Multiclassification with Random Forest Algorithm for Highly Imbalanced Particle Data Structure
semanticscholar(2022)
摘要
Data used in particle physics analyses have an imbalanced nature in which the events of interest are rare due to the broad background. Classification algorithms provided by supervised machine learning (ML) approaches can be utilized to interpret skewed dataset. In this study, the ground state of the bottomonium (Υ (1S)) and its excited states (Υ (2S), Υ (3S) and Υ (4S)) were determined by application of multiclassification approach based on Random Forest Classifier (RFC). Model learning from highly imbalanced data can be improved by implementation of resampling techniques for preprocessing dataset and modification of the weighting strategy. For this purpose, five widely used oversampling and two hybrid strategies, using over and under resampling together, were adjusted to RFC. Moreover, class weights applied RFC, Weighted Random Forest (WRF), was used in the analysis. Due to the data structure, performance of the applied models was evaluated by the derivatives of confusion matrix. It is revealed that hybrid techniques implemented in RFC is suitable for handling highly imbalanced classes. G-mean and BAcc scores of upsilon states presented that with SMOTEENN strategy the model exhibited highest classification achievement, more than 90%, with high sensitivity implying the success of the application on multiclassification.
更多查看译文
AI 理解论文
溯源树
样例
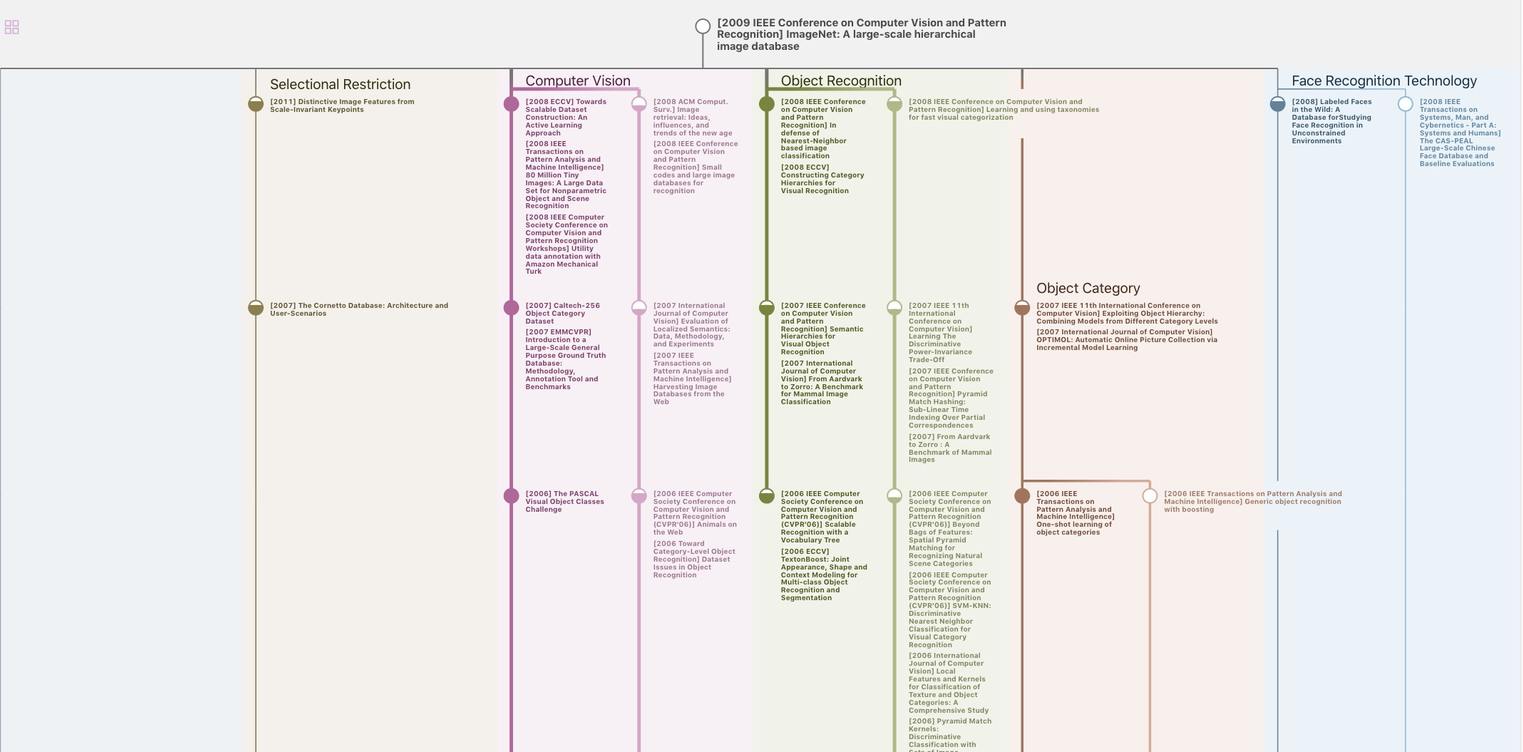
生成溯源树,研究论文发展脉络
Chat Paper
正在生成论文摘要