Comparative study of ensemble models of deep convolutional neural networks for crop pests classification
MULTIMEDIA TOOLS AND APPLICATIONS(2023)
摘要
Pest infestations on wheat, corn, soybean, and other crops can cause substantial losses to their yield. Classification of crop pests is of considerable importance for accurate and intelligent pest control. Ensemble models can effectively improve the accuracy of crop pests classification and different ensemble models can produce different results. To study the advantages and disadvantages of ensemble models under different agricultural production environments, six basic models are trained on a D0 dataset. Then, three models with the best classification performance are selected. Finally, the ensemble models, i.e., linear ensemble named SAEnsemble and nonlinear ensemble SBPEnsemble, are designed to combine the basic models for crop pests classification. The accuracies of SAEnsemble and SBPEnsemble improved by 0.85% and 1.49% respectively compared to the basic model with the highest accuracy. Comparison of the two proposed ensemble models shows that the accuracy of SAEnsemble is lower when the pests are very similar to the background, while SBPEnsemble is more likely to make wrong predictions under the condition of huge difference between pests and background. Therefore, in practical agricultural production, choosing linear or nonlinear ensemble methods according to different situations can effectively improve the accuracy of crop pests classification.
更多查看译文
关键词
Crop pest classification,Linear and nonlinear,Ensemble model
AI 理解论文
溯源树
样例
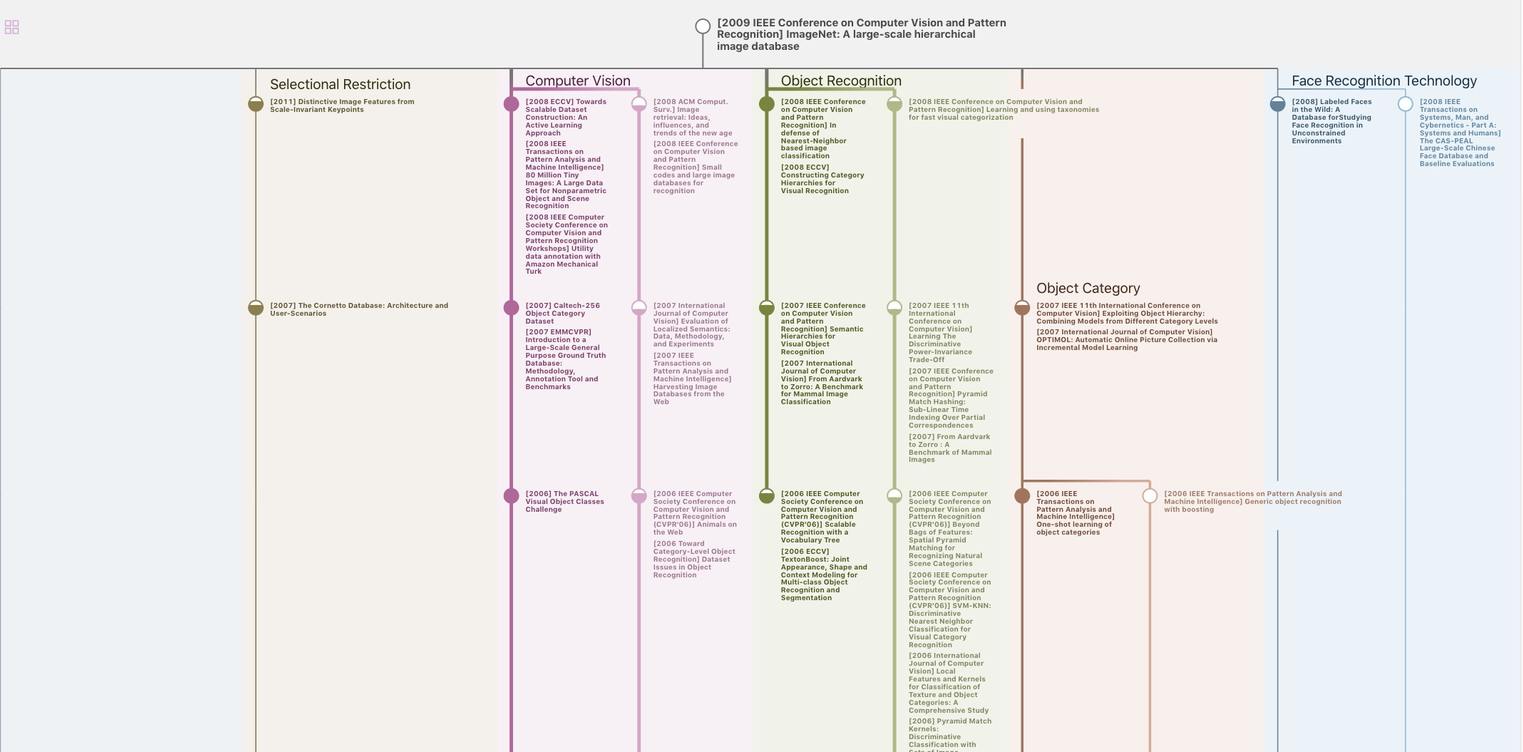
生成溯源树,研究论文发展脉络
Chat Paper
正在生成论文摘要