Research on Identification of Corn Disease Occurrence Degree Based on Improved ResNeXt Network
INTERNATIONAL JOURNAL OF PATTERN RECOGNITION AND ARTIFICIAL INTELLIGENCE(2022)
摘要
Aggregate depth residual network (ResNeXt) can not only improve the accuracy without increasing the parameter complexity, but also reduce the number of super parameters. It is one of the popular convolutional neural network models for image recognition. Maize diseases have a great impact on maize yield, quality and farmers' income. Rapid and effective identification of the severity of maize diseases plays an important role in accurate control and accurate drug use. The general ResNeXt model has large spots in extracting image features, but the spots of corn diseases are small and the extracted features are not obvious, which affects the recognition accuracy. Therefore, an improved ResNeXt model is proposed to recognize the occurrence degree of corn diseases. First, the original data of maize disease degree are classified according to national standards. Second, the original data are extended through data enhancement. Third, the original ResNeXt101 model is improved. The first layer convolution kernel is changed to three 3 * 3 convolution kernels, and the cardinality is adjusted to 64. Finally, the improved model is verified. The recognition accuracy of corn disease degree is 89.667%, which is 0.98% higher than the original model. Through testing on 276 actually collected corn disease images, the recognition accuracy is 90.22%. Therefore, this method is feasible for the diagnosis of corn disease degree and can provide an important basis for accurate prevention and control.
更多查看译文
关键词
Corn,degree of disease,improved ResNeXt,data enhancement,image recognition
AI 理解论文
溯源树
样例
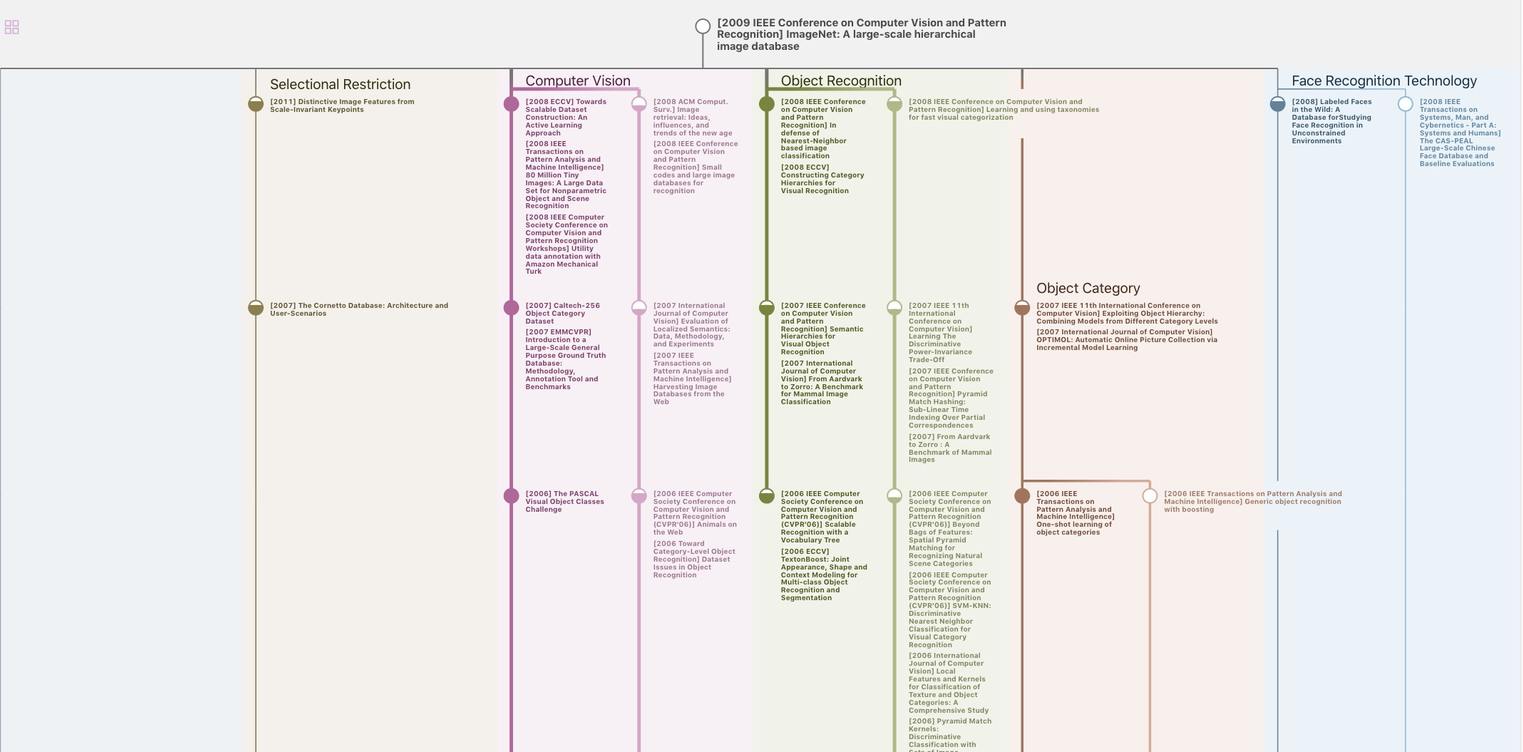
生成溯源树,研究论文发展脉络
Chat Paper
正在生成论文摘要