Fault Diagnosis for Limited Annotation Signals and Strong Noise Based on Interpretable Attention Mechanism
IEEE Sensors Journal(2022)
摘要
Deep learning methods based on vibration signals of rotating machinery have been continuously developed in fault diagnosis. However, there are still three challenges in intelligent fault diagnosis: (1) Limited annotation data; (2) Interference of strong noise; (3) Continuous changes of signals due to working conditions. To solve the problems above, a method based on dual-path convolution with attention mechanism and capsule network (WDACN) is established for efficient diagnosis, where the more dominant informative segments of vibration signal are focused by a novel attention mechanism, namely, Multi-branch Parallelized Attention Mechanism (MBPAM). Besides, an improved visualization method—Gradient Score Class Activation Mapping (GS-CAM) is proposed to analyze the attention distribution on time domain signals from the perspective of interpretability. Experiments are conducted on the data of bearings and gearbox, which prove that WDACN has excellent capacities of generalization and robustness.
更多查看译文
关键词
Convolutional neural network,attention mechanism,limited data,interpretability,capsule network,fault diagnosis
AI 理解论文
溯源树
样例
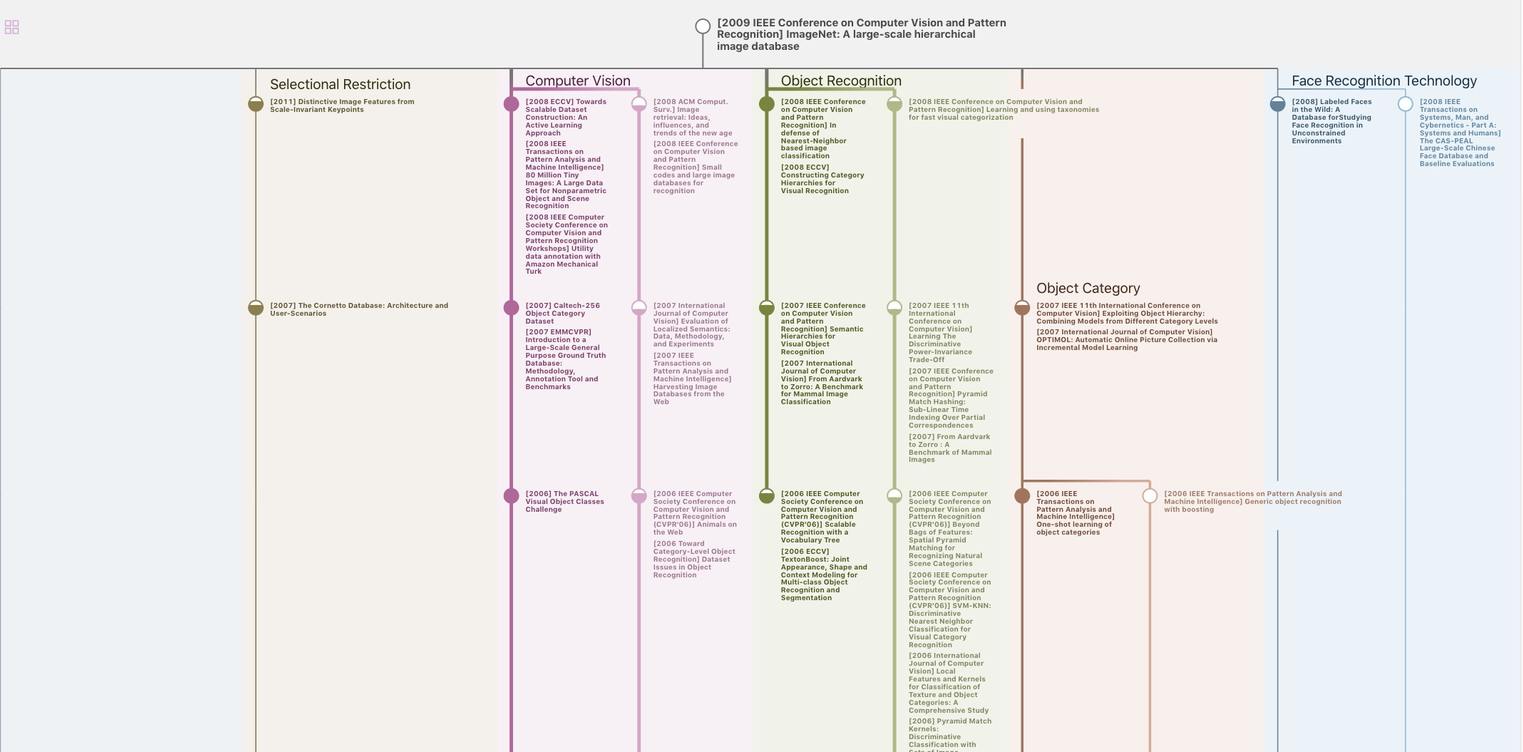
生成溯源树,研究论文发展脉络
Chat Paper
正在生成论文摘要