样例
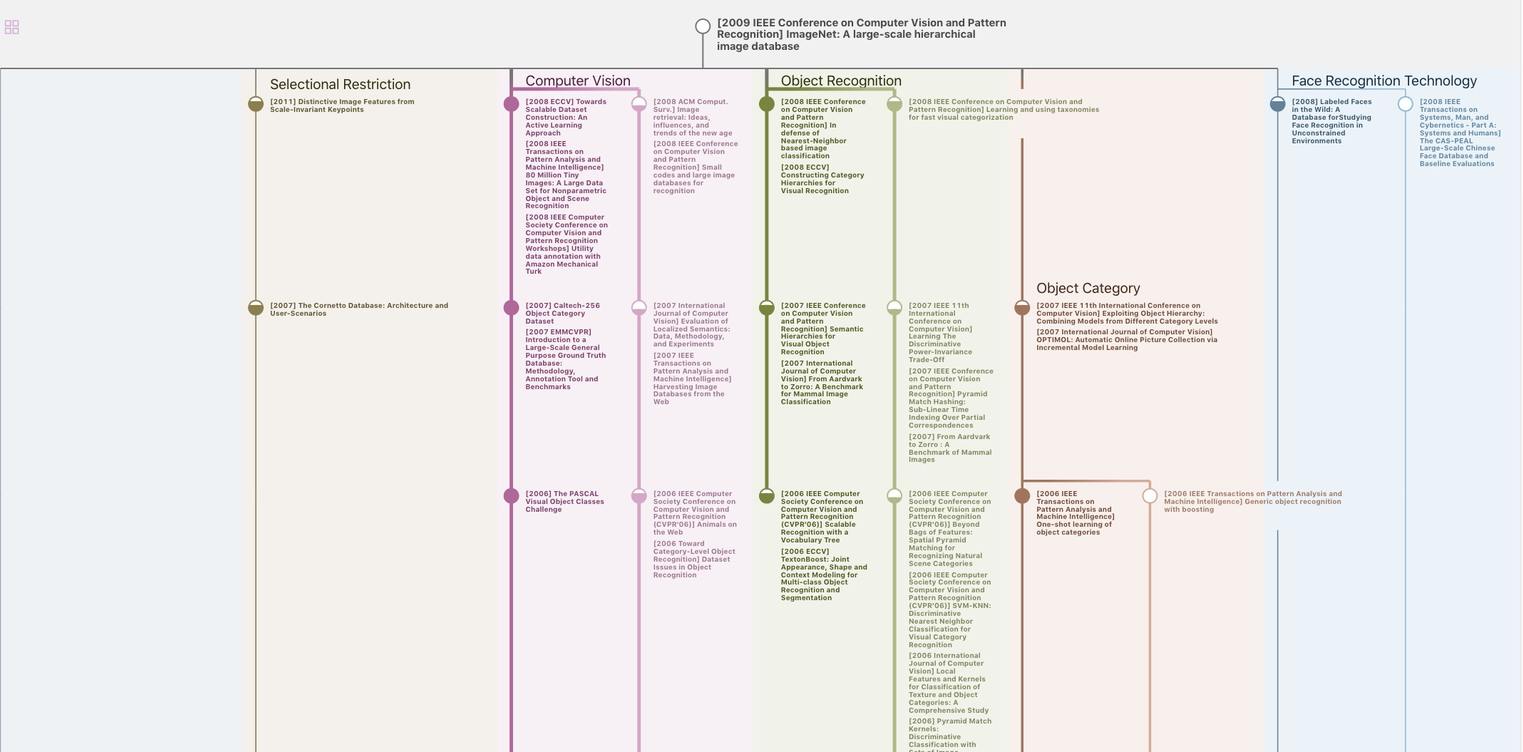
生成溯源树,研究论文发展脉络
Chat Paper
正在生成论文摘要