Backdoor attacks-resilient aggregation based on Robust Filtering of Outliers in federated learning for image classification
Knowledge-Based Systems(2022)
摘要
Federated Learning is a distributed machine learning paradigm vulnerable to different kind of adversarial attacks, since its distributed nature and the inaccessibility of the data by the central server. In this work, we focus on model-poisoning backdoor attacks, because they are characterized by their stealth and effectiveness. We claim that the model updates of the clients of a federated learning setting follow a Gaussian distribution, and those ones with an outlier behavior in that distribution are likely to be adversarial clients. We propose a new federated aggregation operator called Robust Filtering of one-dimensional Outliers (RFOut-1d), which works as a resilient defensive mechanism to model-poisoning backdoor attacks. RFOut-1d is based on an univariate outlier detection method that filters out the model updates of the adversarial clients. The results on three federated image classification dataset show that RFOut-1d dissipates the impact of the backdoor attacks to almost nullifying them throughout all the learning rounds, as well as it keeps the performance of the federated learning model and it outperforms that state-of-the-art defenses against backdoor attacks.
更多查看译文
关键词
Federated Learning,Backdoor attacks,Resilient aggregation,Robust filtering of outliers
AI 理解论文
溯源树
样例
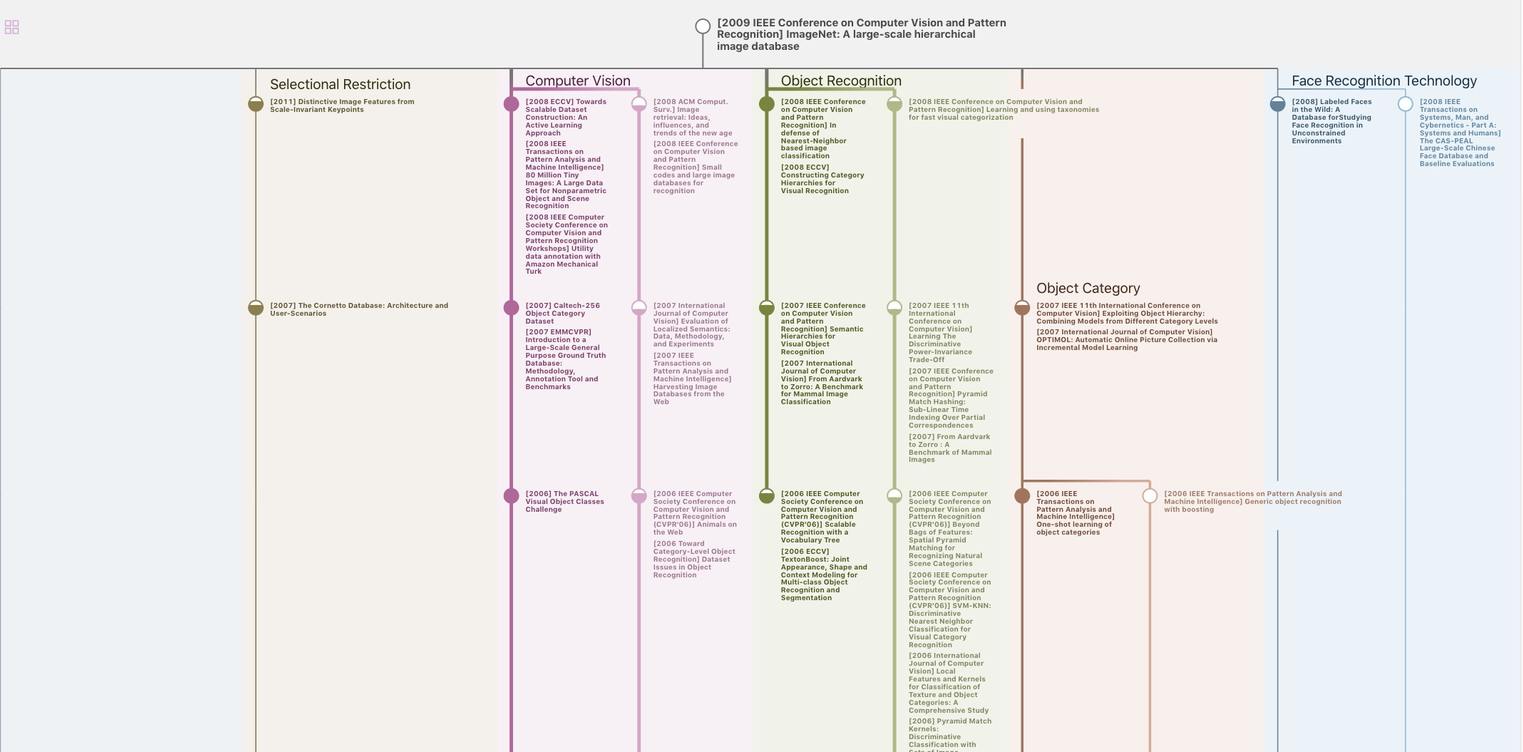
生成溯源树,研究论文发展脉络
Chat Paper
正在生成论文摘要