Acronym Extraction with Hybrid Strategies.
AAAI Conference on Artificial Intelligence(2022)
摘要
Acronym extraction plays an important role in scientific document understanding. Recently, the AAAI-22 Workshop on Scientific Document Understanding released multiple highquality datasets and attracted widespread attention. In this work, we present our hybrid strategies with adversarial training for this task. Specifically, we first apply pre-trained models to obtain contextualized text encoding. Then, on the one hand, we employ a sequence labeling strategy with BiLSTM and CRF to tag each word in a sentence. On the other hand, we use a span selection strategy that directly predicts the acronym and long-form spans. In addition, we adopt adversarial training to further improve the robustness and generalization ability of our models. Experimental results show that both methods outperform strong baselines and rank high on the SDU@AAAI-22 Shared Task 1: Acronym Extraction, our scores rank 2nd in 4 test sets and 3rd in 3 test sets. Moreover, the ablation study further verifies the effectiveness of each component. Our code is available at https://github.com/carlyoung1999/AAAI-SDU-Task1 .
更多查看译文
AI 理解论文
溯源树
样例
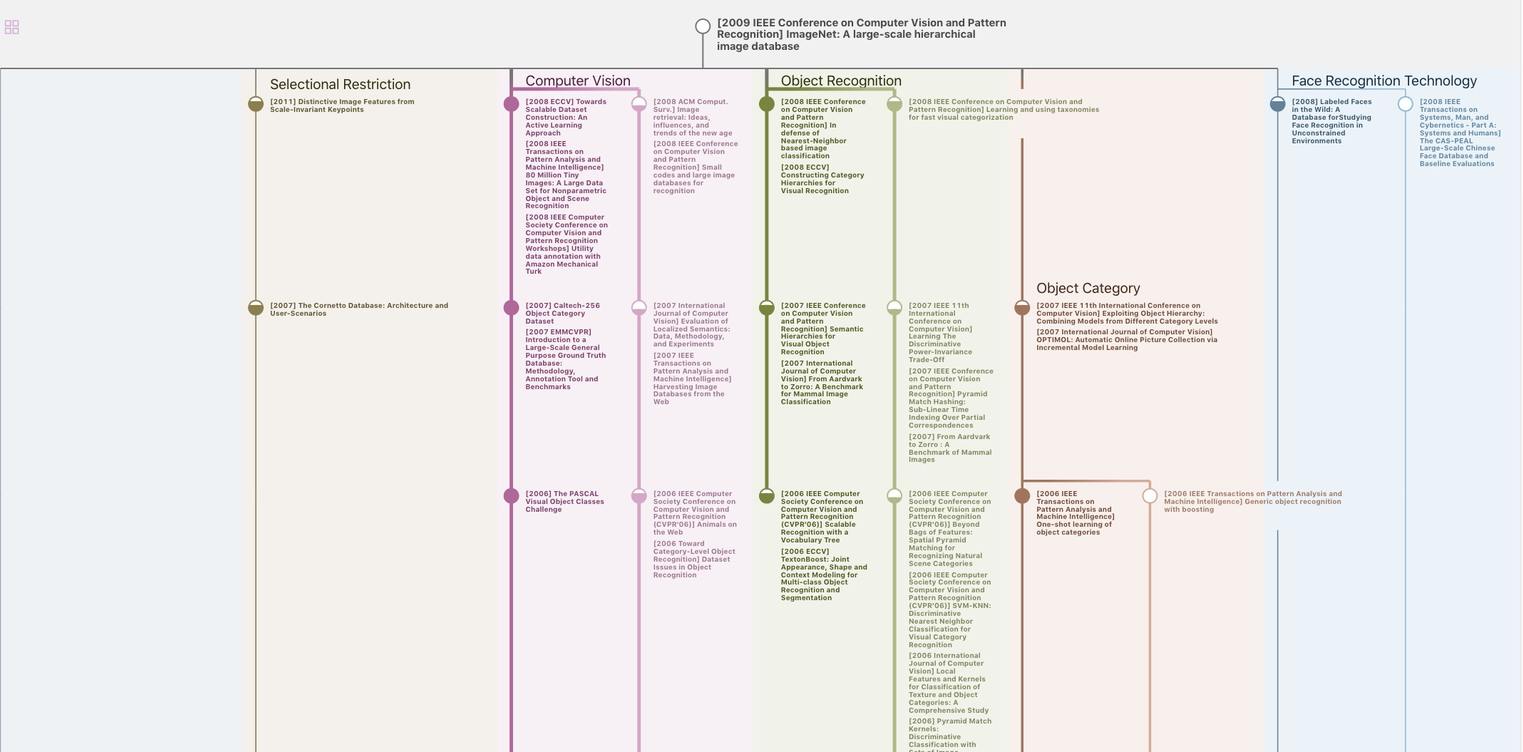
生成溯源树,研究论文发展脉络
Chat Paper
正在生成论文摘要