A Spatial AMH Copula-Based Dissimilarity Measure to Cluster Variables in Panel Data
semanticscholar(2021)
摘要
Investigating thermal energy demand is crucial for the development of sustainable cities and efficient use of renewable sources. Despite the advances made in this field, the analysis of energy data provided by smart grids is currently a demanding challenge. In this paper, we develop a clustering methodology based on a novel dissimilarity measure to analyze a high temporal resolution panel data for district heating demand in the Italian city Bozen-Bolzano. Starting from the characteristics of this data, we explore the usefulness of the Ali-Mikhail-Haq copula in defining a new dissimilarity measure to cluster variables in a hierarchical framework. We show that our proposal is particularly sensitive to small dissimilarities based on tiny differences in the dependence level. Therefore, the proposed measure is able to better distinguish between objects with low dissimilarity than classic rank-based dissimilarity measures. Moreover, our proposal is defined in a spatial version that is able to take into account the spatial location of the compared objects. We investigate the proposed measure through Monte Carlo studies and compare it with the corresponding spatial Kendall's correlation-based dissimilarity measure. Finally, the application to real data makes it possible to find clusters of buildings homogeneous with respect to their main characteristics, such as energy efficiency and heating surface, to support the design, expansion and management of district heating systems.
更多查看译文
关键词
cluster variables,dissimilarity measure,panel,copula-based
AI 理解论文
溯源树
样例
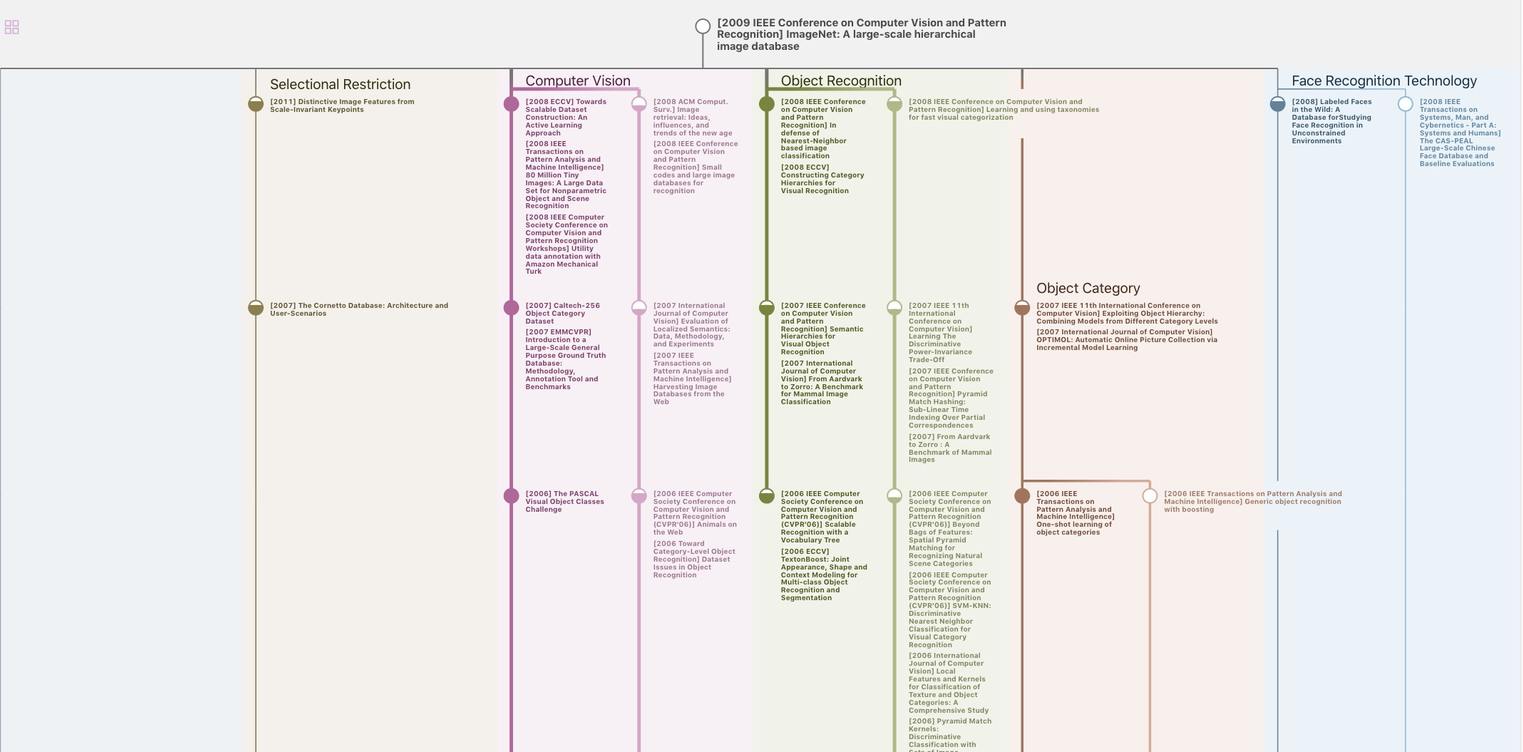
生成溯源树,研究论文发展脉络
Chat Paper
正在生成论文摘要