Multi-context unsupervised domain adaption for HEp-2 cell classification using maximum partial classifier discrepancy
The Journal of Supercomputing(2022)
摘要
Identifying human epithelial-2 (HEp-2) cells in indirect immune fluorescence (IIF) is the most commonly used method for the diagnosis of autoimmune diseases. Although supervised deep learning networks have made remarkable progress on HEp-2 cell staining pattern classification, the high-performance relies on a large amount of labeled training data. Unfortunately, large-scale labeled datasets are scarce due to the expensive costs of labeling medical images. Therefore, we propose an unsupervised domain adaption (UDA) model, namely MDA-MPCD, to classify unlabeled HEp-2 cell samples. The proposed model involves two major aspects: (a) multi-context domain adaption (MDA) generator and (b) maximum partial classifier discrepancy (MPCD) architecture. The MDA generator can extract multi-context features from complex cell images while providing more comprehensive and diverse information for the classifier. The MPCD architecture, utilizing the mapping variation of feature transfer, focuses on the discrepancy from the cross-domain gap by separating the activations in the classifier. The proposed model dominates all comparison methods during evaluation, achieving 85.35% and 96.08% mean accuracy on two UDA tasks, respectively. Furthermore, the model is demonstrated to adapt from rich label domain to unlabeled domain by detailed ablation experiments and visualization results. The proposed MDA-MPCD has potential as a clinical scheme, enabling effective and accurate classification of HEp-2 cell staining pattern without labeling the target data.
更多查看译文
关键词
Unsupervised domain adaption,HEp-2 cell classification,Multi-context,Maximum classifier discrepancy
AI 理解论文
溯源树
样例
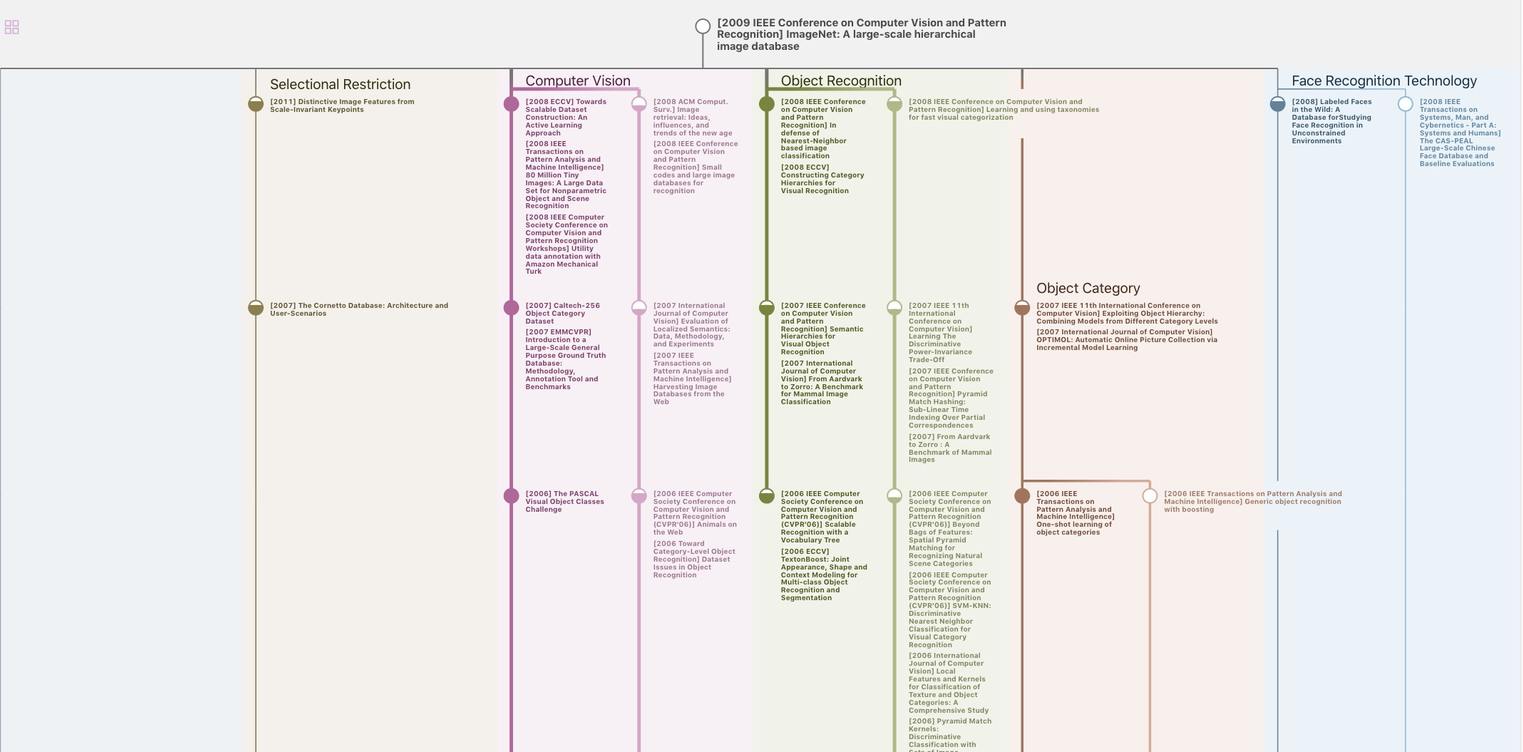
生成溯源树,研究论文发展脉络
Chat Paper
正在生成论文摘要