Density Peak Clustering with connectivity estimation
Knowledge-Based Systems(2022)
摘要
In 2014, a novel clustering algorithm called Density Peak Clustering (DPC) was proposed in journal Science, which has received great attention in many fields due to its simplicity and effectiveness. However, empirical studies have demonstrated that DPC has two main deficiencies: 1. It is very hard to identify the true cluster centers in the decision graph provided by DPC, especially when handling clusters with non-spherical shapes and non-uniform densities; 2. The performance of DPC is significantly affected by the ‘chain reaction’, i.e., an incorrect assignment of the point with the highest density of a region will lead all points in this region to the same wrong cluster. To address these two deficiencies, a density peak clustering with connectivity estimation (DPC”–CE) is presented. In the improved algorithm, points with higher relative distance are chosen as local centers for further calculation. Then a graph-based strategy is proposed to estimate the connectivity information between local centers. With the estimated information, a distance punishment which considers both Euclidean distance and connectivity information is further applied to reassess the similarity between local centers. By adding connectivity information into distance calculation, DPC-CE can not only ensure the true cluster centers can stand out in the decision graph, but also assign all local centers correctly, even on clusters with arbitrary shapes and non-uniform densities. And because of the ‘chain reaction’ we discussed above, those local centers will further lead all points around them to the right cluster. Experimental results on 14 synthetic datasets and 10 read-world datasets demonstrate the effectiveness and robustness of DPC”–CE in terms of three evaluation metrics.
更多查看译文
关键词
Clustering,Density peaks,Local centers,Connectivity estimation,Distance punishment
AI 理解论文
溯源树
样例
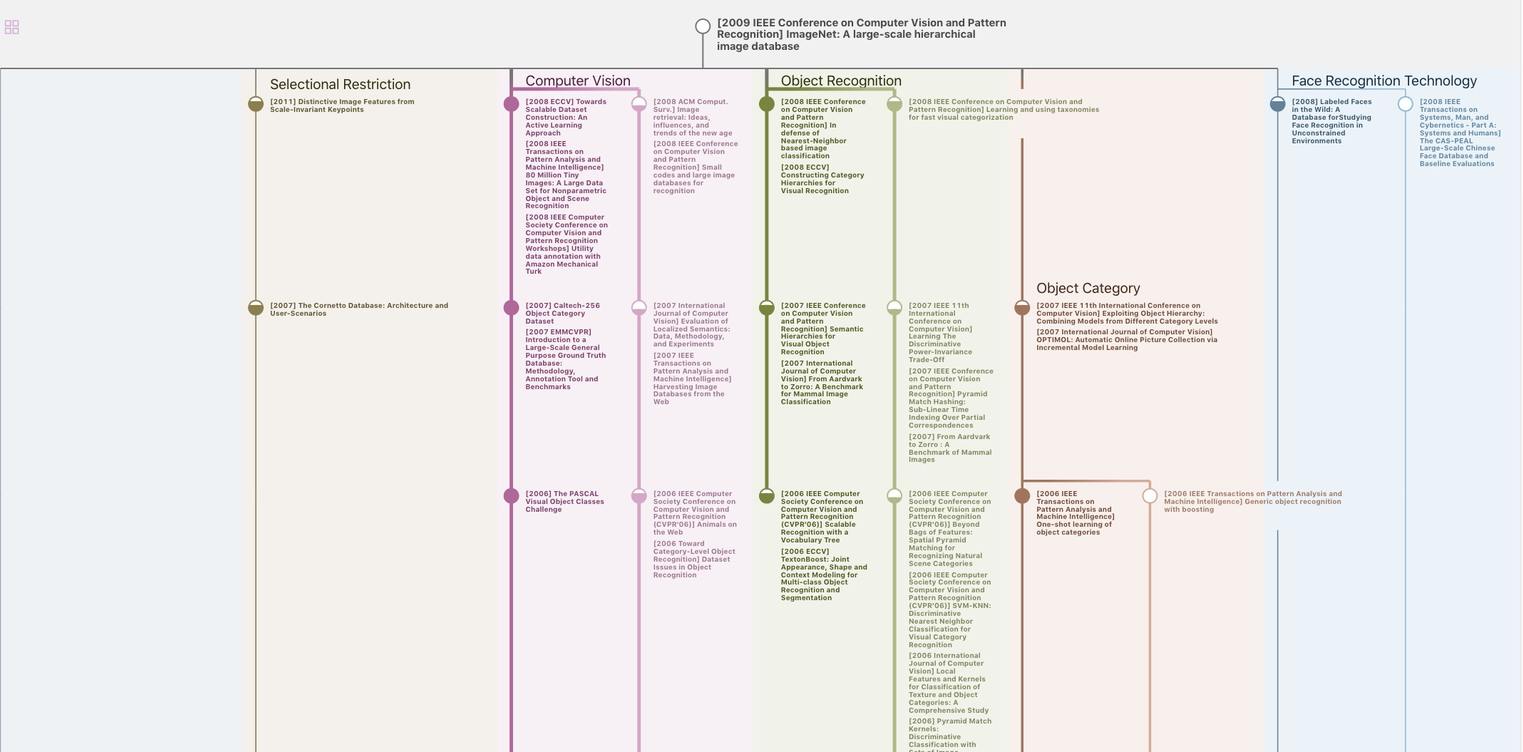
生成溯源树,研究论文发展脉络
Chat Paper
正在生成论文摘要