Efficient Subgroup Discovery Through Auto-Encoding
IDA(2022)
摘要
Current subgroup discovery methods struggle to produce good results for large real-life datasets with high dimensionality. Run times can become high and dependencies between attributes are hard to capture. We propose a method in which auto-encoding is applied for dimensionality reduction before subgroup discovery is performed. In an experimental study, we find that auto-encoding increases both the quality and coverage for our dataset with over 500 attributes. On the dataset with over 250 attributes and the one with the most instances, the coverage improves, while the quality remains similar. For smaller datasets, quality and coverage remain similar or see a minor decrease. Additionally, we greatly improve the run time for each dataset-algorithm combination; for the datasets with over 250 and 500 attributes run times decrease by a factor of on average 150 and 200, respectively. We conclude that dimensionality reduction is a promising method for subgroup discovery in datasets with many attributes and/or a high number of instances.
更多查看译文
关键词
Subgroup discovery, Auto-encoding, Dimensionality reduction
AI 理解论文
溯源树
样例
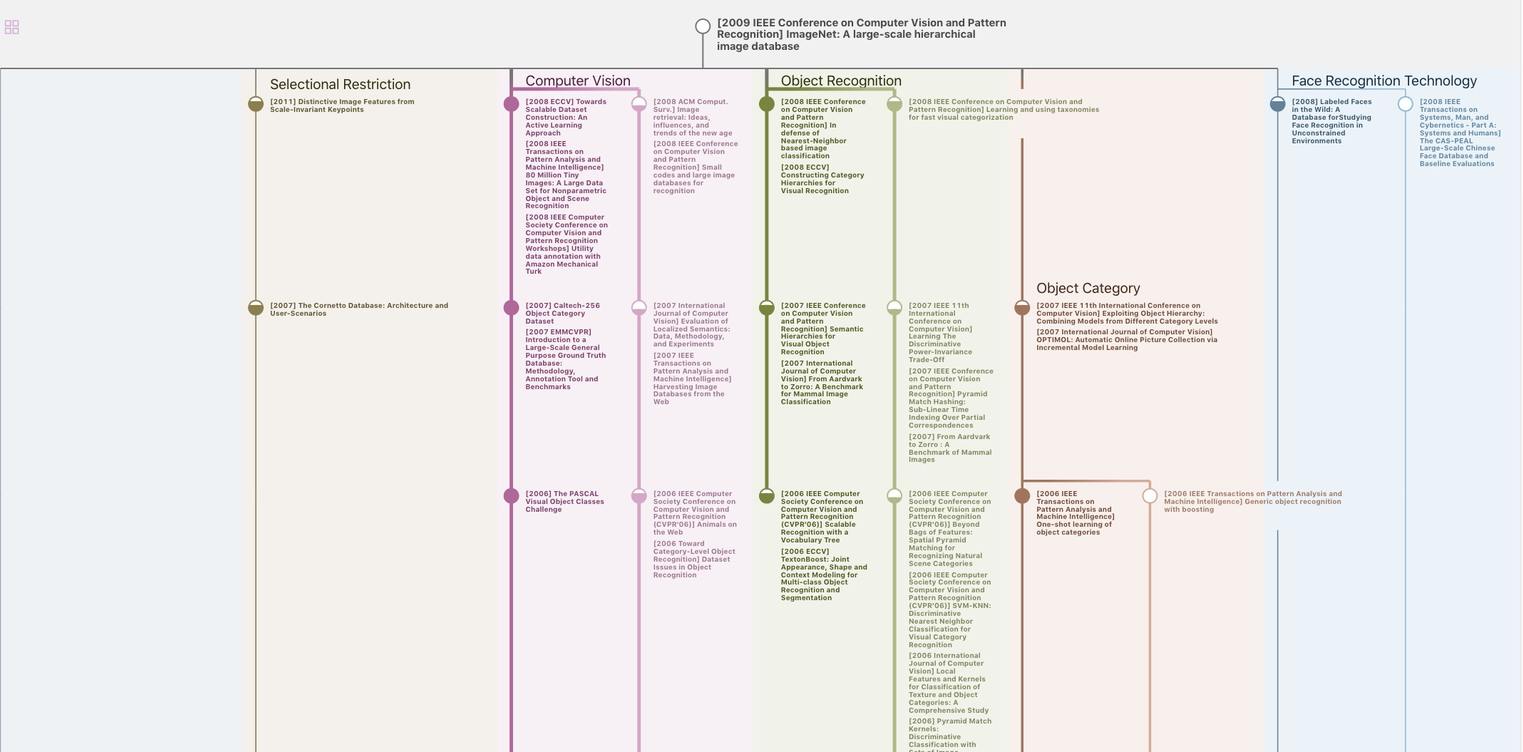
生成溯源树,研究论文发展脉络
Chat Paper
正在生成论文摘要