Feature-weighted AdaBoost classifier for punctuation prediction in Tamil and Hindi NLP systems
EXPERT SYSTEMS(2022)
摘要
Punctuation marks play a vital role in text representation and interpretation, and are useful in enhancing the performance of modern Natural Language Processing (NLP) systems such as voice input typing aids, machine translation, and speech synthesis systems. Punctuation marks, except period, are inherently not available in Indian languages such as Tamil and Hindi. However, some modern forms of writing such as news articles, blogs, stories, and so forth, incorporate user-defined punctuation marks in these languages. The current work proposes an automatic punctuation prediction system for texts in Tamil and Hindi using classification approach, where punctuation prediction is considered as a multi-class classification problem. Word-level text features are chosen and are analysed to validate their language-dependency and significance towards punctuation prediction. A Feature-weighted AdaBoost (FAda) classifier is proposed that defines a novel boosting factor to adjust the hypothesis weight of the weak classifiers, hence reducing the number of false classifications. It is observed that the proposed classifier outperforms the other classification techniques such as, AdaBoost, SVM, CART, CRF, and Bi-LSTM by a maximum difference of 50% and 16% in the macro F1-scores for Tamil and Hindi texts, respectively. The proposed classifier performs on par with the attention-based classifier for both Tamil and Hindi texts. Further, as a proof of concept, the proposed punctuation prediction system is applied to voice keyboard, machine translation, and speech synthesis systems, to validate the effect of the punctuation marks on the performance of these Natural Language Processing (NLP) systems.
更多查看译文
关键词
feature contribution,feature-weighted AdaBoost,NLP system,punctuation prediction
AI 理解论文
溯源树
样例
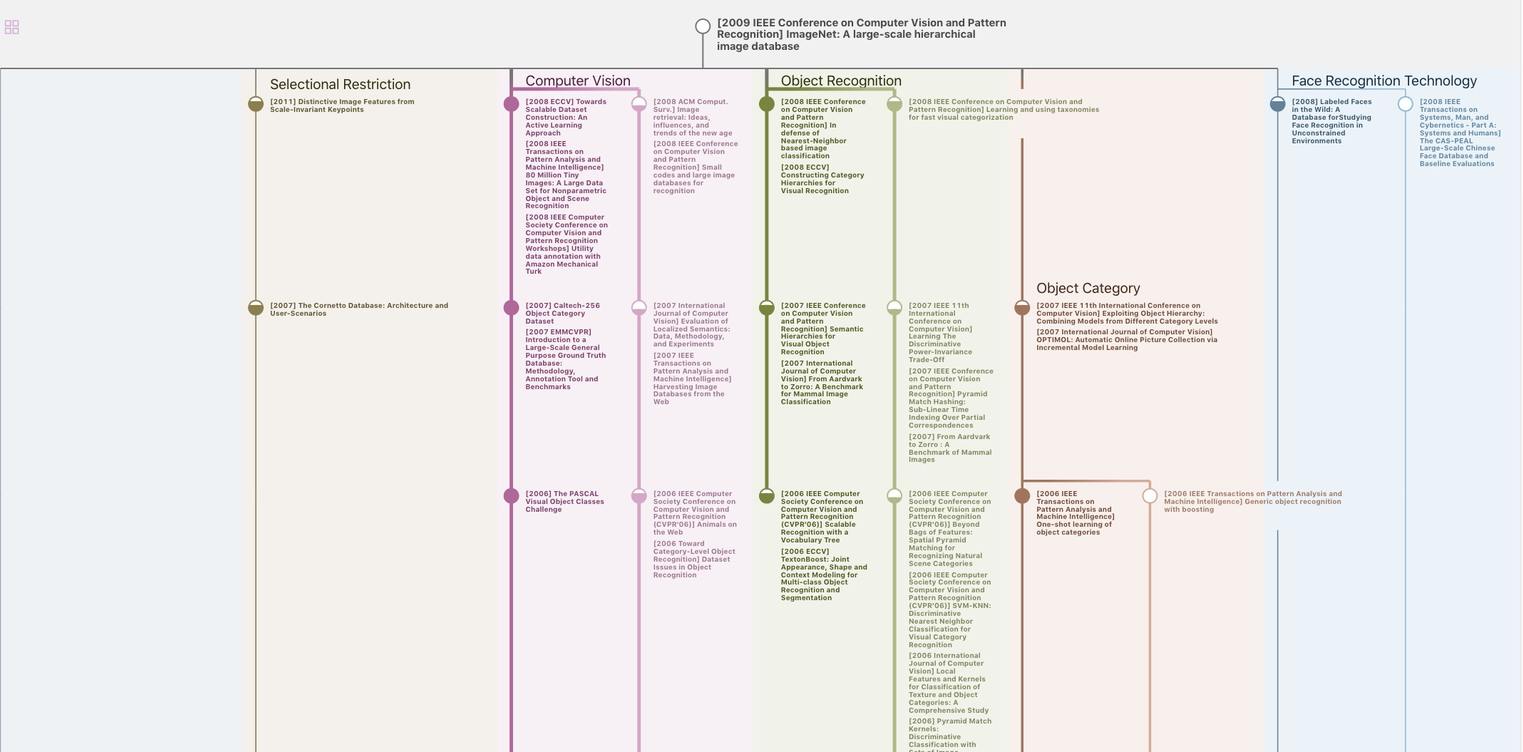
生成溯源树,研究论文发展脉络
Chat Paper
正在生成论文摘要