ADeADA: Adaptive Density-aware Active Domain Adaptation for Semantic Segmentation
ArXiv(2022)
摘要
In the field of domain adaptation, a trade-off exists between the model performance and the number of target domain annotations. Active learning, maximizing model performance with few informative labeled data, comes in handy for such a scenario. In this work, we present ADeADA, a general active domain adaptation framework for semantic segmentation. To adapt the model to the target domain with minimum queried labels, we propose acquiring labels of the samples with high probability density in the target domain yet with low probability density in the source domain, complementary to the existing source domain labeled data. To further facilitate the label efficiency, we design an adaptive budget allocation policy, which dynamically balances the labeling budgets among different categories as well as between density-aware and uncertainty-based methods. Extensive experiments show that our method outperforms existing active learning and domain adaptation baselines on two benchmarks, GTA5 → Cityscapes and SYNTHIA → Cityscapes. With less than 5% target domain annotations, our method reaches comparable results with that of full supervision.
更多查看译文
AI 理解论文
溯源树
样例
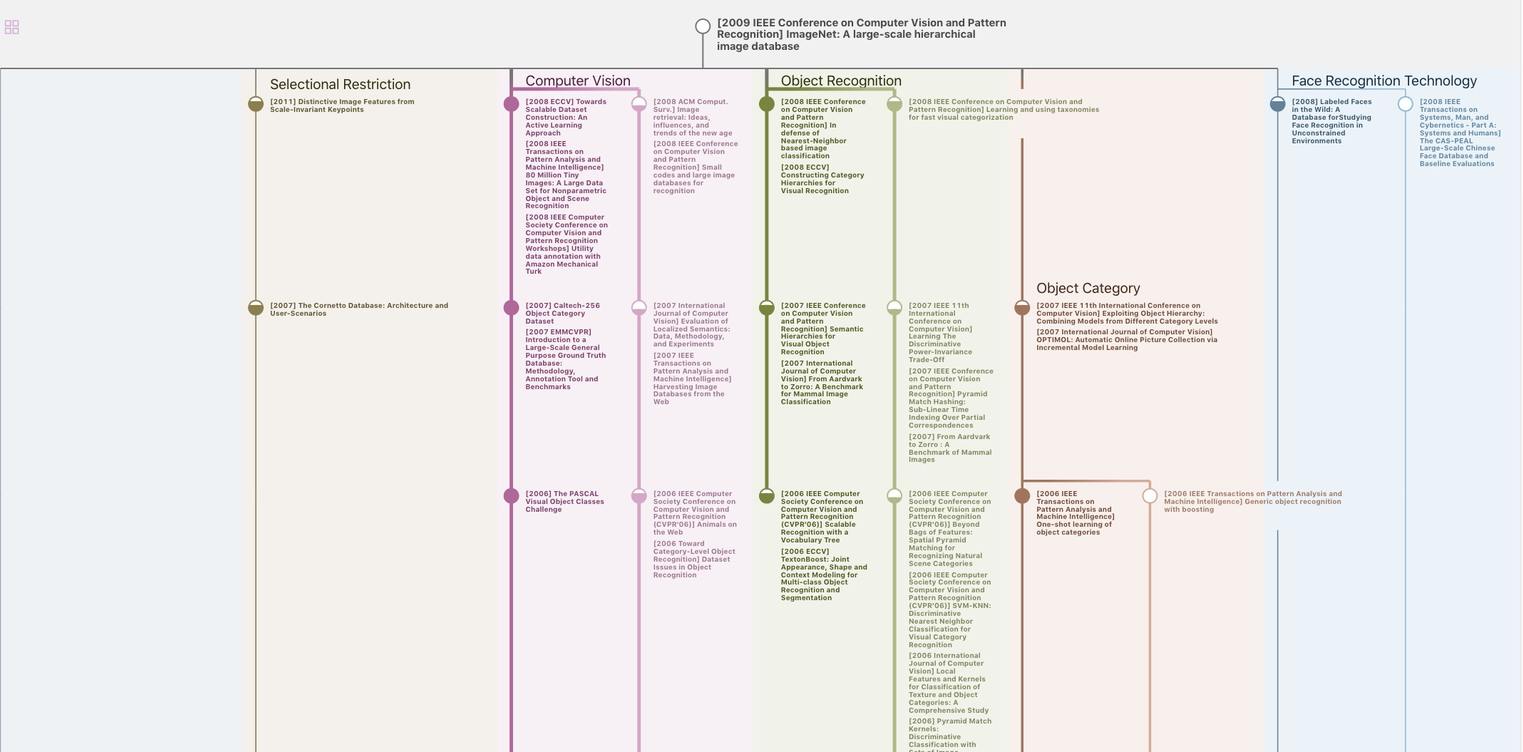
生成溯源树,研究论文发展脉络
Chat Paper
正在生成论文摘要