InDiD: Instant Disorder Detection Via a Principled Neural Network
PROCEEDINGS OF THE 30TH ACM INTERNATIONAL CONFERENCE ON MULTIMEDIA, MM 2022(2022)
Skolkovo Inst Sci & Technol Skoltech
Abstract
For sequential data, a change point is a moment of abrupt regime switch in data streams. Such changes appear in different scenarios, including simpler data from sensors and more challenging video surveillance data. We need to detect disorders as fast as possible. Classic approaches for change point detection (CPD) might underperform for semi-structured sequential data because they cannot process its structure without a proper representation. We propose a principled loss function that balances change detection delay and time to a false alarm. It approximates classic rigorous solutions but is differentiable and allows representation learning for deep models. We consider synthetic sequences, real-world data sensors and videos with change points. We carefully labelled available video data with change point moments and released it for the first time. Experiments suggest that complex data require meaningful representations tailored for the specificity of the CPD task --- and our approach provides them outperforming considered baselines. For example, for explosion detection in video, the F1 score for our method is 0.53 compared to baseline scores of 0.31 and 0.35.
MoreTranslated text
Key words
change point detection,representation learning,video analysis and understanding
PDF
View via Publisher
AI Read Science
AI Summary
AI Summary is the key point extracted automatically understanding the full text of the paper, including the background, methods, results, conclusions, icons and other key content, so that you can get the outline of the paper at a glance.
Example
Background
Key content
Introduction
Methods
Results
Related work
Fund
Key content
- Pretraining has recently greatly promoted the development of natural language processing (NLP)
- We show that M6 outperforms the baselines in multimodal downstream tasks, and the large M6 with 10 parameters can reach a better performance
- We propose a method called M6 that is able to process information of multiple modalities and perform both single-modal and cross-modal understanding and generation
- The model is scaled to large model with 10 billion parameters with sophisticated deployment, and the 10 -parameter M6-large is the largest pretrained model in Chinese
- Experimental results show that our proposed M6 outperforms the baseline in a number of downstream tasks concerning both single modality and multiple modalities We will continue the pretraining of extremely large models by increasing data to explore the limit of its performance
Try using models to generate summary,it takes about 60s
Must-Reading Tree
Example
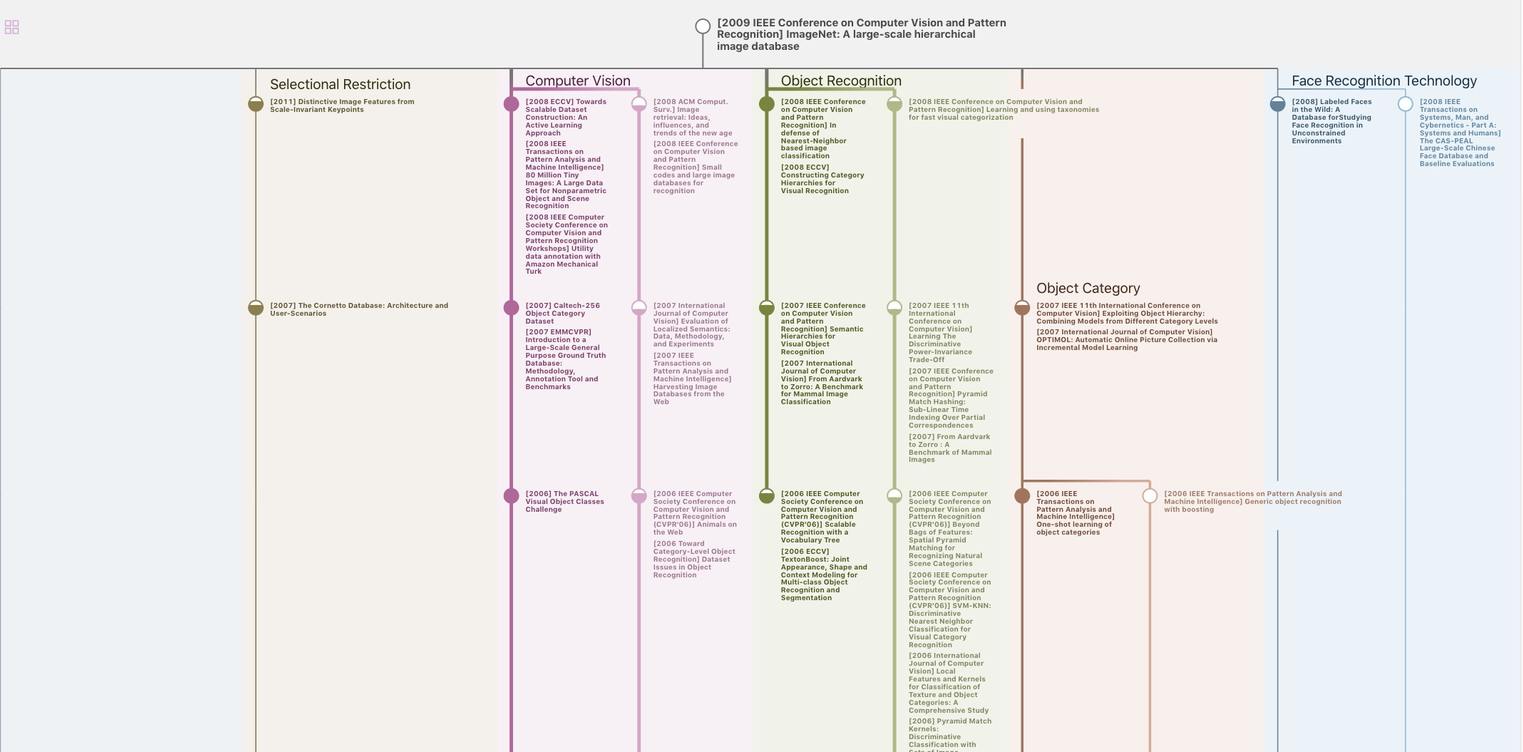
Generate MRT to find the research sequence of this paper
Related Papers
2018
被引用469 | 浏览
2019
被引用14 | 浏览
2014
被引用34327 | 浏览
Data Disclaimer
The page data are from open Internet sources, cooperative publishers and automatic analysis results through AI technology. We do not make any commitments and guarantees for the validity, accuracy, correctness, reliability, completeness and timeliness of the page data. If you have any questions, please contact us by email: report@aminer.cn
Chat Paper
GPU is busy, summary generation fails
Rerequest