Gaussian Processes for Autonomous Data Acquisition at Large-Scale Synchrotron and Neutron Scattering Facilities
semanticscholar(2021)
摘要
The execution and analysis of ever more complex experiments are increasingly challenged by the vast dimensionality of the parameter spaces that underlie investigations in the biological, chemical, physical, and materials sciences. While an increase in data-acquisition rates should allow broader querying of the parameter space, the complexity of experiments and the subtle dependence of the model function on input parameters remains daunting due to the sheer number of variables. To meet these challenges, new strategies for autonomous data acquisition are rapidly coming to fruition, and are being deployed across a spectrum of scientific experiments. One promising direction that is being explored is the use of Gaussian process regression (GPR). GPR is a quick, non-parametric, and robust approximation and uncertainty quantification method that can directly be applied to autonomous data acquisition. In this work, we review and reformulate our most recent contributions to GPR-driven autonomous experimentation in more general terms, and illustrate the functionality of the techniques we present, using new, real-world examples from large experimental facilities in the United States (ALS and NSLS II) and France (ILL). We start by introducing the basics of a GPR-driven autonomous loop with a focus on Gaussian processes. We then shift the focus to the infrastructure that has to be built around GPR to create a closed-loop. Finally, our examples show that Gaussian-process-based autonomous data acquisition is a widely applicable method that can facilitate the optimal utilization of instruments and facilities by enabling the efficient acquisition of high-value datasets.
更多查看译文
AI 理解论文
溯源树
样例
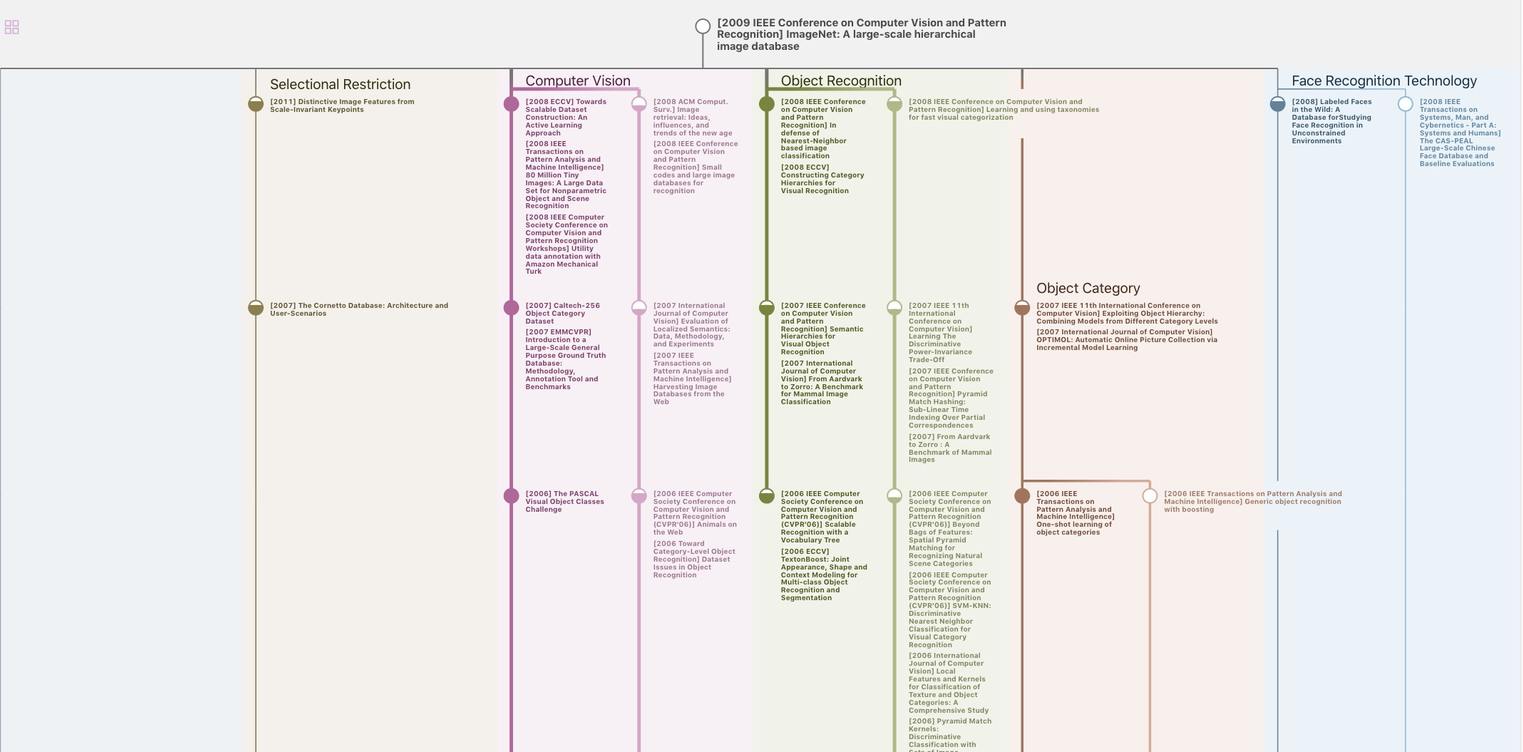
生成溯源树,研究论文发展脉络
Chat Paper
正在生成论文摘要