Understanding the Design-Space of Sparse/Dense Multiphase GNN dataflows on Spatial Accelerators
IEEE International Parallel and Distributed Processing Symposium (IPDPS)(2022)
摘要
Graph Neural Networks (GNNs) have garnered a lot of recent interest because of their success in learning representations from graph-structured data across several critical applications in cloud and HPC. Owing to their unique compute and memory characteristics that come from an interplay between dense and sparse phases of computations, the emergence of recon-figurable dataflow (aka spatial) accelerators offers promise for acceleration by mapping optimized dataflows (i.e., computation order and parallelism) for both phases. The goal of this work is to characterize and understand the design-space of dataflow choices for running GNNs on spatial accelerators in order for mappers or design-space exploration tools to optimize the dataflow based on the workload. Specifically, we propose a taxonomy to describe all possible choices for mapping the dense and sparse phases of GNN inference, spatially and temporally over a spatial accelerator, capturing both the intra-phase dataflow and the inter-phase (pipelined) dataflow. Using this taxonomy, we do deep-dives into the cost and benefits of several dataflows and perform case studies on implications of hardware parameters for dataflows and value of flexibility to support pipelined execution.
更多查看译文
关键词
Graph Neural Networks,Spatial Accelerators,Dataflows,Pipelined Parallelism
AI 理解论文
溯源树
样例
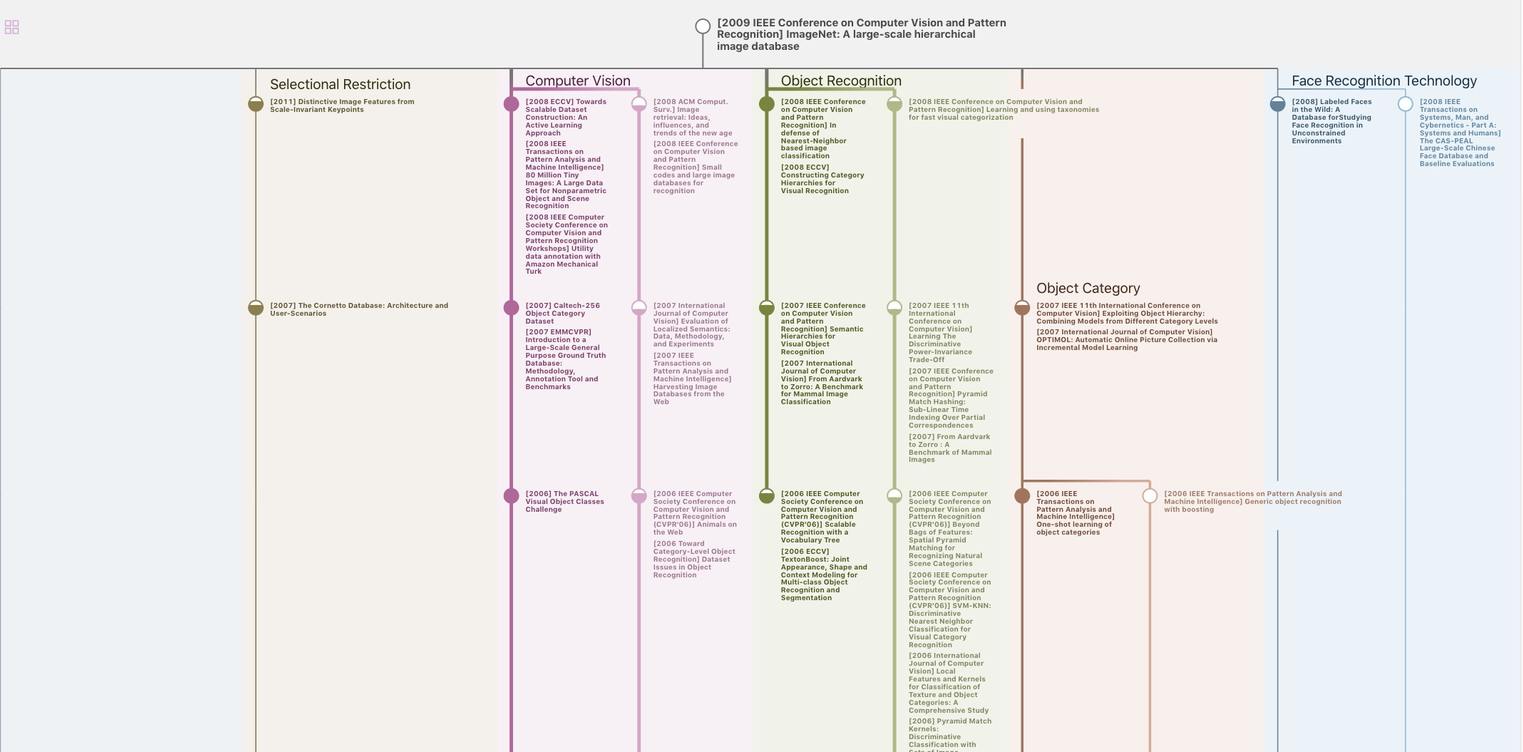
生成溯源树,研究论文发展脉络
Chat Paper
正在生成论文摘要