Accounting for longitudinal data structures when disseminating synthetic data to the public
semanticscholar(2021)
摘要
In this talk we evaluate if the concept of differential privacy can be used to disseminate detailed geocoding information without compromising the confidentiality of the individuals included in the database. To enable the release of detailed geographical information we propose a differentially private procedure based on a microaggregation algorithm with a fixed minimal cluster size. We evaluate whether meaningful results can be obtained with this approach using administrative data gathered by the German Federal Employment Agency. Detailed geocoding information has been added to this database recently and plans call for making this valuable source of information available to the scientific community. We generate differentially private microdata using different levels of geographical detail to identify the most detailed level that still provides acceptable analytical validity while offering strong differential privacy guarantees. Accounting for longitudinal data structures when disseminating synthetic data to the public Sana Rashid∗, Jörg Drechsler∗∗, Robin Mitra∗∗∗ ∗ University of Southampton Highfield Campus, Mathematics Building 54, Southampton SO17 1BJ, sanarashidmahmood@gmail.com ∗∗ Institute for Employment Research and University of Maryland, Regensburger Str. 104, 90478 Nuremberg, Germany, joerg.drechsler@iab.de ∗∗∗ School of Mathematics, Cardiff University, Cardiff, CF24 4AG, mitrar5@cardiff.ac.uk Abstract. When generating synthetic data for public release, careful attention must be given to the selection of appropriate synthesis models. If the dataset has a longitudinal structure it is not obvious which synthesis model should be used to account for the design. Using multiple imputation for missing data, it has been shown previously that employing fixed effects at the imputation stage may adversely affect inferences obtained by an analyst wishing to use random effects to account for the clustering of observations within units and vice versa. Since it is generally unknown which model users of the data will prefer, a synthesis model should be preferred that suits both analysis models. We evaluate several strategies for generating longitudinal synthetic datasets using extensive simulation studies. In our evaluations, we consider both, the analytical validity and the risk of disclosure resulting from the different synthesis strategies. We find that synthesis models should be preferred that cannot be classified as pure random or fixed effects models. We illustrate our findings using data from the German IAB Establishment Panel. When generating synthetic data for public release, careful attention must be given to the selection of appropriate synthesis models. If the dataset has a longitudinal structure it is not obvious which synthesis model should be used to account for the design. Using multiple imputation for missing data, it has been shown previously that employing fixed effects at the imputation stage may adversely affect inferences obtained by an analyst wishing to use random effects to account for the clustering of observations within units and vice versa. Since it is generally unknown which model users of the data will prefer, a synthesis model should be preferred that suits both analysis models. We evaluate several strategies for generating longitudinal synthetic datasets using extensive simulation studies. In our evaluations, we consider both, the analytical validity and the risk of disclosure resulting from the different synthesis strategies. We find that synthesis models should be preferred that cannot be classified as pure random or fixed effects models. We illustrate our findings using data from the German IAB Establishment Panel.
更多查看译文
AI 理解论文
溯源树
样例
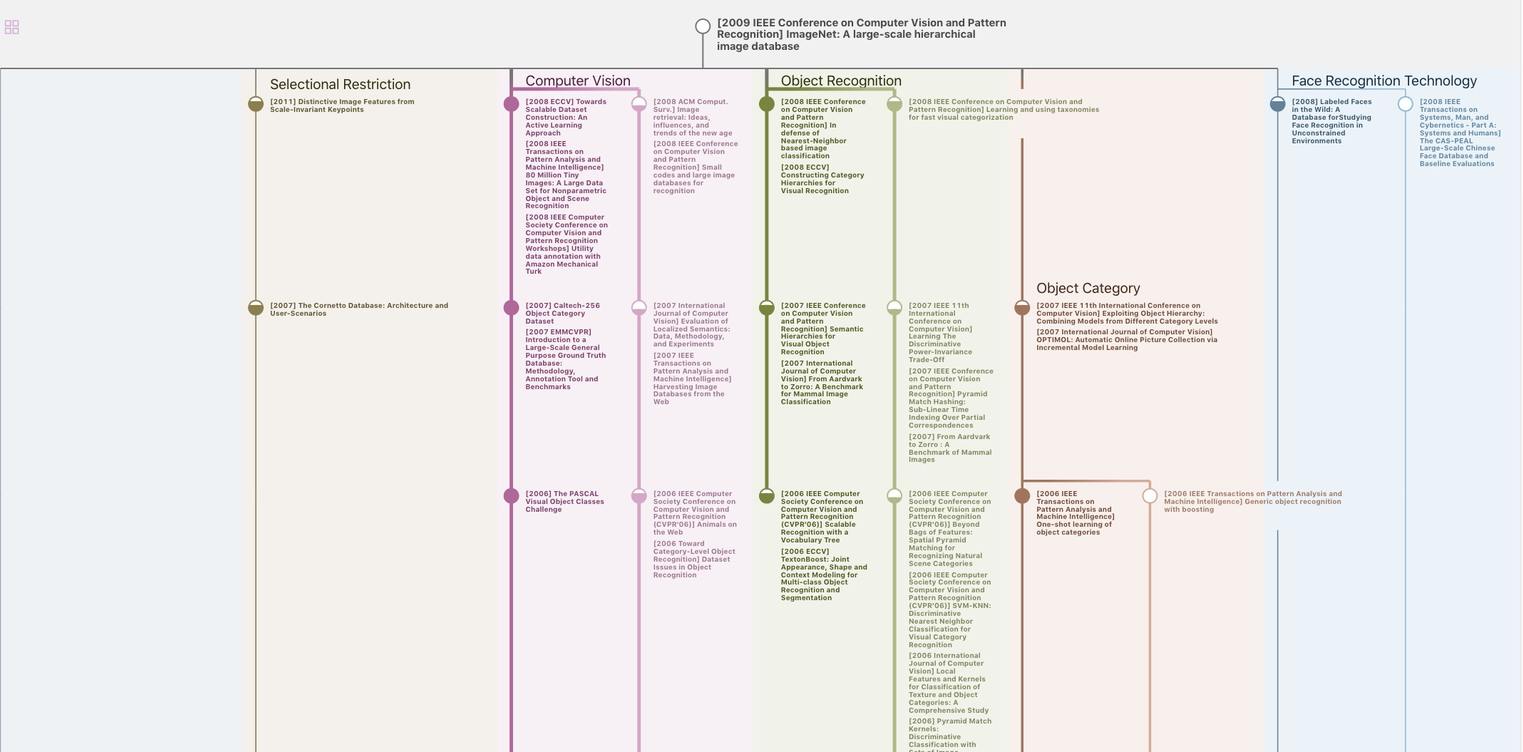
生成溯源树,研究论文发展脉络
Chat Paper
正在生成论文摘要