Approximate Exploitability: Learning a Best Response
European Conference on Artificial Intelligence(2021)
摘要
A standard metric used to measure the approximate optimality of policies in imperfect information games is exploitability, i.e. the performance of a policy against its worst-case opponent. However, exploitability is intractable to compute in large games as it requires a full traversal of the game tree to calculate a best response to the given policy. We introduce a new metric, approximate exploitability, that calculates an analogous metric using an approximate best response; the approximation is done by using search and reinforcement learning. This is a generalization of local best response, a domain specific evaluation metric used in poker. We provide empirical results for a specific instance of the method, demonstrating that our method converges to exploitability in the tabular and function approximation settings for small games. In large games, our method learns to exploit both strong and weak agents, learning to exploit an AlphaZero agent. 1
更多查看译文
关键词
Machine Learning: Reinforcement Learning,Agent-based and Multi-agent Systems: Multi-agent Learning,Agent-based and Multi-agent Systems: Noncooperative Games
AI 理解论文
溯源树
样例
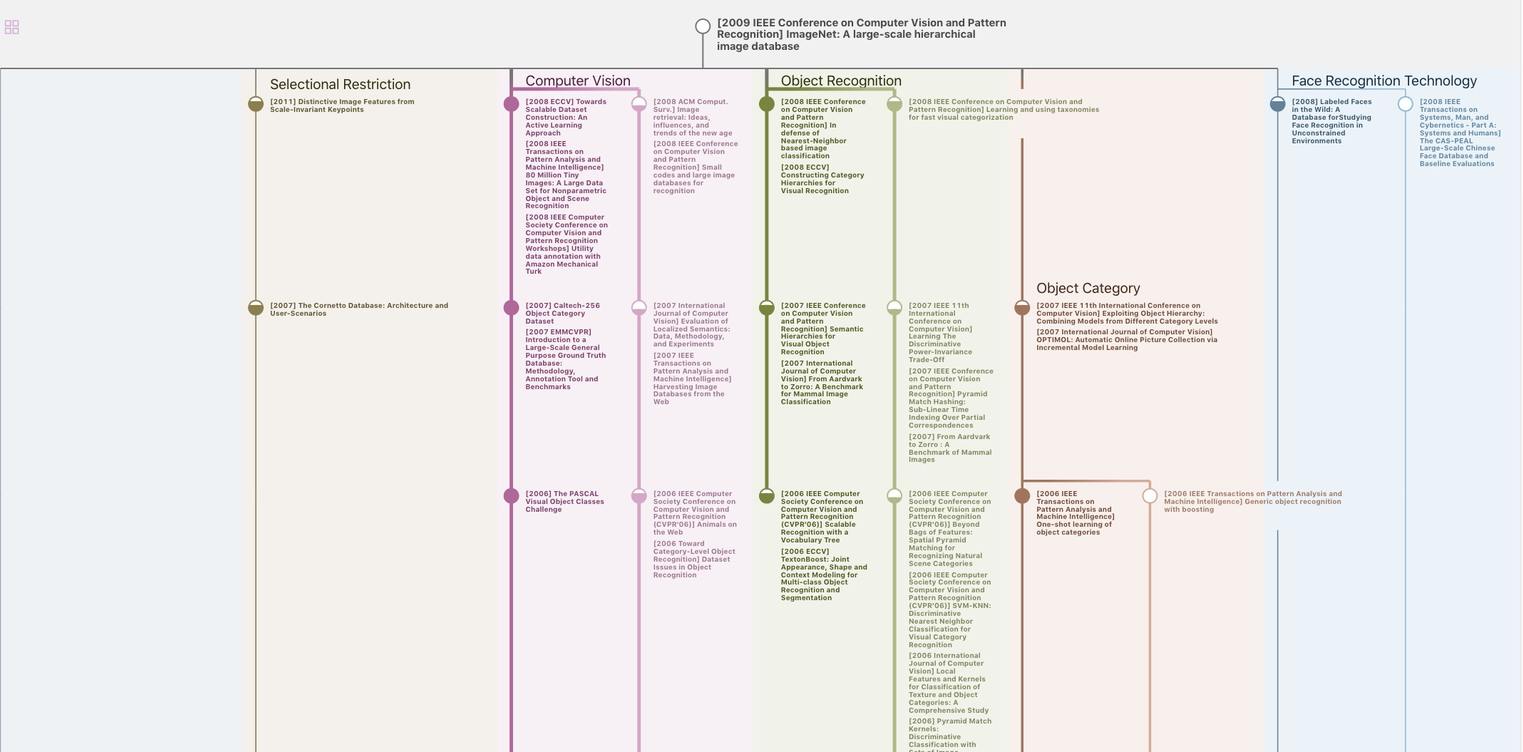
生成溯源树,研究论文发展脉络
Chat Paper
正在生成论文摘要