Network classification-based structural analysis of real networks and their model-generated counterparts
NETWORK SCIENCE(2022)
摘要
Data-driven analysis of complex networks has been in the focus of research for decades. An important area of research is to study how well real networks can be described with a small selection of metrics, furthermore how well network models can capture the relations between graph metrics observed in real networks. In this paper, we apply machine-learning techniques to investigate the aforementioned problems. We study 500 real-world networks along with 2000 synthetic networks generated by four frequently used network models with previously calibrated parameters to make the generated graphs as similar to the real networks as possible. This paper unifies several branches of data-driven complex network analysis, such as the study of graph metrics and their pair-wise relationships, network similarity estimation, model calibration, and graph classification. We find that the correlation profiles of the structural measures significantly differ across network domains and the domain can be efficiently determined using a small selection of graph metrics. The structural properties of the network models with fixed parameters are robust enough to perform parameter calibration. The goodness-of-fit of the network models highly depends on the network domain. By solving classification problems, we find that the models lack the capability of generating a graph with a high clustering coefficient and relatively large diameter simultaneously. On the other hand, models are able to capture exactly the degree-distribution-related metrics.
更多查看译文
关键词
complex networks, network models, model calibration, model stability, domain prediction, network characteristics, network classification, network similarity
AI 理解论文
溯源树
样例
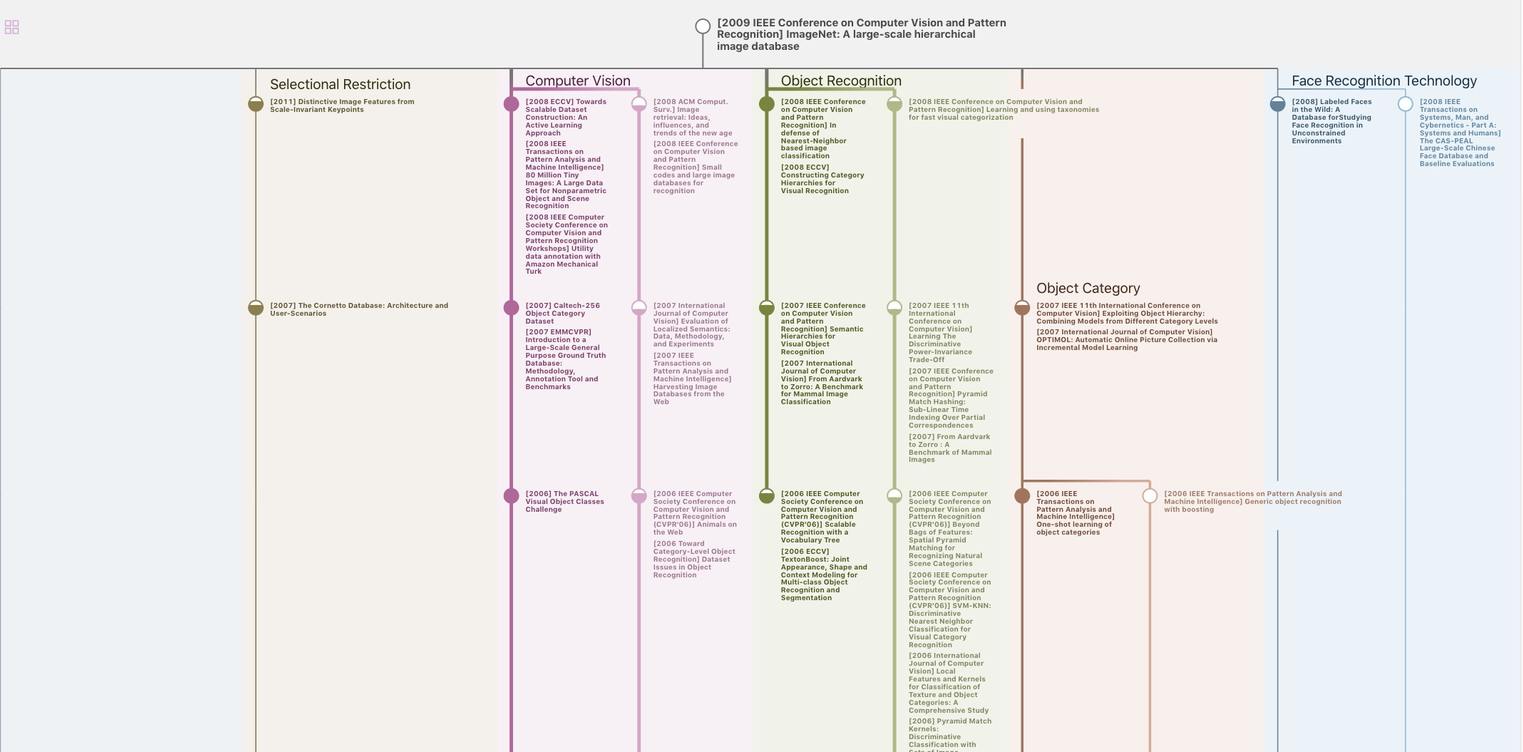
生成溯源树,研究论文发展脉络
Chat Paper
正在生成论文摘要