Performing indoor PM2.5 prediction with low-cost data and machine learning
FACILITIES(2022)
摘要
Purpose The paper aims to develop a particle matter (PM2.5) prediction model for open-plan office space using a variety of data sources. Monitoring of PM2.5 levels is not widely applied in indoor settings. Many reliable methods of monitoring PM2.5 require either time-consuming or expensive equipment, thus making PM2.5 monitoring impractical for many settings. The goal of this paper is to identify possible low-cost, low-effort data sources that building managers can use in combination with machine learning (ML) models to approximate the performance of much more costly monitoring devices. Design/methodology/approach This study identified a variety of data sources, including freely available, public data, data from low-cost sensors and data from expensive, high-quality sensors. This study examined a variety of neural network architectures, including traditional artificial neural networks, generalized recurrent neural networks and long short-term memory neural networks as candidates for the prediction model. The authors trained the selected predictive model using this data and identified data sources that can be cheaply combined to approximate more expensive data sources. Findings The paper identified combinations of free data sources such as building damper percentages and weather data and low-cost sensors such as Wi-Fi-based occupancy estimator or a Plantower PMS7003 sensor that perform nearly as well as predictions made based on nephelometer data. Originality/value This work demonstrates that by combining low-cost sensors and ML, indoor PM2.5 monitoring can be performed at a drastically reduced cost with minimal error compared to more traditional approaches.
更多查看译文
关键词
Indoor air quality, Environmental sensing, Machine learning, Engineering economics, Neural networks, Air quality prediction
AI 理解论文
溯源树
样例
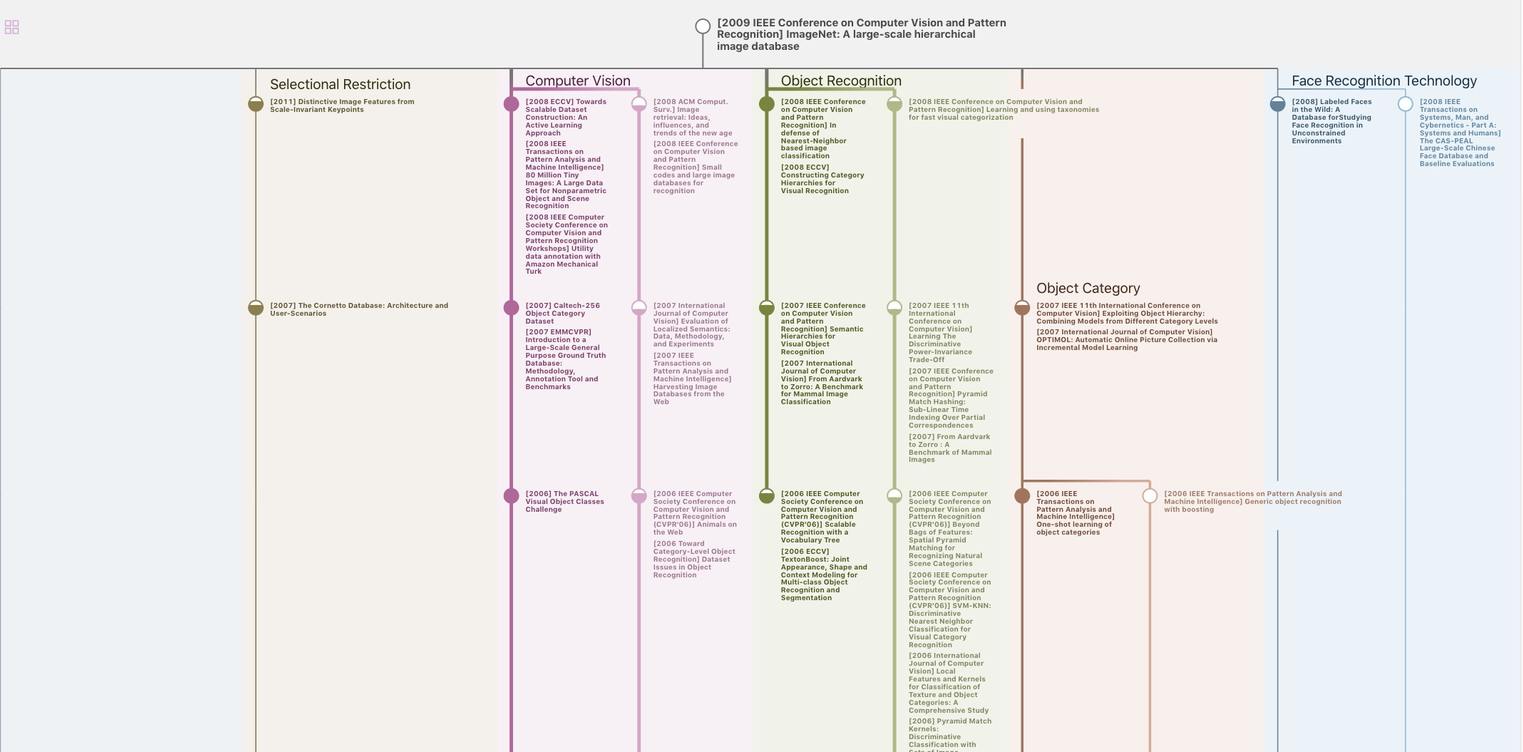
生成溯源树,研究论文发展脉络
Chat Paper
正在生成论文摘要