Partial Knowledge Transfer in Visual Recognition Systems via Joint Loss-Aware Consistency Learning
IEEE Transactions on Industrial Informatics(2022)
摘要
One of the key challenges for the implementation of visual recognition systems in the real world is to construct prediction models that can realize the knowledge transfer from the seen data to the unseen data. Specifically, partial knowledge transfer (PKT) aims to address a more common and realistic scenario in which we are accessible to a label-rich source domain while working on a relative label-scarce target domain. The essence of PKT is to explore the latent categories across different domains and simultaneously facilitate the positive transfer from these data. In this article, we propose a joint loss-aware consistency learning (JLACL) to effectively enhance the transferability of knowledge in visual recognition systems, which conducts an iterative optimization on three-level losses, including discrepancy loss, consensus loss, and cross-entropy loss. The discrepancy loss is designed to eliminate the class distribution bias by a similarity perception metric between the source and target domains. The consensus loss can assist to preserve domain-invariant and representative features for model learning by exploring correlation. Moreover, we also find that using the cross-entropy loss to determine the shared label space, which can help to alleviate the negative transfer by suppressing the features with nonshared labels. Finally, the PKT can be successfully achieved by joint optimization of total losses. Extensive experiments on several public and challenging datasets in visual recognition applications adequately demonstrate the superiority of our proposed JLACL over existing state-of-the-art PKT methods.
更多查看译文
关键词
Consistency learning,domain discrepancy,loss-aware optimization,partial knowledge transfer (PKT),visual recognition systems (VRSs)
AI 理解论文
溯源树
样例
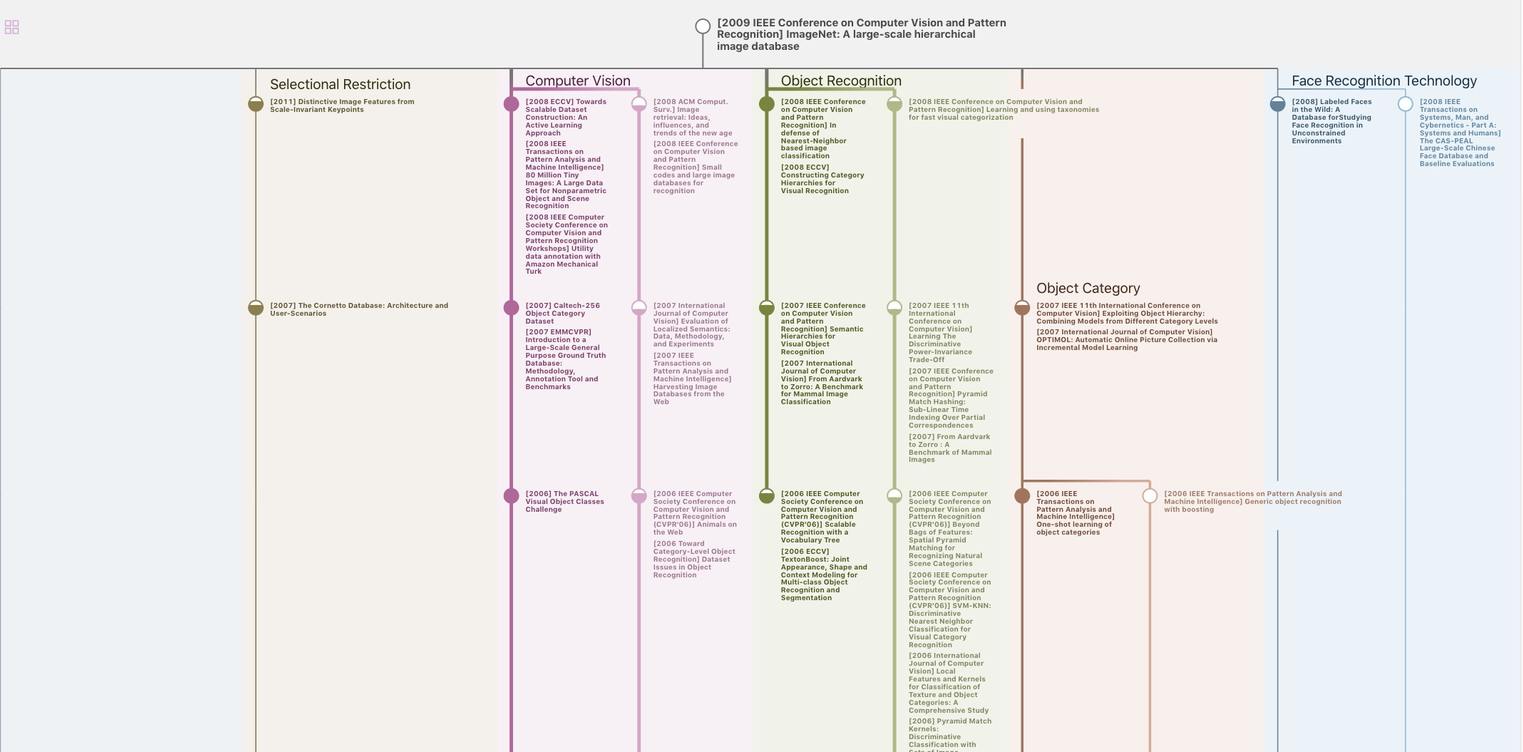
生成溯源树,研究论文发展脉络
Chat Paper
正在生成论文摘要