Estimating crop lower limit on Vertosol soils in the presence of variable levels of subsoil salinity
semanticscholar(2021)
Abstract
We have developed methods to estimate plant available water capacity (PAWC) and crop lower limit (CLL) based on soil and landscape information. These methods require adjustment for subsoil constraints, which tend to reduce PAWC. For example, subsoil salinity, indicated by high chloride concentration, typically increases CLL towards the drained upper limit (DUL), thereby reducing PAWC. We define this phenomenon “fractional closure” (FC). We analysed the effect of chloride on the FC of PAWC for five crops commonly grown in Vertosols in Australia. Our analysis successfully reflected the different sensitivities of bread wheat and chickpea to subsoil chloride, but showed barley, durum and canola were similar to bread wheat. Estimation of crop-specific CLL, thence PAWC, for varying levels of subsoil chloride will enable improved crop management decisions. Introduction Knowledge of PAWC can assist growers and advisers through improving the quality of yield forecasts and informing crop management decisions including if, when and what crop to sow and the level of fertiliser inputs required for the expected production potential at that site. The APSoil database comprises PAWC characterisations, determined in situ by measuring DUL and CLL (Burk and Dalgliesh 2013), for ~1100 sites in Australia. However, in situ determination of PAWC is labour intensive and often impractical, so methods to estimate PAWC using soil and landscape information have been developed (Verburg et al 2020). PAWC, including DUL and CLL, can be predicted by combining soil attribute layers from digital soil maps and pedotransfer functions or by using soillandscape information to find an existing PAWC characterisation for a similar soil and crop (e.g. in Australia from the APSoil database). Either way, the estimated CLL, a property of both the soil and the crop, will need to be adjusted for local conditions, such as accounting for the presence and severity of subsoil constraints. For Vertosols in northern NSW and QLD, subsoil salinity, as indicated by high chloride levels (Dang et al 2006), is typically associated with an increase in CLL, thus narrowing the difference between CLL and DUL and reducing the PAWC (Dang et al. 2006b, 2008; Hochman et al., 2007). Chloride concentrations often increase with increasing depth in the soils of the northern grain growing region, thus the impact on reduced PAWC is typically greater with depth. The focus of this study was to develop a method for estimating the extent of PAWC reduction in response to subsoil chloride concentration and explore crop differences therein. We built on an approach earlier used by Hochman et al (2007) and Dang et al (2006b) of first predicting the CLL for unconstrained conditions and then assessing the necessary adjustments to account for increased chloride concentration. Dang et al (2006b) estimated the unconstrained CLL based on particle size analysis. These predictions contain some uncertainty and ignore the fact that the CLL will also taper on account of reduced rooting density with depth. Hochman et al (2007), in a study for wheat only, derived a depth function for unconstrained CLL, but found little improvement in predicted CLL when adding the chloride constraint. Herein, we rederive a depth function for unconstrained CLL, based strictly on PAWC profiles without constraints, and then extend the analysis of estimating CLL in the presence of subsoil chloride to five crops (barley, canola, chickpea, durum and bread wheat). Methods Estimating CLL as a function of chloride comprised two main steps. The first step involved estimating the likely unconstrained CLL as a function of depth, CLLd. The second step adds the effect of chloride onto CLLd. Depth prediction of CLL on unconstrained profiles The unconstrained CLL (CLLd) can be estimated by a linear function (Hochman et al. 2007): _____________________________________________________________________________________________________ © Proceedings of the 20th Agronomy Australia Conference, 2022 Toowoomba Qld www.agronomyaustraliaproceedings.org CLLd = CLL0.4 + fd ∗ d (1) Where CLL0.4 is the CLL measured at the 0.4 m depth, fd is the depth parameter, and d is depth (mm). Here, fd was estimated anew using Vertosol soil profiles selected from the APSoil database, from northern NSW and QLD, with a maximum chloride concentration of 100 mg/kg (resulting in 12 profiles). The Nelder-Mead optimisation routine was then used to derive fd by minimising the sum of the squared differences between CLLd, derived from equation (1) and the field measured CLL. Fractional closure analysis to derive CLL as a function of depth and chloride The fractional closure (FC) is defined as follows: FC = (CLL-CLLd)/(DUL-CLLd) (2) With CLLd as per equation (1). The FC enables evaluation of the level of chloride constraint for a particular profile. For a profile with low chloride, the difference between CLL and CLLd will likely be negligible, and FC will tend to be closer to zero. On the other hand, for a soil profile with high chloride, the CLL is likely to be appreciably greater than CLLd and FC will tend to be closer to 1. Previous work has shown that the FC can be approximated as (Hochman et al. 2007): FC = 1-a*exp(b*cl) (3) Where a and b are parameters and cl is the soil chloride concentration (mg/kg) at the depth of interest. Combining equations (2) and (3) and solving for CLL results in: CLL = CLLd + FC*(DUL-CLLd) (4) CLLcl = CLLd + (1-a*exp(b*cl))*(DUL-CLLd) Where CLLcl is the predicted CLL from depth and chloride. The FC was constrained between 0 and 1 as follows: CLLcl = min[DUL, CLLd + (max[0, (1 a*exp(b*cl))]*(DUL-CLLd)] (5) The a and b parameters in equation (5) were optimised for each crop using data originally presented in Dang et al (2006b). The crops analysed, with the respective number of sites in parenthesis, are as follows: barley (10), canola (7), chickpea (11), durum wheat (7) and bread wheat (16). The NelderMead optimisation routine (implemented in Python 3.8, SciPy module) was used to optimise a and b by minimising the sum of the squared differences between CLLcl and the actual CLL. Results Illustrative example of CLLd and CLLcl, showing FC increasing with chloride and depth (Figure 1 (left)). Regression analysis of observed vs fitted for the depth prediction of CLL on the set of unconstrained soils from the APSoil database resulted in a highly significant R (0.87) with root mean square error (RMSE) of 0.021 (Figure 1 (right)). The optimised parameter, fd, 3.25e-05, was slightly less than the previously reported value of 4.5e-05 (Hochman et al 2007). The FC analysis (Table 1 and Figure 2) resulted in R of 0.86 for barley and ranged from 0.83 to 0.85 for the remaining crops. The RMSE ranged from 0.019 to 0.023. Table 1. FC analysis for five crop species and optimised a and b parameters (equation (5)). Crop N sites N values R RMSE a b Barley 10 40 0.86 0.019 1.47 -0.0017 Canola 7 28 0.83 0.019 1.51 -0.0017 Chickpea 11 44 0.83 0.020 1.67 -0.0025 Durum 7 28 0.85 0.019 1.65 -0.0002 Wheat 16 64 0.84 0.023 1.62 -0.0019 _____________________________________________________________________________________________________ © Proceedings of the 20th Agronomy Australia Conference, 2022 Toowoomba Qld www.agronomyaustraliaproceedings.org For all crops, at chloride concentration < ~200 mg/kg, FC was approximately zero, i.e., CLL was unaffected by chloride (Figure 2). Above ~250 mg/kg chloride, CLL increased above CLLd, as indicated by the increasing FC. This threshold matches earlier indications of there being a threshold (Hochman et al. 2007). The concentration required for halving the FC was ~500 mg/kg for chickpea and ~600 mg/kg for the other crops; appreciably greater than the concentration determined previously, 416 mg/kg, for halving the water extraction coefficient in APSIM (Hochman et al. 2007). The data confirms that CLL for chickpea tapers faster towards the DUL than that of the other crops as chloride increases, reflecting its higher sensitivity to salinity. The increased sensitivity of durum relative to wheat, reported previously (Dang et al 2006b, Dang et al 2008), was not reflected (Figure 2) and requires further investigation. Figure 1. Illustration of CLLd (unconstrained), predicted using equation (1), and CLLcl predicted from equation (5) using the shown chloride concentration (left). Regression analysis of observed CLL and depth predicted CLL, CLLd, (eq1) using unconstrained (i.e. chloride less than 100 mg/kg) Vertosols from the APSoil database (ref) (listed in the figure legend) (right). Figure 2. Optimised FC function plotted (see parameters in Table 1) with the actual FC for each crop, shown (a) to (e). Comparison of FC functions for each crop shown in (f). _____________________________________________________________________________________________________ © Proceedings of the 20th Agronomy Australia Conference, 2022 Toowoomba Qld www.agronomyaustraliaproceedings.org A potential limitation of this technique is that estimating the unconstrained CLL, using eq1, assumes that the 0.4 m depth is itself unconstrained. If CLL at the 0.4 m depth is adversely impacted by subsoil salinity or any other subsoil constraint, the analysis will tend to overestimate FC. Previous analysis (Hochman et al. 2001) in developing CLL predictions for different crops, based on measured DUL and soil suborder (grey and black Vertosols), resulted in greater differences between crops; a result, aside from chickpea, that was not overly apparent herein and requires further investigation. Conclusion The chloride-constrained CLL was successfully modelled for five crop species, enabling estimation of crop-specific PAWC in the presence of varying levels of subsoil chloride. Chickpea was the most sensitive crop to subsoil chloride, while there was no discernible difference between the other crops (barley, canola, durum wheat and bread wheat). References Burk L, Dalgliesh N (2013)
MoreTranslated text
AI Read Science
Must-Reading Tree
Example
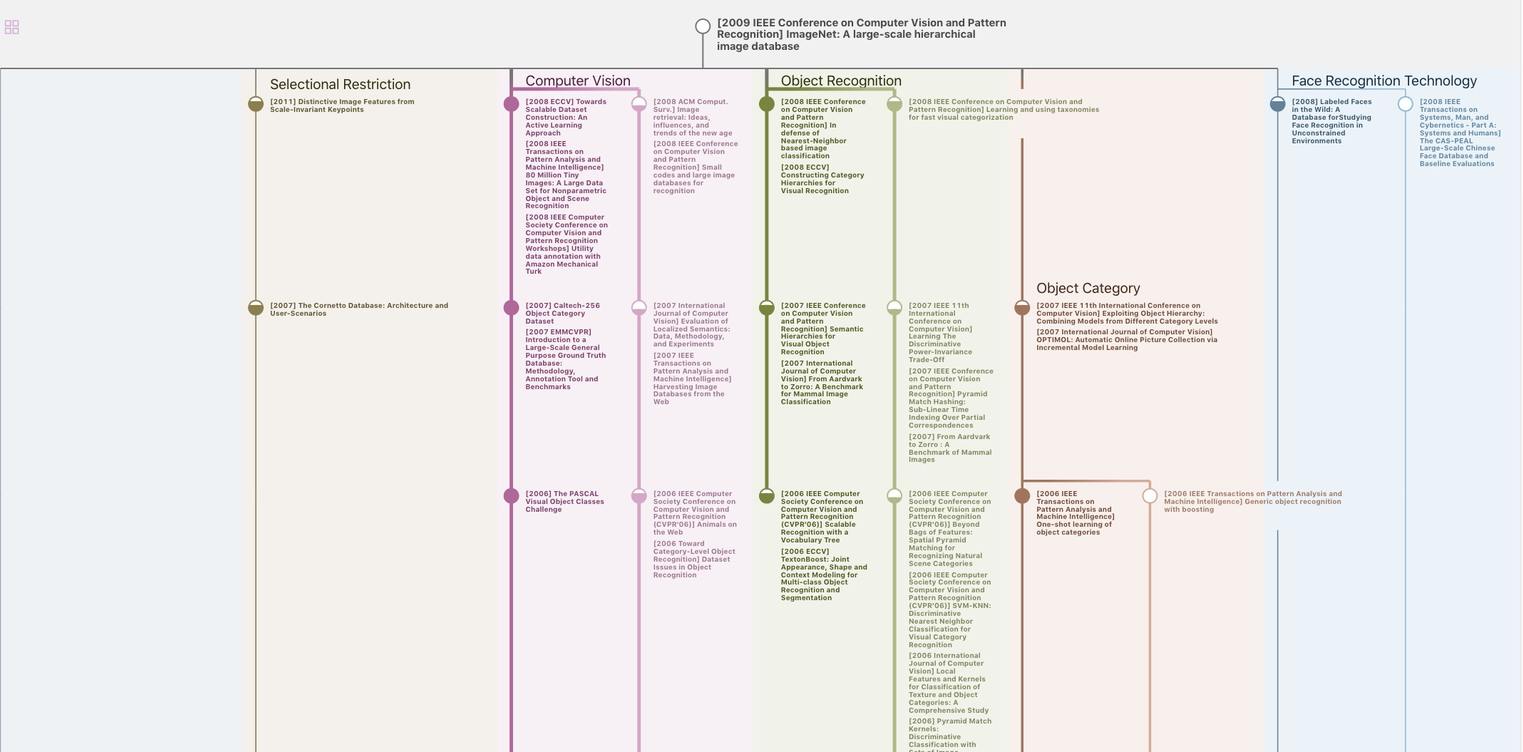
Generate MRT to find the research sequence of this paper
Chat Paper
Summary is being generated by the instructions you defined